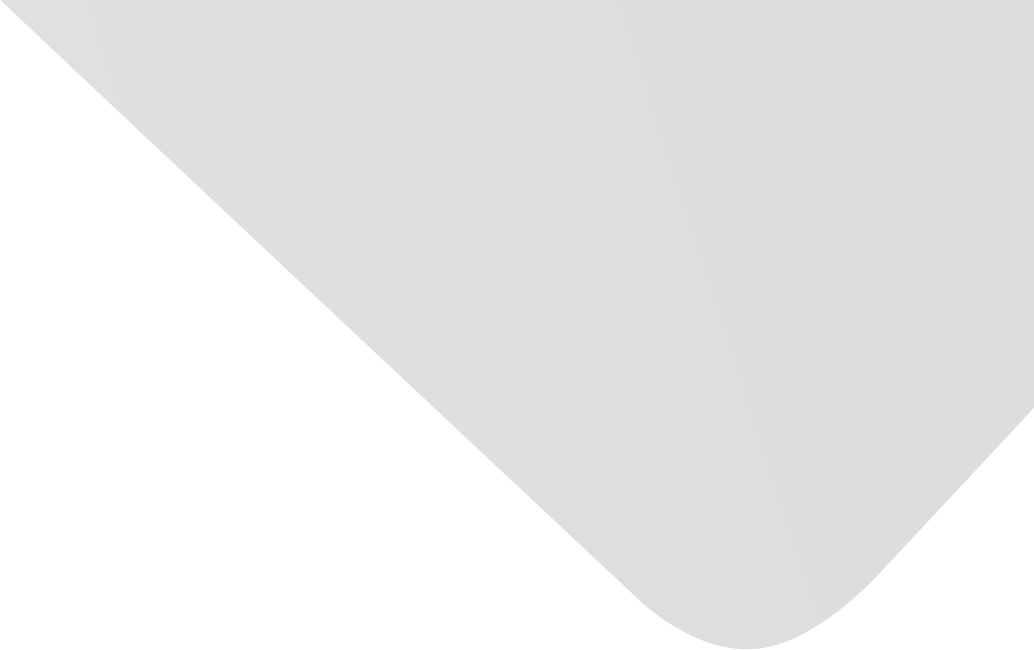
Medical Image Classification Algorithm Based on Weight Initialization-Sliding Window Fusion Convolutional Neural Network
Author
Source
Issue
Vol. 2019, Issue 2019 (31 Dec. 2019), pp.1-15, 15 p.
Publisher
Hindawi Publishing Corporation
Publication Date
2019-10-20
Country of Publication
Egypt
No. of Pages
15
Main Subjects
Abstract EN
Due to the complexity of medical images, traditional medical image classification methods have been unable to meet actual application needs.
In recent years, the rapid development of deep learning theory has provided a technical approach for solving medical image classification tasks.
However, deep learning has the following problems in medical image classification.
First, it is impossible to construct a deep learning model hierarchy for medical image properties; second, the network initialization weights of deep learning models are not well optimized.
Therefore, this paper starts from the perspective of network optimization and improves the nonlinear modeling ability of the network through optimization methods.
A new network weight initialization method is proposed, which alleviates the problem that existing deep learning model initialization is limited by the type of the nonlinear unit adopted and increases the potential of the neural network to handle different visual tasks.
Moreover, through an in-depth study of the multicolumn convolutional neural network framework, this paper finds that the number of features and the convolution kernel size at different levels of the convolutional neural network are different.
In contrast, the proposed method can construct different convolutional neural network models that adapt better to the characteristics of the medical images of interest and thus can better train the resulting heterogeneous multicolumn convolutional neural networks.
Finally, using the adaptive sliding window fusion mechanism proposed in this paper, both methods jointly complete the classification task of medical images.
Based on the above ideas, this paper proposes a medical classification algorithm based on a weight initialization/sliding window fusion for multilevel convolutional neural networks.
The methods proposed in this study were applied to breast mass, brain tumor tissue, and medical image database classification experiments.
The results show that the proposed method not only achieves a higher average accuracy than that of traditional machine learning and other deep learning methods but also is more stable and more robust.
American Psychological Association (APA)
An, Feng-Ping. 2019. Medical Image Classification Algorithm Based on Weight Initialization-Sliding Window Fusion Convolutional Neural Network. Complexity،Vol. 2019, no. 2019, pp.1-15.
https://search.emarefa.net/detail/BIM-1133141
Modern Language Association (MLA)
An, Feng-Ping. Medical Image Classification Algorithm Based on Weight Initialization-Sliding Window Fusion Convolutional Neural Network. Complexity No. 2019 (2019), pp.1-15.
https://search.emarefa.net/detail/BIM-1133141
American Medical Association (AMA)
An, Feng-Ping. Medical Image Classification Algorithm Based on Weight Initialization-Sliding Window Fusion Convolutional Neural Network. Complexity. 2019. Vol. 2019, no. 2019, pp.1-15.
https://search.emarefa.net/detail/BIM-1133141
Data Type
Journal Articles
Language
English
Notes
Includes bibliographical references
Record ID
BIM-1133141