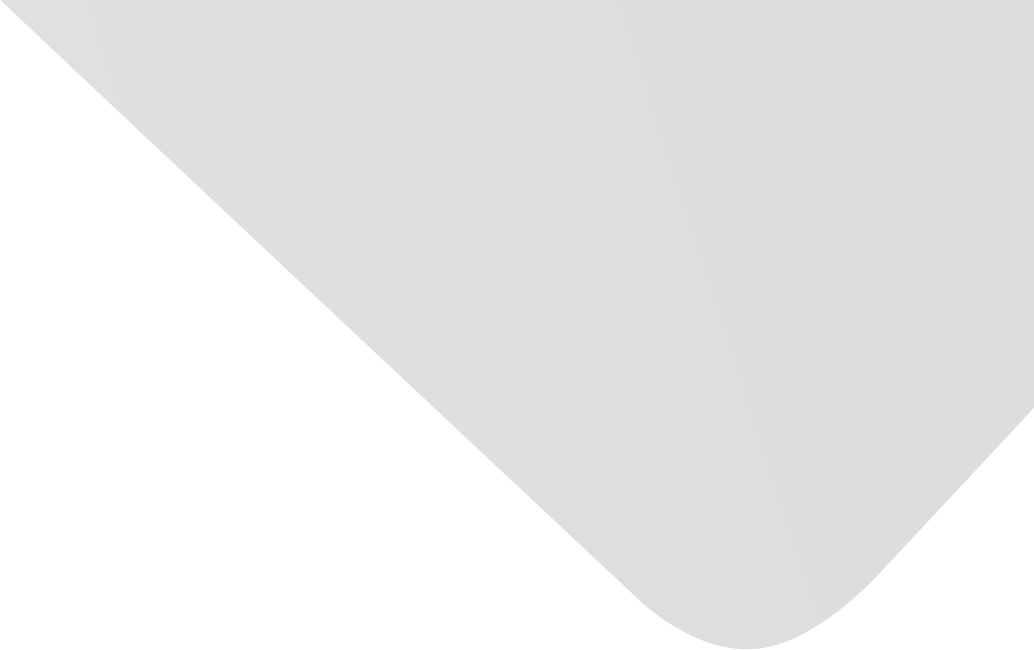
Effect of Missing Data Imputation on Deep Learning Prediction Performance for Vesicoureteral Reflux and Recurrent Urinary Tract Infection Clinical Study
Joint Authors
Köse, Timur
Özgür, Su
Coşgun, Erdal
Keskinoğlu, Ahmet
Keskinoğlu, Pembe
Source
Issue
Vol. 2020, Issue 2020 (31 Dec. 2020), pp.1-15, 15 p.
Publisher
Hindawi Publishing Corporation
Publication Date
2020-07-15
Country of Publication
Egypt
No. of Pages
15
Main Subjects
Abstract EN
Missing observations are always a challenging problem that we have to deal with in diseases that require follow-up.
In hospital records for vesicoureteral reflux (VUR) and recurrent urinary tract infection (rUTI), the number of complete cases is very low on demographic and clinical characteristics, laboratory findings, and imaging data.
On the other hand, deep learning (DL) approaches can be used for highly missing observation scenarios with its own missing ratio algorithm.
In this study, the effects of multiple imputation techniques MICE and FAMD on the performance of DL in the differential diagnosis were compared.
The data of a retrospective cross-sectional study including 611 pediatric patients were evaluated (425 with VUR, 186 with rUTI, 26.65% missing ratio) in this research.
CNTK and R 3.6.3 have been used for evaluating different models for 34 features (physical, laboratory, and imaging findings).
In the differential diagnosis of VUR and rUTI, the best performance was obtained by deep learning with MICE algorithm with its values, respectively, 64.05% accuracy, 64.59% sensitivity, and 62.62% specificity.
FAMD algorithm performed with accuracy=61.52, sensitivity=60.20, and specificity was found out to be 61.00 with 3 principal components on missing imputation phase.
DL-based approaches can evaluate datasets without doing preomit/impute missing values from datasets.
Once DL method is used together with appropriate missing imputation techniques, it shows higher predictive performance.
American Psychological Association (APA)
Köse, Timur& Özgür, Su& Coşgun, Erdal& Keskinoğlu, Ahmet& Keskinoğlu, Pembe. 2020. Effect of Missing Data Imputation on Deep Learning Prediction Performance for Vesicoureteral Reflux and Recurrent Urinary Tract Infection Clinical Study. BioMed Research International،Vol. 2020, no. 2020, pp.1-15.
https://search.emarefa.net/detail/BIM-1132123
Modern Language Association (MLA)
Köse, Timur…[et al.]. Effect of Missing Data Imputation on Deep Learning Prediction Performance for Vesicoureteral Reflux and Recurrent Urinary Tract Infection Clinical Study. BioMed Research International No. 2020 (2020), pp.1-15.
https://search.emarefa.net/detail/BIM-1132123
American Medical Association (AMA)
Köse, Timur& Özgür, Su& Coşgun, Erdal& Keskinoğlu, Ahmet& Keskinoğlu, Pembe. Effect of Missing Data Imputation on Deep Learning Prediction Performance for Vesicoureteral Reflux and Recurrent Urinary Tract Infection Clinical Study. BioMed Research International. 2020. Vol. 2020, no. 2020, pp.1-15.
https://search.emarefa.net/detail/BIM-1132123
Data Type
Journal Articles
Language
English
Notes
Includes bibliographical references
Record ID
BIM-1132123