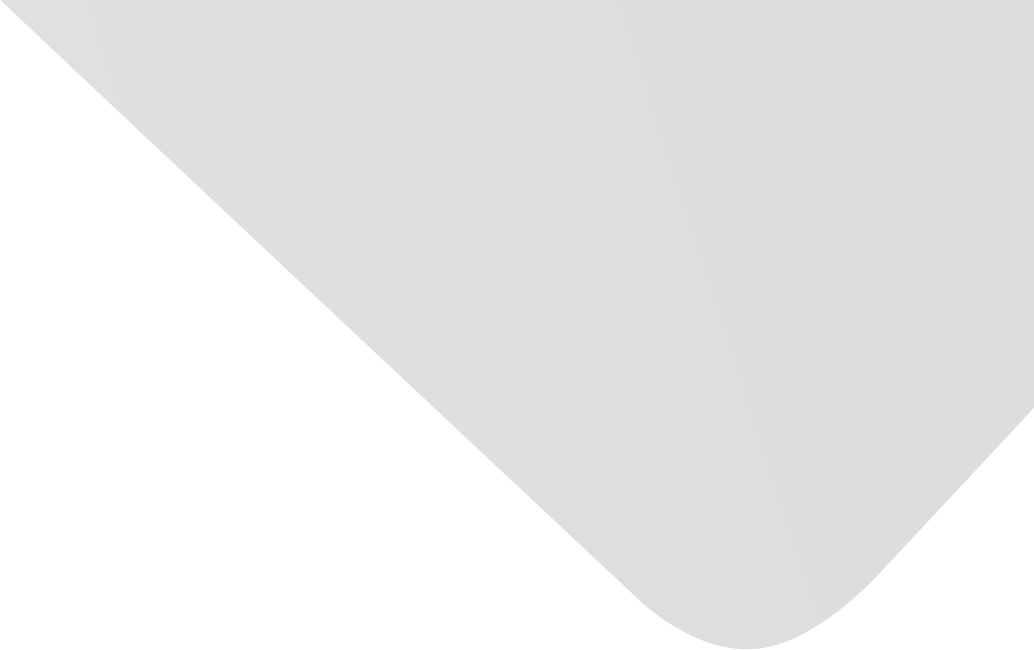
An Approach to Data Reduction for Learning from Big Datasets: Integrating Stacking, Rotation, and Agent Population Learning Techniques
Joint Authors
Czarnowski, Ireneusz
Jędrzejowicz, Piotr
Source
Issue
Vol. 2018, Issue 2018 (31 Dec. 2018), pp.1-13, 13 p.
Publisher
Hindawi Publishing Corporation
Publication Date
2018-11-05
Country of Publication
Egypt
No. of Pages
13
Main Subjects
Abstract EN
In the paper, several data reduction techniques for machine learning from big datasets are discussed and evaluated.
The discussed approach focuses on combining several techniques including stacking, rotation, and data reduction aimed at improving the performance of the machine classification.
Stacking is seen as the technique allowing to take advantage of the multiple classification models.
The rotation-based techniques are used to increase the heterogeneity of the stacking ensembles.
Data reduction makes it possible to classify instances belonging to big datasets.
We propose to use an agent-based population learning algorithm for data reduction in the feature and instance dimensions.
For diversification of the classifier ensembles within the rotation also, alternatively, principal component analysis and independent component analysis are used.
The research question addressed in the paper is formulated as follows: does the performance of a classifier using the reduced dataset be improved by integrating the data reduction mechanism with the rotation-based technique and the stacking?
American Psychological Association (APA)
Czarnowski, Ireneusz& Jędrzejowicz, Piotr. 2018. An Approach to Data Reduction for Learning from Big Datasets: Integrating Stacking, Rotation, and Agent Population Learning Techniques. Complexity،Vol. 2018, no. 2018, pp.1-13.
https://search.emarefa.net/detail/BIM-1135796
Modern Language Association (MLA)
Czarnowski, Ireneusz& Jędrzejowicz, Piotr. An Approach to Data Reduction for Learning from Big Datasets: Integrating Stacking, Rotation, and Agent Population Learning Techniques. Complexity No. 2018 (2018), pp.1-13.
https://search.emarefa.net/detail/BIM-1135796
American Medical Association (AMA)
Czarnowski, Ireneusz& Jędrzejowicz, Piotr. An Approach to Data Reduction for Learning from Big Datasets: Integrating Stacking, Rotation, and Agent Population Learning Techniques. Complexity. 2018. Vol. 2018, no. 2018, pp.1-13.
https://search.emarefa.net/detail/BIM-1135796
Data Type
Journal Articles
Language
English
Notes
Includes bibliographical references
Record ID
BIM-1135796