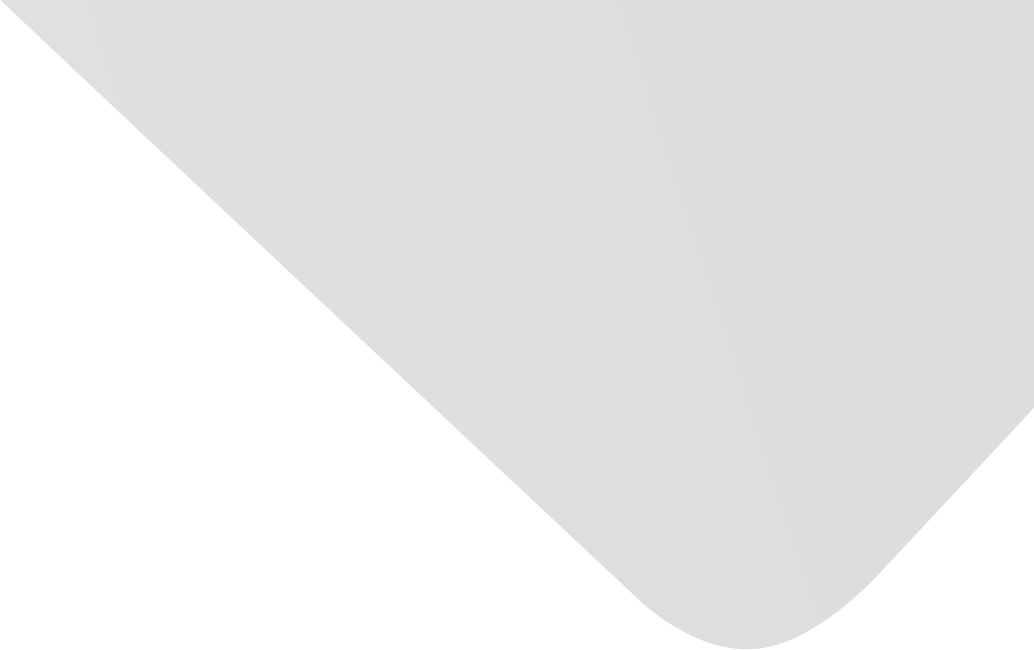
Establishment and Analysis of a Combined Diagnostic Model of Polycystic Ovary Syndrome with Random Forest and Artificial Neural Network
Joint Authors
Xie, Ning-Ning
Wang, Fang-Fang
Zhou, Jue
Liu, Chang
Qu, Fan
Source
Issue
Vol. 2020, Issue 2020 (31 Dec. 2020), pp.1-13, 13 p.
Publisher
Hindawi Publishing Corporation
Publication Date
2020-08-20
Country of Publication
Egypt
No. of Pages
13
Main Subjects
Abstract EN
Polycystic ovary syndrome (PCOS) is one of the most common metabolic and reproductive endocrinopathies.
However, few studies have tried to develop a diagnostic model based on gene biomarkers.
In this study, we applied a computational method by combining two machine learning algorithms, including random forest (RF) and artificial neural network (ANN), to identify gene biomarkers and construct diagnostic model.
We collected gene expression data from Gene Expression Omnibus (GEO) database containing 76 PCOS samples and 57 normal samples; five datasets were utilized, including one dataset for screening differentially expressed genes (DEGs), two training datasets, and two validation datasets.
Firstly, based on RF, 12 key genes in 264 DEGs were identified to be vital for classification of PCOS and normal samples.
Moreover, the weights of these key genes were calculated using ANN with microarray and RNA-seq training dataset, respectively.
Furthermore, the diagnostic models for two types of datasets were developed and named neuralPCOS.
Finally, two validation datasets were used to test and compare the performance of neuralPCOS with other two set of marker genes by area under curve (AUC).
Our model achieved an AUC of 0.7273 in microarray dataset, and 0.6488 in RNA-seq dataset.
To conclude, we uncovered gene biomarkers and developed a novel diagnostic model of PCOS, which would be helpful for diagnosis.
American Psychological Association (APA)
Xie, Ning-Ning& Wang, Fang-Fang& Zhou, Jue& Liu, Chang& Qu, Fan. 2020. Establishment and Analysis of a Combined Diagnostic Model of Polycystic Ovary Syndrome with Random Forest and Artificial Neural Network. BioMed Research International،Vol. 2020, no. 2020, pp.1-13.
https://search.emarefa.net/detail/BIM-1132627
Modern Language Association (MLA)
Xie, Ning-Ning…[et al.]. Establishment and Analysis of a Combined Diagnostic Model of Polycystic Ovary Syndrome with Random Forest and Artificial Neural Network. BioMed Research International No. 2020 (2020), pp.1-13.
https://search.emarefa.net/detail/BIM-1132627
American Medical Association (AMA)
Xie, Ning-Ning& Wang, Fang-Fang& Zhou, Jue& Liu, Chang& Qu, Fan. Establishment and Analysis of a Combined Diagnostic Model of Polycystic Ovary Syndrome with Random Forest and Artificial Neural Network. BioMed Research International. 2020. Vol. 2020, no. 2020, pp.1-13.
https://search.emarefa.net/detail/BIM-1132627
Data Type
Journal Articles
Language
English
Notes
Includes bibliographical references
Record ID
BIM-1132627