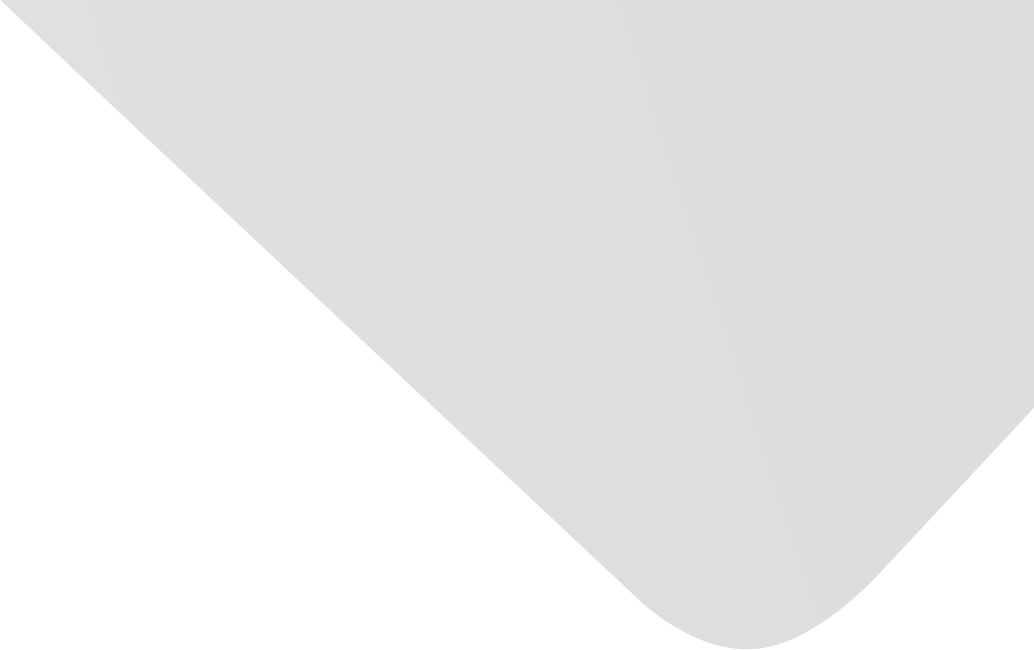
Convolutional Recurrent Neural Network for Fault Diagnosis of High-Speed Train Bogie
Joint Authors
Huang, Deqing
Liang, Kaiwei
Qin, Na
Fu, Yuanzhe
Source
Issue
Vol. 2018, Issue 2018 (31 Dec. 2018), pp.1-13, 13 p.
Publisher
Hindawi Publishing Corporation
Publication Date
2018-10-23
Country of Publication
Egypt
No. of Pages
13
Main Subjects
Abstract EN
Timely detection and efficient recognition of fault are challenging for the bogie of high-speed train (HST), owing to the fact that different types of fault signals have similar characteristics in the same frequency range.
Notice that convolutional neural networks (CNNs) are powerful in extracting high-level local features and that recurrent neural networks (RNNs) are capable of learning long-term context dependencies in vibration signals.
In this paper, by combining CNN and RNN, a so-called convolutional recurrent neural network (CRNN) is proposed to diagnose various faults of the HST bogie, where the capabilities of CNN and RNN are inherited simultaneously.
Within the novel architecture, the proposed CRNN first filters out the features from the original data through convolutional layers.
Then, four recurrent layers with simple recurrent cell are used to model the context information in the extracted features.
By comparing the performance of the presented CRNN with CNN, RNN, and ensemble learning, experimental results show that CRNN achieves not only the best performance with accuracy of 97.8% but also the least time spent in training model.
American Psychological Association (APA)
Liang, Kaiwei& Qin, Na& Huang, Deqing& Fu, Yuanzhe. 2018. Convolutional Recurrent Neural Network for Fault Diagnosis of High-Speed Train Bogie. Complexity،Vol. 2018, no. 2018, pp.1-13.
https://search.emarefa.net/detail/BIM-1134147
Modern Language Association (MLA)
Liang, Kaiwei…[et al.]. Convolutional Recurrent Neural Network for Fault Diagnosis of High-Speed Train Bogie. Complexity No. 2018 (2018), pp.1-13.
https://search.emarefa.net/detail/BIM-1134147
American Medical Association (AMA)
Liang, Kaiwei& Qin, Na& Huang, Deqing& Fu, Yuanzhe. Convolutional Recurrent Neural Network for Fault Diagnosis of High-Speed Train Bogie. Complexity. 2018. Vol. 2018, no. 2018, pp.1-13.
https://search.emarefa.net/detail/BIM-1134147
Data Type
Journal Articles
Language
English
Notes
Includes bibliographical references
Record ID
BIM-1134147