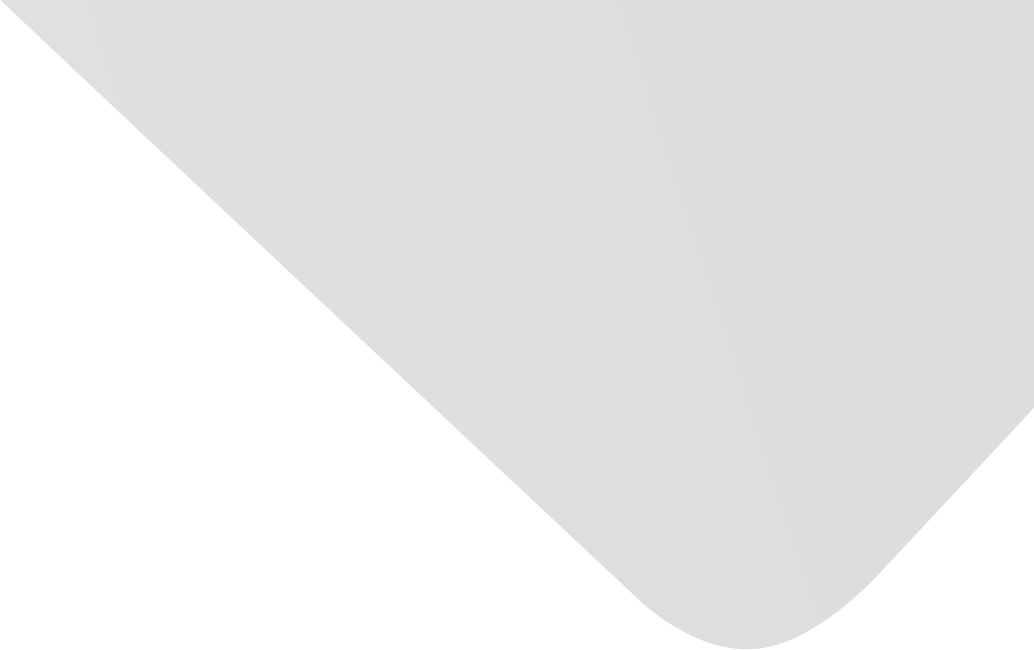
Is Deep Learning for Image Recognition Applicable to Stock Market Prediction?
Joint Authors
Sim, Hyun Sik
Kim, Hae In
Ahn, Jae Joon
Source
Issue
Vol. 2019, Issue 2019 (31 Dec. 2019), pp.1-10, 10 p.
Publisher
Hindawi Publishing Corporation
Publication Date
2019-02-19
Country of Publication
Egypt
No. of Pages
10
Main Subjects
Abstract EN
Stock market prediction is a challenging issue for investors.
In this paper, we propose a stock price prediction model based on convolutional neural network (CNN) to validate the applicability of new learning methods in stock markets.
When applying CNN, 9 technical indicators were chosen as predictors of the forecasting model, and the technical indicators were converted to images of the time series graph.
For verifying the usefulness of deep learning for image recognition in stock markets, the predictive accuracies of the proposed model were compared to typical artificial neural network (ANN) model and support vector machine (SVM) model.
From the experimental results, we can see that CNN can be a desirable choice for building stock prediction models.
To examine the performance of the proposed method, an empirical study was performed using the S&P 500 index.
This study addresses two critical issues regarding the use of CNN for stock price prediction: how to use CNN and how to optimize them.
American Psychological Association (APA)
Sim, Hyun Sik& Kim, Hae In& Ahn, Jae Joon. 2019. Is Deep Learning for Image Recognition Applicable to Stock Market Prediction?. Complexity،Vol. 2019, no. 2019, pp.1-10.
https://search.emarefa.net/detail/BIM-1131785
Modern Language Association (MLA)
Sim, Hyun Sik…[et al.]. Is Deep Learning for Image Recognition Applicable to Stock Market Prediction?. Complexity No. 2019 (2019), pp.1-10.
https://search.emarefa.net/detail/BIM-1131785
American Medical Association (AMA)
Sim, Hyun Sik& Kim, Hae In& Ahn, Jae Joon. Is Deep Learning for Image Recognition Applicable to Stock Market Prediction?. Complexity. 2019. Vol. 2019, no. 2019, pp.1-10.
https://search.emarefa.net/detail/BIM-1131785
Data Type
Journal Articles
Language
English
Notes
Includes bibliographical references
Record ID
BIM-1131785