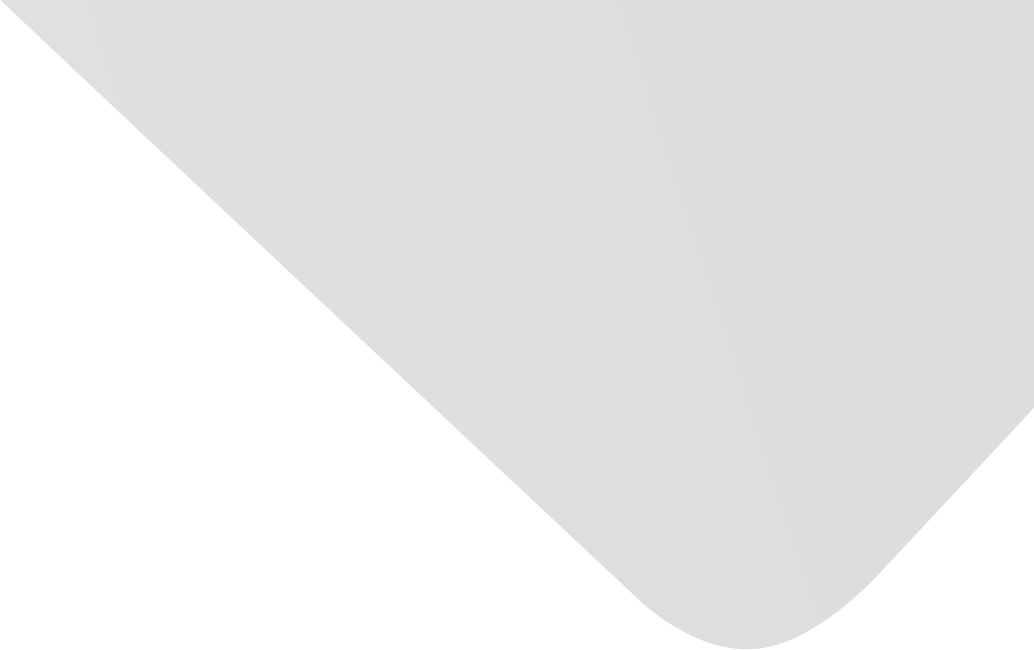
Focal CTC Loss for Chinese Optical Character Recognition on Unbalanced Datasets
Joint Authors
Zhang, Shengping
Feng, Xinjie
Yao, Hongxun
Source
Issue
Vol. 2019, Issue 2019 (31 Dec. 2019), pp.1-11, 11 p.
Publisher
Hindawi Publishing Corporation
Publication Date
2019-01-02
Country of Publication
Egypt
No. of Pages
11
Main Subjects
Abstract EN
In this paper, we propose a novel deep model for unbalanced distribution Character Recognition by employing focal loss based connectionist temporal classification (CTC) function.
Previous works utilize Traditional CTC to compute prediction losses.
However, some datasets may consist of extremely unbalanced samples, such as Chinese.
In other words, both training and testing sets contain large amounts of low-frequent samples.
The low-frequent samples have very limited influence on the model during training.
To solve this issue, we modify the traditional CTC by fusing focal loss with it and thus make the model attend to the low-frequent samples at training stage.
In order to demonstrate the advantage of the proposed method, we conduct experiments on two types of datasets: synthetic and real image sequence datasets.
The results on both datasets demonstrate that the proposed focal CTC loss function achieves desired performance on unbalanced datasets.
Specifically, our method outperforms traditional CTC by 3 to 9 percentages in accuracy on average.
American Psychological Association (APA)
Feng, Xinjie& Yao, Hongxun& Zhang, Shengping. 2019. Focal CTC Loss for Chinese Optical Character Recognition on Unbalanced Datasets. Complexity،Vol. 2019, no. 2019, pp.1-11.
https://search.emarefa.net/detail/BIM-1133203
Modern Language Association (MLA)
Feng, Xinjie…[et al.]. Focal CTC Loss for Chinese Optical Character Recognition on Unbalanced Datasets. Complexity No. 2019 (2019), pp.1-11.
https://search.emarefa.net/detail/BIM-1133203
American Medical Association (AMA)
Feng, Xinjie& Yao, Hongxun& Zhang, Shengping. Focal CTC Loss for Chinese Optical Character Recognition on Unbalanced Datasets. Complexity. 2019. Vol. 2019, no. 2019, pp.1-11.
https://search.emarefa.net/detail/BIM-1133203
Data Type
Journal Articles
Language
English
Notes
Includes bibliographical references
Record ID
BIM-1133203