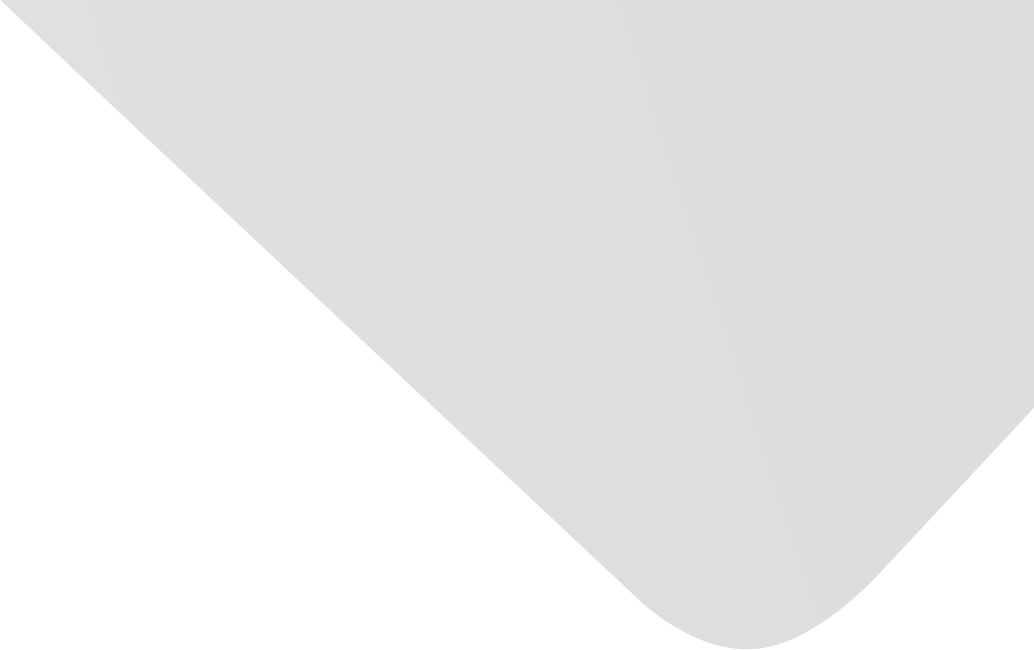
A Neural Machine Translation Model for Arabic Dialects That Utilizes Multitask Learning (MTL)
Joint Authors
Park, Seong-Bae
Park, Se-Young
Baniata, Laith H.
Source
Computational Intelligence and Neuroscience
Issue
Vol. 2018, Issue 2018 (31 Dec. 2018), pp.1-10, 10 p.
Publisher
Hindawi Publishing Corporation
Publication Date
2018-12-10
Country of Publication
Egypt
No. of Pages
10
Main Subjects
Abstract EN
In this research article, we study the problem of employing a neural machine translation model to translate Arabic dialects to Modern Standard Arabic.
The proposed solution of the neural machine translation model is prompted by the recurrent neural network-based encoder-decoder neural machine translation model that has been proposed recently, which generalizes machine translation as sequence learning problems.
We propose the development of a multitask learning (MTL) model which shares one decoder among language pairs, and every source language has a separate encoder.
The proposed model can be applied to limited volumes of data as well as extensive amounts of data.
Experiments carried out have shown that the proposed MTL model can ensure a higher quality of translation when compared to the individually learned model.
American Psychological Association (APA)
Baniata, Laith H.& Park, Se-Young& Park, Seong-Bae. 2018. A Neural Machine Translation Model for Arabic Dialects That Utilizes Multitask Learning (MTL). Computational Intelligence and Neuroscience،Vol. 2018, no. 2018, pp.1-10.
https://search.emarefa.net/detail/BIM-1130835
Modern Language Association (MLA)
Baniata, Laith H.…[et al.]. A Neural Machine Translation Model for Arabic Dialects That Utilizes Multitask Learning (MTL). Computational Intelligence and Neuroscience No. 2018 (2018), pp.1-10.
https://search.emarefa.net/detail/BIM-1130835
American Medical Association (AMA)
Baniata, Laith H.& Park, Se-Young& Park, Seong-Bae. A Neural Machine Translation Model for Arabic Dialects That Utilizes Multitask Learning (MTL). Computational Intelligence and Neuroscience. 2018. Vol. 2018, no. 2018, pp.1-10.
https://search.emarefa.net/detail/BIM-1130835
Data Type
Journal Articles
Language
English
Notes
Includes bibliographical references
Record ID
BIM-1130835