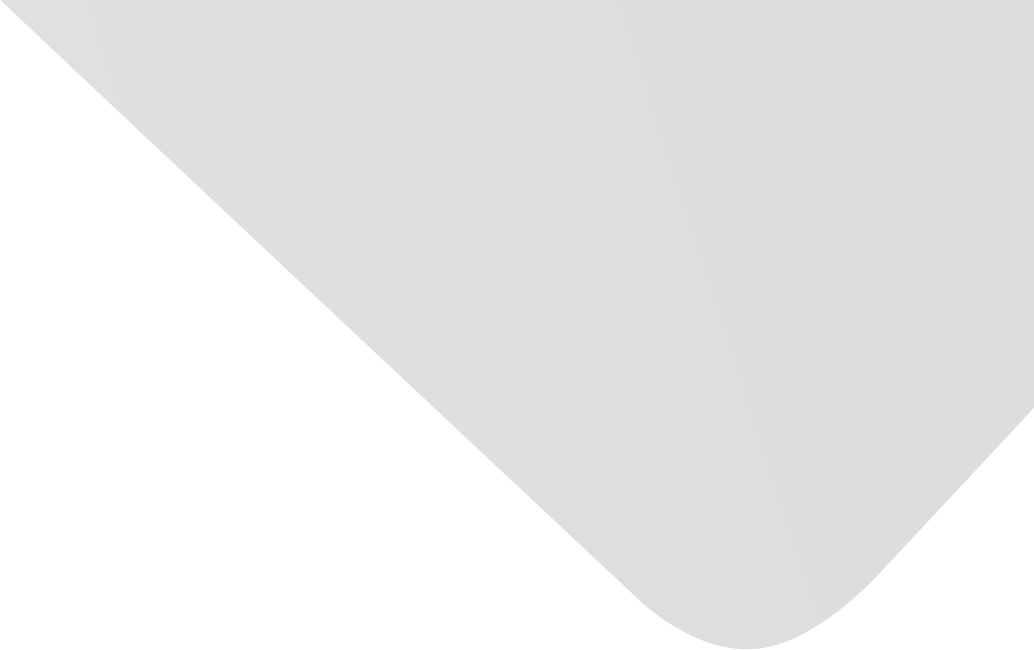
A Pervasive Approach to EEG-Based Depression Detection
Joint Authors
Chen, Yiqiang
Hu, Bin
Cai, Hanshu
Han, Jiashuo
Chen, Yunfei
Sha, Xiaocong
Wang, Ziyang
Yang, Jing
Feng, Lei
Ding, Zhijie
Gutknecht, Jürg
Source
Issue
Vol. 2018, Issue 2018 (31 Dec. 2018), pp.1-13, 13 p.
Publisher
Hindawi Publishing Corporation
Publication Date
2018-02-06
Country of Publication
Egypt
No. of Pages
13
Main Subjects
Abstract EN
Nowadays, depression is the world’s major health concern and economic burden worldwide.
However, due to the limitations of current methods for depression diagnosis, a pervasive and objective approach is essential.
In the present study, a psychophysiological database, containing 213 (92 depressed patients and 121 normal controls) subjects, was constructed.
The electroencephalogram (EEG) signals of all participants under resting state and sound stimulation were collected using a pervasive prefrontal-lobe three-electrode EEG system at Fp1, Fp2, and Fpz electrode sites.
After denoising using the Finite Impulse Response filter combining the Kalman derivation formula, Discrete Wavelet Transformation, and an Adaptive Predictor Filter, a total of 270 linear and nonlinear features were extracted.
Then, the minimal-redundancy-maximal-relevance feature selection technique reduced the dimensionality of the feature space.
Four classification methods (Support Vector Machine, K-Nearest Neighbor, Classification Trees, and Artificial Neural Network) distinguished the depressed participants from normal controls.
The classifiers’ performances were evaluated using 10-fold cross-validation.
The results showed that K-Nearest Neighbor (KNN) had the highest accuracy of 79.27%.
The result also suggested that the absolute power of the theta wave might be a valid characteristic for discriminating depression.
This study proves the feasibility of a pervasive three-electrode EEG acquisition system for depression diagnosis.
American Psychological Association (APA)
Cai, Hanshu& Han, Jiashuo& Chen, Yunfei& Sha, Xiaocong& Wang, Ziyang& Hu, Bin…[et al.]. 2018. A Pervasive Approach to EEG-Based Depression Detection. Complexity،Vol. 2018, no. 2018, pp.1-13.
https://search.emarefa.net/detail/BIM-1134572
Modern Language Association (MLA)
Cai, Hanshu…[et al.]. A Pervasive Approach to EEG-Based Depression Detection. Complexity No. 2018 (2018), pp.1-13.
https://search.emarefa.net/detail/BIM-1134572
American Medical Association (AMA)
Cai, Hanshu& Han, Jiashuo& Chen, Yunfei& Sha, Xiaocong& Wang, Ziyang& Hu, Bin…[et al.]. A Pervasive Approach to EEG-Based Depression Detection. Complexity. 2018. Vol. 2018, no. 2018, pp.1-13.
https://search.emarefa.net/detail/BIM-1134572
Data Type
Journal Articles
Language
English
Notes
Includes bibliographical references
Record ID
BIM-1134572