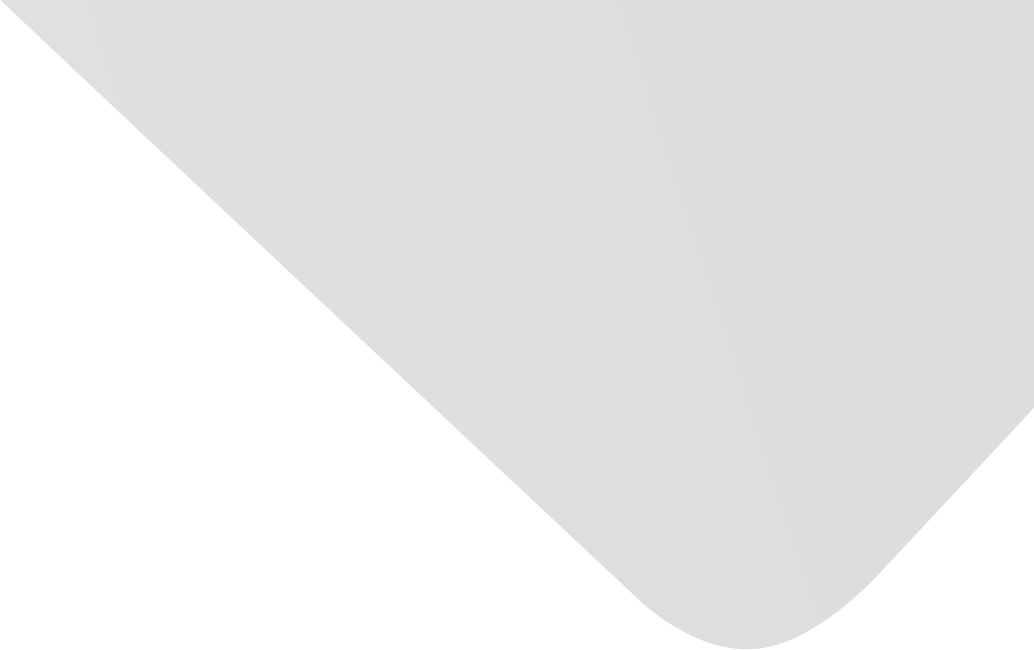
Blind Parameter Identification of MAR Model and Mutation Hybrid GWO-SCA Optimized SVM for Fault Diagnosis of Rotating Machinery
Joint Authors
Fu, Wenlong
Tan, Jiawen
Zhang, Xiaoyuan
Chen, Tie
Wang, Kai
Source
Issue
Vol. 2019, Issue 2019 (31 Dec. 2019), pp.1-17, 17 p.
Publisher
Hindawi Publishing Corporation
Publication Date
2019-04-17
Country of Publication
Egypt
No. of Pages
17
Main Subjects
Abstract EN
As a crucial and widely used component in industrial fields with great complexity, the health condition of rotating machinery is directly related to production efficiency and safety.
Consequently, recognizing and diagnosing rotating machine faults remain to be one of the main concerns in preventing failures of mechanical systems, which can enhance the reliability and efficiency of mechanical systems.
In this paper, a novel approach based on blind parameter identification of MAR model and mutation hybrid GWO-SCA optimization is proposed to diagnose faults for rotating machinery.
Signals collected from different types of faults were firstly split into sets of intrinsic mode functions (IMFs) by variational mode decomposition (VMD), the decomposing mode number K of which was preset with central frequency observation method.
Then the multivariate autoregressive (MAR) model of all IMFs was established, whose order was determined by Schwartz Bayes Criterion (SBC), and all parameters of the model were identified blindly through QR decomposition, where key features were subsequently extracted via principal component analysis (PCA) to construct feature vectors of different fault types.
Afterwards, a hybrid optimization algorithm combining mutation operator, grey wolf optimizer (GWO), and sine cosine algorithm (SCA), termed mutation hybrid GWO-SCA (MHGWOSCA), was proposed for parameter selection of support vector machine (SVM).
The optimal SVM model was later employed to classify different fault samples.
The engineering application and contrastive analysis indicate the availability and superiority of the proposed method.
American Psychological Association (APA)
Fu, Wenlong& Tan, Jiawen& Zhang, Xiaoyuan& Chen, Tie& Wang, Kai. 2019. Blind Parameter Identification of MAR Model and Mutation Hybrid GWO-SCA Optimized SVM for Fault Diagnosis of Rotating Machinery. Complexity،Vol. 2019, no. 2019, pp.1-17.
https://search.emarefa.net/detail/BIM-1131372
Modern Language Association (MLA)
Fu, Wenlong…[et al.]. Blind Parameter Identification of MAR Model and Mutation Hybrid GWO-SCA Optimized SVM for Fault Diagnosis of Rotating Machinery. Complexity No. 2019 (2019), pp.1-17.
https://search.emarefa.net/detail/BIM-1131372
American Medical Association (AMA)
Fu, Wenlong& Tan, Jiawen& Zhang, Xiaoyuan& Chen, Tie& Wang, Kai. Blind Parameter Identification of MAR Model and Mutation Hybrid GWO-SCA Optimized SVM for Fault Diagnosis of Rotating Machinery. Complexity. 2019. Vol. 2019, no. 2019, pp.1-17.
https://search.emarefa.net/detail/BIM-1131372
Data Type
Journal Articles
Language
English
Notes
Includes bibliographical references
Record ID
BIM-1131372