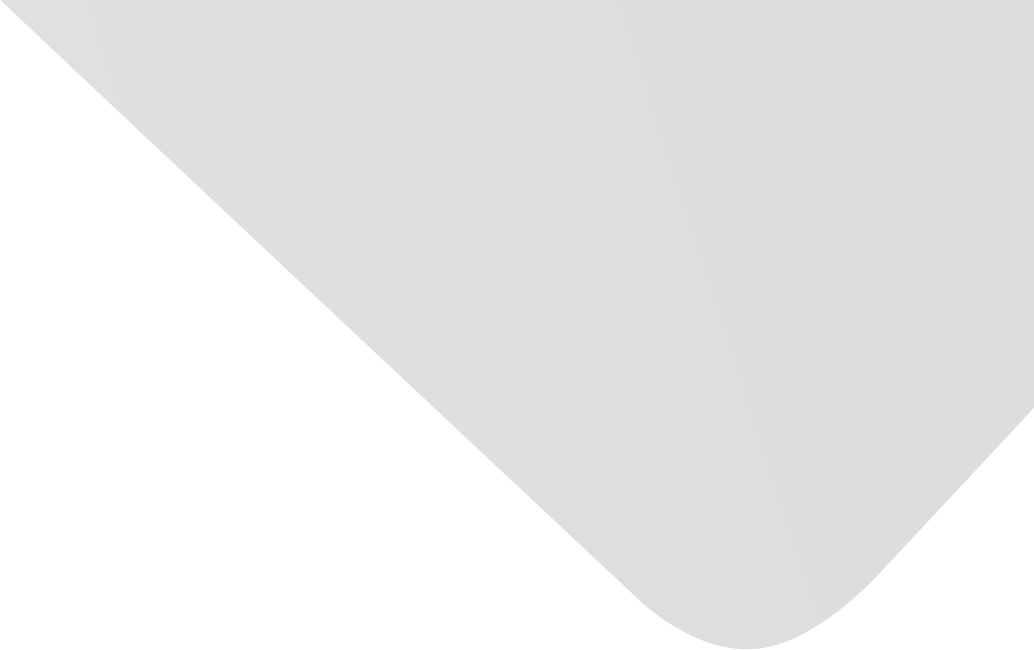
Robust Semisupervised Nonnegative Local Coordinate Factorization for Data Representation
Joint Authors
Lv, Qian
Yan, Chenggang
Tang, Kewei
Zhang, Jie
Wei, Jiang
Source
Issue
Vol. 2018, Issue 2018 (31 Dec. 2018), pp.1-16, 16 p.
Publisher
Hindawi Publishing Corporation
Publication Date
2018-08-01
Country of Publication
Egypt
No. of Pages
16
Main Subjects
Abstract EN
Obtaining an optimum data representation is a challenging issue that arises in many intellectual data processing techniques such as data mining, pattern recognition, and gene clustering.
Many existing methods formulate this problem as a nonnegative matrix factorization (NMF) approximation problem.
The standard NMF uses the least square loss function, which is not robust to outlier points and noises and fails to utilize prior label information to enhance the discriminability of representations.
In this study, we develop a novel matrix factorization method called robust semisupervised nonnegative local coordinate factorization by integrating robust NMF, a robust local coordinate constraint, and local spline regression into a unified framework.
We use the l2,1 norm for the loss function of the NMF and a local coordinate constraint term to make our method insensitive to outlier points and noises.
In addition, we exploit the local and global consistencies of sample labels to guarantee that data representation is compact and discriminative.
An efficient multiplicative updating algorithm is deduced to solve the novel loss function, followed by a strict proof of the convergence.
Several experiments conducted in this study on face and gene datasets clearly indicate that the proposed method is more effective and robust compared to the state-of-the-art methods.
American Psychological Association (APA)
Wei, Jiang& Lv, Qian& Yan, Chenggang& Tang, Kewei& Zhang, Jie. 2018. Robust Semisupervised Nonnegative Local Coordinate Factorization for Data Representation. Complexity،Vol. 2018, no. 2018, pp.1-16.
https://search.emarefa.net/detail/BIM-1135971
Modern Language Association (MLA)
Wei, Jiang…[et al.]. Robust Semisupervised Nonnegative Local Coordinate Factorization for Data Representation. Complexity No. 2018 (2018), pp.1-16.
https://search.emarefa.net/detail/BIM-1135971
American Medical Association (AMA)
Wei, Jiang& Lv, Qian& Yan, Chenggang& Tang, Kewei& Zhang, Jie. Robust Semisupervised Nonnegative Local Coordinate Factorization for Data Representation. Complexity. 2018. Vol. 2018, no. 2018, pp.1-16.
https://search.emarefa.net/detail/BIM-1135971
Data Type
Journal Articles
Language
English
Notes
Includes bibliographical references
Record ID
BIM-1135971