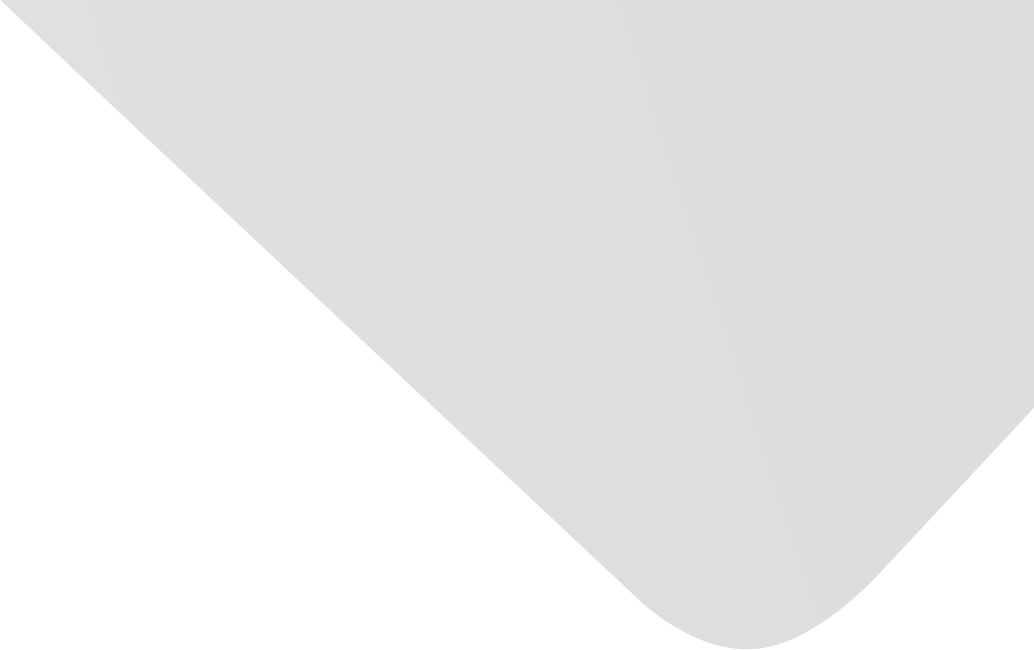
Fully Automated Delineation of Gross Tumor Volume for Head and Neck Cancer on PET-CT Using Deep Learning: A Dual-Center Study
Joint Authors
Huang, Bingsheng
Li, Qiaoliang
Chen, Zhewei
Feng, Shi-Ting
Ye, Yufeng
Huang, Bin
Wu, Po-Man
Wong, Ching-Yee Oliver
Zheng, Liyun
Liu, Yong
Wang, Tian-Fu
Source
Contrast Media & Molecular Imaging
Issue
Vol. 2018, Issue 2018 (31 Dec. 2018), pp.1-12, 12 p.
Publisher
Hindawi Publishing Corporation
Publication Date
2018-10-24
Country of Publication
Egypt
No. of Pages
12
Main Subjects
Abstract EN
Purpose.
In this study, we proposed an automated deep learning (DL) method for head and neck cancer (HNC) gross tumor volume (GTV) contouring on positron emission tomography-computed tomography (PET-CT) images.
Materials and Methods.
PET-CT images were collected from 22 newly diagnosed HNC patients, of whom 17 (Database 1) and 5 (Database 2) were from two centers, respectively.
An oncologist and a radiologist decided the gold standard of GTV manually by consensus.
We developed a deep convolutional neural network (DCNN) and trained the network based on the two-dimensional PET-CT images and the gold standard of GTV in the training dataset.
We did two experiments: Experiment 1, with Database 1 only, and Experiment 2, with both Databases 1 and 2.
In both Experiment 1 and Experiment 2, we evaluated the proposed method using a leave-one-out cross-validation strategy.
We compared the median results in Experiment 2 (GTVa) with the performance of other methods in the literature and with the gold standard (GTVm).
Results.
A tumor segmentation task for a patient on coregistered PET-CT images took less than one minute.
The dice similarity coefficient (DSC) of the proposed method in Experiment 1 and Experiment 2 was 0.481∼0.872 and 0.482∼0.868, respectively.
The DSC of GTVa was better than that in previous studies.
A high correlation was found between GTVa and GTVm (R = 0.99, P<0.001).
The median volume difference (%) between GTVm and GTVa was 10.9%.
The median values of DSC, sensitivity, and precision of GTVa were 0.785, 0.764, and 0.789, respectively.
Conclusion.
A fully automatic GTV contouring method for HNC based on DCNN and PET-CT from dual centers has been successfully proposed with high accuracy and efficiency.
Our proposed method is of help to the clinicians in HNC management.
American Psychological Association (APA)
Huang, Bin& Chen, Zhewei& Wu, Po-Man& Ye, Yufeng& Feng, Shi-Ting& Wong, Ching-Yee Oliver…[et al.]. 2018. Fully Automated Delineation of Gross Tumor Volume for Head and Neck Cancer on PET-CT Using Deep Learning: A Dual-Center Study. Contrast Media & Molecular Imaging،Vol. 2018, no. 2018, pp.1-12.
https://search.emarefa.net/detail/BIM-1131609
Modern Language Association (MLA)
Huang, Bin…[et al.]. Fully Automated Delineation of Gross Tumor Volume for Head and Neck Cancer on PET-CT Using Deep Learning: A Dual-Center Study. Contrast Media & Molecular Imaging No. 2018 (2018), pp.1-12.
https://search.emarefa.net/detail/BIM-1131609
American Medical Association (AMA)
Huang, Bin& Chen, Zhewei& Wu, Po-Man& Ye, Yufeng& Feng, Shi-Ting& Wong, Ching-Yee Oliver…[et al.]. Fully Automated Delineation of Gross Tumor Volume for Head and Neck Cancer on PET-CT Using Deep Learning: A Dual-Center Study. Contrast Media & Molecular Imaging. 2018. Vol. 2018, no. 2018, pp.1-12.
https://search.emarefa.net/detail/BIM-1131609
Data Type
Journal Articles
Language
English
Notes
Includes bibliographical references
Record ID
BIM-1131609