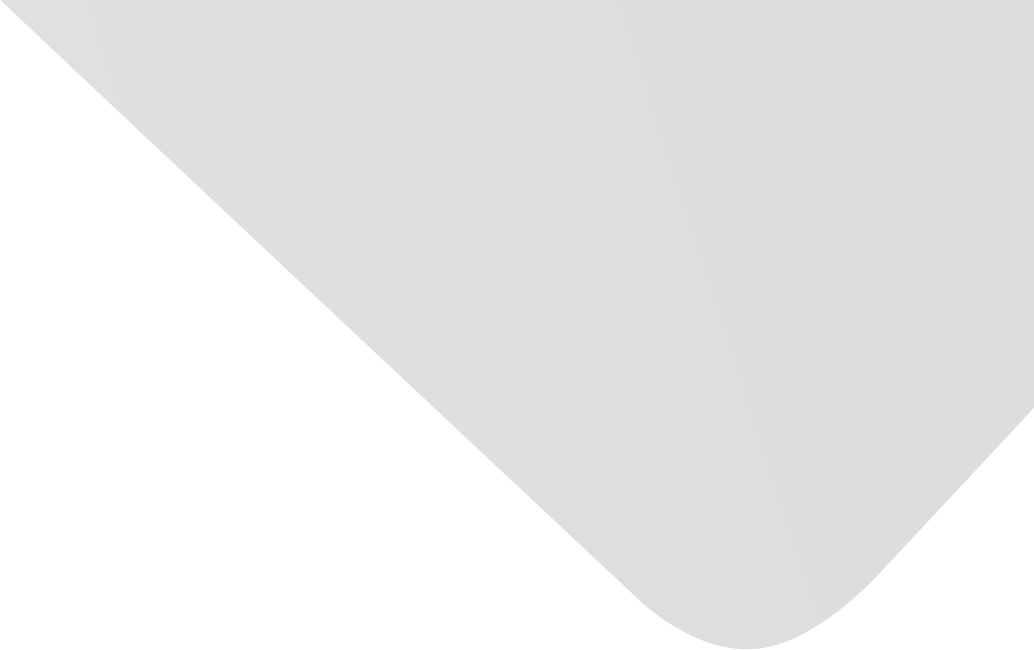
Machine Learning Supports Long Noncoding RNAs as Expression Markers for Endometrial Carcinoma
Joint Authors
Matte, Ursula
Falcon, Tiago
Freitas, Martiela
Mello, Ana Carolina
Coutinho, Laura
Source
Issue
Vol. 2020, Issue 2020 (31 Dec. 2020), pp.1-12, 12 p.
Publisher
Hindawi Publishing Corporation
Publication Date
2020-01-10
Country of Publication
Egypt
No. of Pages
12
Main Subjects
Abstract EN
Uterine corpus endometrial carcinoma (UCEC) is the second most common type of gynecological tumor.
Several research studies have recently shown the potential of different ncRNAs as biomarkers for prognostics and diagnosis in different types of cancers, including UCEC.
Thus, we hypothesized that long noncoding RNAs (lncRNAs) could serve as efficient factors to discriminate solid primary (TP) and normal adjacent (NT) tissues in UCEC with high accuracy.
We performed an in silico differential expression analysis comparing TP and NT from a set of samples downloaded from the Cancer Genome Atlas (TCGA) database, targeting highly differentially expressed lncRNAs that could potentially serve as gene expression markers.
All analyses were performed in R software.
The receiver operator characteristics (ROC) analyses and both supervised and unsupervised machine learning indicated a set of 14 lncRNAs that may serve as biomarkers for UCEC.
Functions and putative pathways were assessed through a coexpression network and target enrichment analysis.
American Psychological Association (APA)
Mello, Ana Carolina& Freitas, Martiela& Coutinho, Laura& Falcon, Tiago& Matte, Ursula. 2020. Machine Learning Supports Long Noncoding RNAs as Expression Markers for Endometrial Carcinoma. BioMed Research International،Vol. 2020, no. 2020, pp.1-12.
https://search.emarefa.net/detail/BIM-1133579
Modern Language Association (MLA)
Mello, Ana Carolina…[et al.]. Machine Learning Supports Long Noncoding RNAs as Expression Markers for Endometrial Carcinoma. BioMed Research International No. 2020 (2020), pp.1-12.
https://search.emarefa.net/detail/BIM-1133579
American Medical Association (AMA)
Mello, Ana Carolina& Freitas, Martiela& Coutinho, Laura& Falcon, Tiago& Matte, Ursula. Machine Learning Supports Long Noncoding RNAs as Expression Markers for Endometrial Carcinoma. BioMed Research International. 2020. Vol. 2020, no. 2020, pp.1-12.
https://search.emarefa.net/detail/BIM-1133579
Data Type
Journal Articles
Language
English
Notes
Includes bibliographical references
Record ID
BIM-1133579