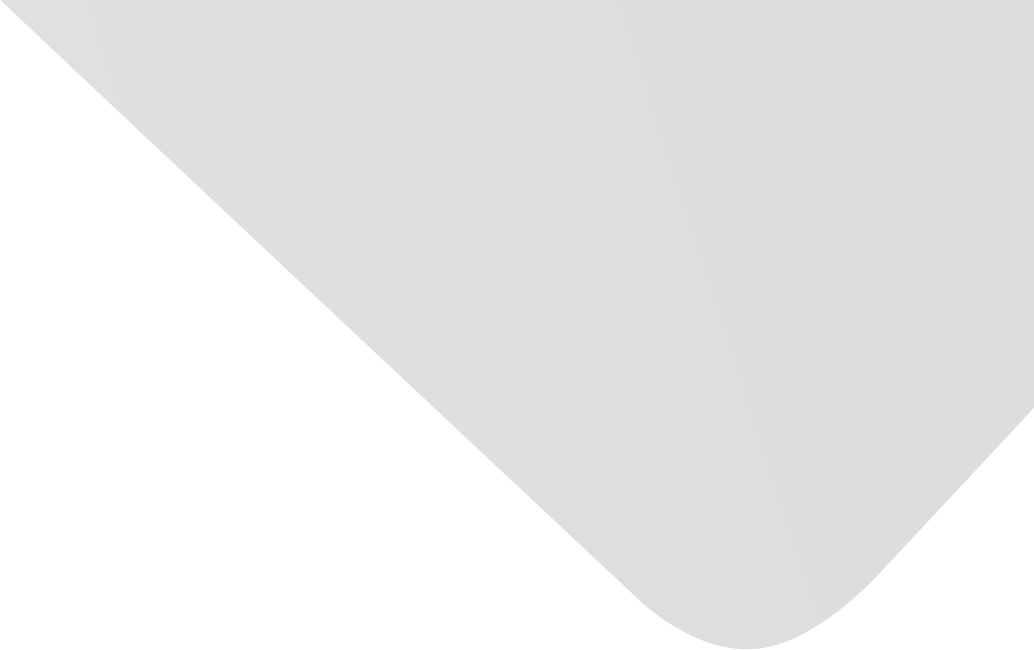
A Differential Evolution-Oriented Pruning Neural Network Model for Bankruptcy Prediction
Joint Authors
Gao, Shangce
Todo, Yuki
Ji, Junkai
Tang, Yajiao
Zhu, Yulin
Tang, Zheng
Source
Issue
Vol. 2019, Issue 2019 (31 Dec. 2019), pp.1-21, 21 p.
Publisher
Hindawi Publishing Corporation
Publication Date
2019-08-04
Country of Publication
Egypt
No. of Pages
21
Main Subjects
Abstract EN
Financial bankruptcy prediction is crucial for financial institutions in assessing the financial health of companies and individuals.
Such work is necessary for financial institutions to establish effective prediction models to make appropriate lending decisions.
In recent decades, various bankruptcy prediction models have been developed for academics and practitioners to predict the likelihood that a loan customer will go bankrupt.
Among them, Artificial Neural Networks (ANNs) have been widely and effectively applied in bankruptcy prediction.
Inspired by the mechanism of biological neurons, we propose an evolutionary pruning neural network (EPNN) model to conduct financial bankruptcy analysis.
The EPNN possesses a dynamic dendritic structure that is trained by a global optimization learning algorithm: the Adaptive Differential Evolution algorithm with Optional External Archive (JADE).
The EPNN can reduce the computational complexity by removing the superfluous and ineffective synapses and dendrites in the structure and is simultaneously able to achieve a competitive classification accuracy.
After simplifying the structure, the EPNN can be entirely replaced by a logic circuit containing the comparators and the logic NOT, AND, and OR gates.
This mechanism makes it feasible to apply the EPNN to bankruptcy analysis in hardware implementations.
To verify the effectiveness of the EPNN, we adopt two benchmark datasets in our experiments.
The experimental results reveal that the EPNN outperforms the Multilayer Perceptron (MLP) model and our previously developed preliminary pruning neural network (PNN) model in terms of accuracy, convergence speed, and Area Under the Receiver Operating Characteristics (ROC) curve (AUC).
In addition, the EPNN also provides competitive and satisfactory classification performances in contrast with other commonly used classification methods.
American Psychological Association (APA)
Tang, Yajiao& Ji, Junkai& Zhu, Yulin& Gao, Shangce& Tang, Zheng& Todo, Yuki. 2019. A Differential Evolution-Oriented Pruning Neural Network Model for Bankruptcy Prediction. Complexity،Vol. 2019, no. 2019, pp.1-21.
https://search.emarefa.net/detail/BIM-1133031
Modern Language Association (MLA)
Tang, Yajiao…[et al.]. A Differential Evolution-Oriented Pruning Neural Network Model for Bankruptcy Prediction. Complexity No. 2019 (2019), pp.1-21.
https://search.emarefa.net/detail/BIM-1133031
American Medical Association (AMA)
Tang, Yajiao& Ji, Junkai& Zhu, Yulin& Gao, Shangce& Tang, Zheng& Todo, Yuki. A Differential Evolution-Oriented Pruning Neural Network Model for Bankruptcy Prediction. Complexity. 2019. Vol. 2019, no. 2019, pp.1-21.
https://search.emarefa.net/detail/BIM-1133031
Data Type
Journal Articles
Language
English
Notes
Includes bibliographical references
Record ID
BIM-1133031