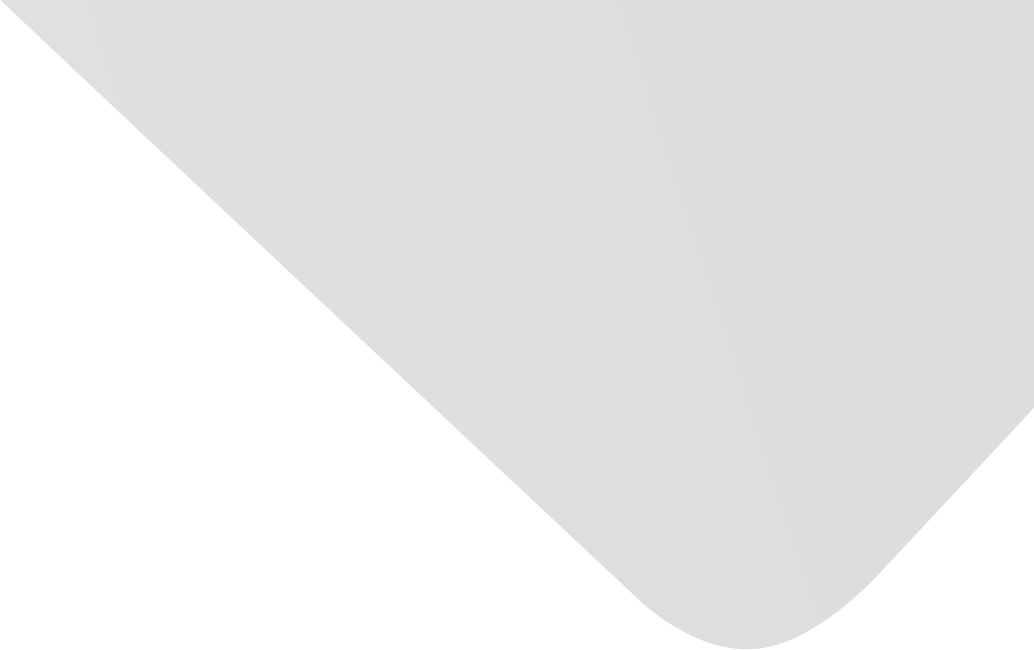
Kernel Entropy Component Analysis with Nongreedy L1-Norm Maximization
Joint Authors
Source
Computational Intelligence and Neuroscience
Issue
Vol. 2018, Issue 2018 (31 Dec. 2018), pp.1-9, 9 p.
Publisher
Hindawi Publishing Corporation
Publication Date
2018-10-14
Country of Publication
Egypt
No. of Pages
9
Main Subjects
Abstract EN
Kernel entropy component analysis (KECA) is a newly proposed dimensionality reduction (DR) method, which has showed superiority in many pattern analysis issues previously solved by principal component analysis (PCA).
The optimized KECA (OKECA) is a state-of-the-art variant of KECA and can return projections retaining more expressive power than KECA.
However, OKECA is sensitive to outliers and accused of its high computational complexities due to its inherent properties of L2-norm.
To handle these two problems, we develop a new extension to KECA, namely, KECA-L1, for DR or feature extraction.
KECA-L1 aims to find a more robust kernel decomposition matrix such that the extracted features retain information potential as much as possible, which is measured by L1-norm.
Accordingly, we design a nongreedy iterative algorithm which has much faster convergence than OKECA’s.
Moreover, a general semisupervised classifier is developed for KECA-based methods and employed into the data classification.
Extensive experiments on data classification and software defect prediction demonstrate that our new method is superior to most existing KECA- and PCA-based approaches.
Code has been also made publicly available.
American Psychological Association (APA)
Ji, Haijin& Huang, Song. 2018. Kernel Entropy Component Analysis with Nongreedy L1-Norm Maximization. Computational Intelligence and Neuroscience،Vol. 2018, no. 2018, pp.1-9.
https://search.emarefa.net/detail/BIM-1130820
Modern Language Association (MLA)
Ji, Haijin& Huang, Song. Kernel Entropy Component Analysis with Nongreedy L1-Norm Maximization. Computational Intelligence and Neuroscience No. 2018 (2018), pp.1-9.
https://search.emarefa.net/detail/BIM-1130820
American Medical Association (AMA)
Ji, Haijin& Huang, Song. Kernel Entropy Component Analysis with Nongreedy L1-Norm Maximization. Computational Intelligence and Neuroscience. 2018. Vol. 2018, no. 2018, pp.1-9.
https://search.emarefa.net/detail/BIM-1130820
Data Type
Journal Articles
Language
English
Notes
Includes bibliographical references
Record ID
BIM-1130820