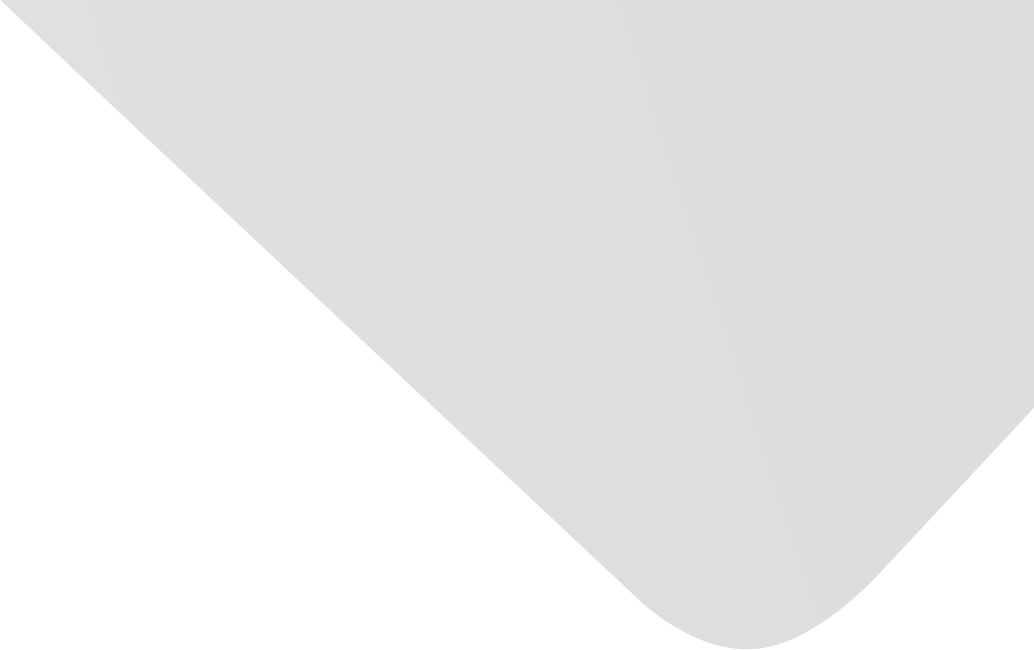
Multilayer Hybrid Deep-Learning Method for Waste Classification and Recycling
Joint Authors
Chu, Yinghao
Huang, Chen
Xie, Xiaodan
Tan, Bohai
Kamal, Shyam
Xiong, Xiaogang
Source
Computational Intelligence and Neuroscience
Issue
Vol. 2018, Issue 2018 (31 Dec. 2018), pp.1-9, 9 p.
Publisher
Hindawi Publishing Corporation
Publication Date
2018-11-01
Country of Publication
Egypt
No. of Pages
9
Main Subjects
Abstract EN
This study proposes a multilayer hybrid deep-learning system (MHS) to automatically sort waste disposed of by individuals in the urban public area.
This system deploys a high-resolution camera to capture waste image and sensors to detect other useful feature information.
The MHS uses a CNN-based algorithm to extract image features and a multilayer perceptrons (MLP) method to consolidate image features and other feature information to classify wastes as recyclable or the others.
The MHS is trained and validated against the manually labelled items, achieving overall classification accuracy higher than 90% under two different testing scenarios, which significantly outperforms a reference CNN-based method relying on image-only inputs.
American Psychological Association (APA)
Chu, Yinghao& Huang, Chen& Xie, Xiaodan& Tan, Bohai& Kamal, Shyam& Xiong, Xiaogang. 2018. Multilayer Hybrid Deep-Learning Method for Waste Classification and Recycling. Computational Intelligence and Neuroscience،Vol. 2018, no. 2018, pp.1-9.
https://search.emarefa.net/detail/BIM-1130761
Modern Language Association (MLA)
Chu, Yinghao…[et al.]. Multilayer Hybrid Deep-Learning Method for Waste Classification and Recycling. Computational Intelligence and Neuroscience No. 2018 (2018), pp.1-9.
https://search.emarefa.net/detail/BIM-1130761
American Medical Association (AMA)
Chu, Yinghao& Huang, Chen& Xie, Xiaodan& Tan, Bohai& Kamal, Shyam& Xiong, Xiaogang. Multilayer Hybrid Deep-Learning Method for Waste Classification and Recycling. Computational Intelligence and Neuroscience. 2018. Vol. 2018, no. 2018, pp.1-9.
https://search.emarefa.net/detail/BIM-1130761
Data Type
Journal Articles
Language
English
Notes
Includes bibliographical references
Record ID
BIM-1130761