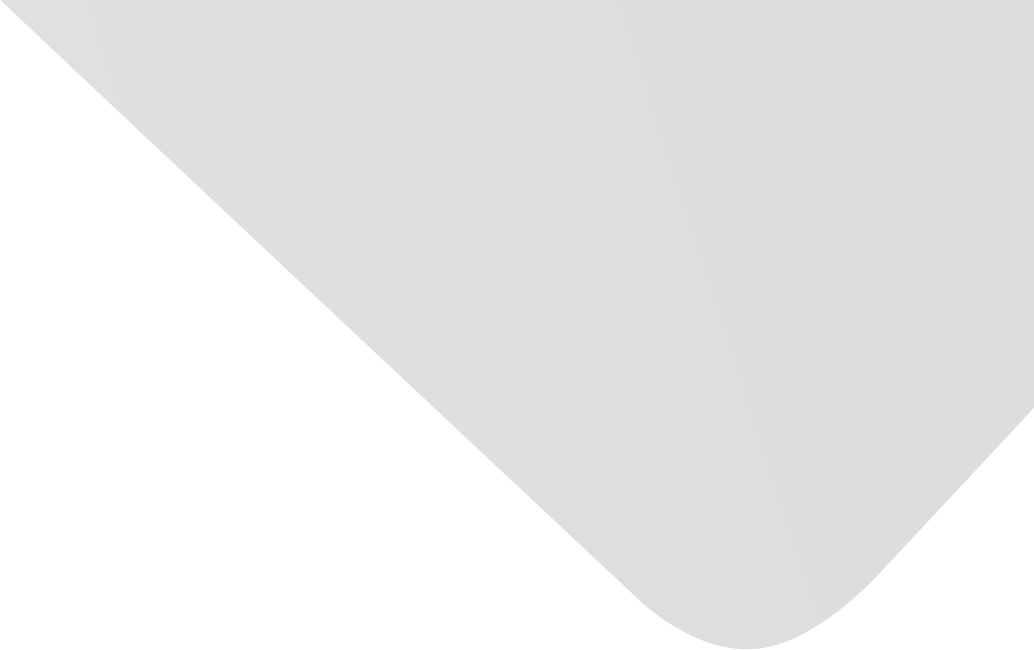
Evaluation for Sortie Generation Capacity of the Carrier Aircraft Based on the Variable Structure RBF Neural Network with the Fast Learning Rate
Joint Authors
Luan, Tiantian
Sun, Mingxiao
Chen, Daidai
Xia, Guoqing
Source
Issue
Vol. 2018, Issue 2018 (31 Dec. 2018), pp.1-19, 19 p.
Publisher
Hindawi Publishing Corporation
Publication Date
2018-10-22
Country of Publication
Egypt
No. of Pages
19
Main Subjects
Abstract EN
The neural network has the advantages of self-learning, self-adaptation, and fault tolerance.
It can establish a qualitative and quantitative evaluation model which is closer to human thought patterns.
However, the structure and the convergence rate of the radial basis function (RBF) neural network need to be improved.
This paper proposes a new variable structure radial basis function (VS-RBF) with a fast learning rate, in order to solve the problem of structural optimization design and parameter learning algorithm for the radial basis function neural network.
The number of neurons in the hidden layer is adjusted by calculating the output information of neurons in the hidden layer and the multi-information between neurons in the hidden layer and output layer.
This method effectively solves the problem that the RBF neural network structure is too large or too small.
The convergence rate of the RBF neural network is improved by using the robust regression algorithm and the fast learning rate algorithm.
At the same time, the convergence analysis of the VS-RBF neural network is given to ensure the stability of the RBF neural network.
Compared with other self-organizing RBF neural networks (self-organizing RBF (SORBF) and rough RBF neural networks (RS-RBF)), VS-RBF has a more compact structure, faster dynamic response speed, and better generalization ability.
The simulations of approximating a typical nonlinear function, identifying UCI datasets, and evaluating sortie generation capacity of an carrier aircraft show the effectiveness of VS-RBF.
American Psychological Association (APA)
Luan, Tiantian& Sun, Mingxiao& Xia, Guoqing& Chen, Daidai. 2018. Evaluation for Sortie Generation Capacity of the Carrier Aircraft Based on the Variable Structure RBF Neural Network with the Fast Learning Rate. Complexity،Vol. 2018, no. 2018, pp.1-19.
https://search.emarefa.net/detail/BIM-1135558
Modern Language Association (MLA)
Luan, Tiantian…[et al.]. Evaluation for Sortie Generation Capacity of the Carrier Aircraft Based on the Variable Structure RBF Neural Network with the Fast Learning Rate. Complexity No. 2018 (2018), pp.1-19.
https://search.emarefa.net/detail/BIM-1135558
American Medical Association (AMA)
Luan, Tiantian& Sun, Mingxiao& Xia, Guoqing& Chen, Daidai. Evaluation for Sortie Generation Capacity of the Carrier Aircraft Based on the Variable Structure RBF Neural Network with the Fast Learning Rate. Complexity. 2018. Vol. 2018, no. 2018, pp.1-19.
https://search.emarefa.net/detail/BIM-1135558
Data Type
Journal Articles
Language
English
Notes
Includes bibliographical references
Record ID
BIM-1135558