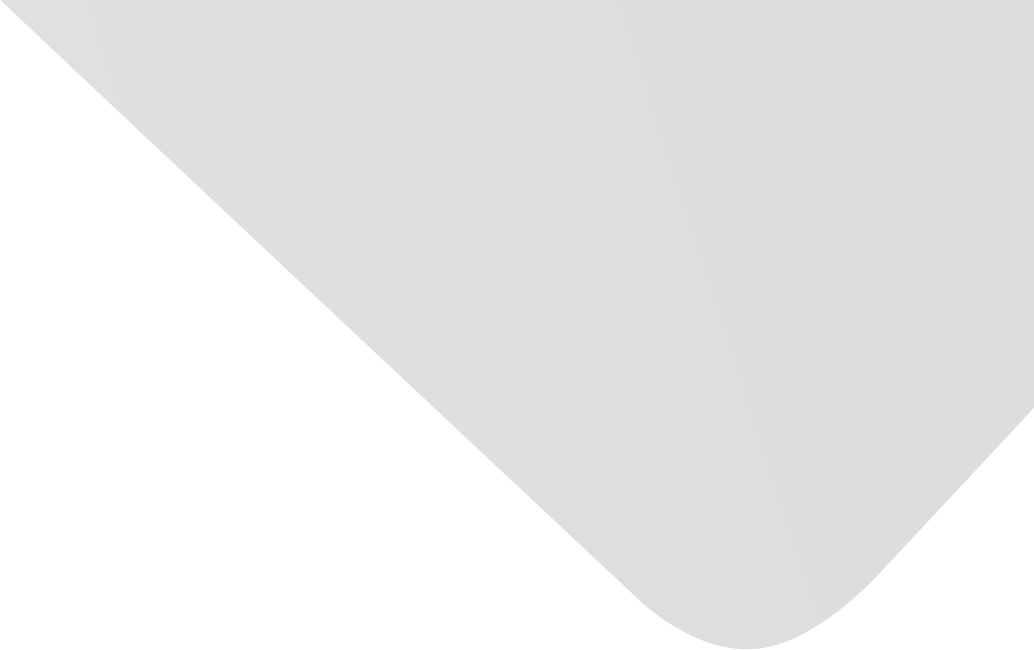
An Improved Demand Forecasting Model Using Deep Learning Approach and Proposed Decision Integration Strategy for Supply Chain
Joint Authors
Kilimci, Zeynep Hilal
Uysal, Mitat
Akyokus, Selim
Akyuz, A. Okay
Uysal, M. Ozan
Atak Bulbul, Berna
Ekmis, Mehmet Ali
Source
Issue
Vol. 2019, Issue 2019 (31 Dec. 2019), pp.1-15, 15 p.
Publisher
Hindawi Publishing Corporation
Publication Date
2019-03-26
Country of Publication
Egypt
No. of Pages
15
Main Subjects
Abstract EN
Demand forecasting is one of the main issues of supply chains.
It aimed to optimize stocks, reduce costs, and increase sales, profit, and customer loyalty.
For this purpose, historical data can be analyzed to improve demand forecasting by using various methods like machine learning techniques, time series analysis, and deep learning models.
In this work, an intelligent demand forecasting system is developed.
This improved model is based on the analysis and interpretation of the historical data by using different forecasting methods which include time series analysis techniques, support vector regression algorithm, and deep learning models.
To the best of our knowledge, this is the first study to blend the deep learning methodology, support vector regression algorithm, and different time series analysis models by a novel decision integration strategy for demand forecasting approach.
The other novelty of this work is the adaptation of boosting ensemble strategy to demand forecasting system by implementing a novel decision integration model.
The developed system is applied and tested on real life data obtained from SOK Market in Turkey which operates as a fast-growing company with 6700 stores, 1500 products, and 23 distribution centers.
A wide range of comparative and extensive experiments demonstrate that the proposed demand forecasting system exhibits noteworthy results compared to the state-of-art studies.
Unlike the state-of-art studies, inclusion of support vector regression, deep learning model, and a novel integration strategy to the proposed forecasting system ensures significant accuracy improvement.
American Psychological Association (APA)
Kilimci, Zeynep Hilal& Akyuz, A. Okay& Uysal, Mitat& Akyokus, Selim& Uysal, M. Ozan& Atak Bulbul, Berna…[et al.]. 2019. An Improved Demand Forecasting Model Using Deep Learning Approach and Proposed Decision Integration Strategy for Supply Chain. Complexity،Vol. 2019, no. 2019, pp.1-15.
https://search.emarefa.net/detail/BIM-1133121
Modern Language Association (MLA)
Kilimci, Zeynep Hilal…[et al.]. An Improved Demand Forecasting Model Using Deep Learning Approach and Proposed Decision Integration Strategy for Supply Chain. Complexity No. 2019 (2019), pp.1-15.
https://search.emarefa.net/detail/BIM-1133121
American Medical Association (AMA)
Kilimci, Zeynep Hilal& Akyuz, A. Okay& Uysal, Mitat& Akyokus, Selim& Uysal, M. Ozan& Atak Bulbul, Berna…[et al.]. An Improved Demand Forecasting Model Using Deep Learning Approach and Proposed Decision Integration Strategy for Supply Chain. Complexity. 2019. Vol. 2019, no. 2019, pp.1-15.
https://search.emarefa.net/detail/BIM-1133121
Data Type
Journal Articles
Language
English
Notes
Includes bibliographical references
Record ID
BIM-1133121