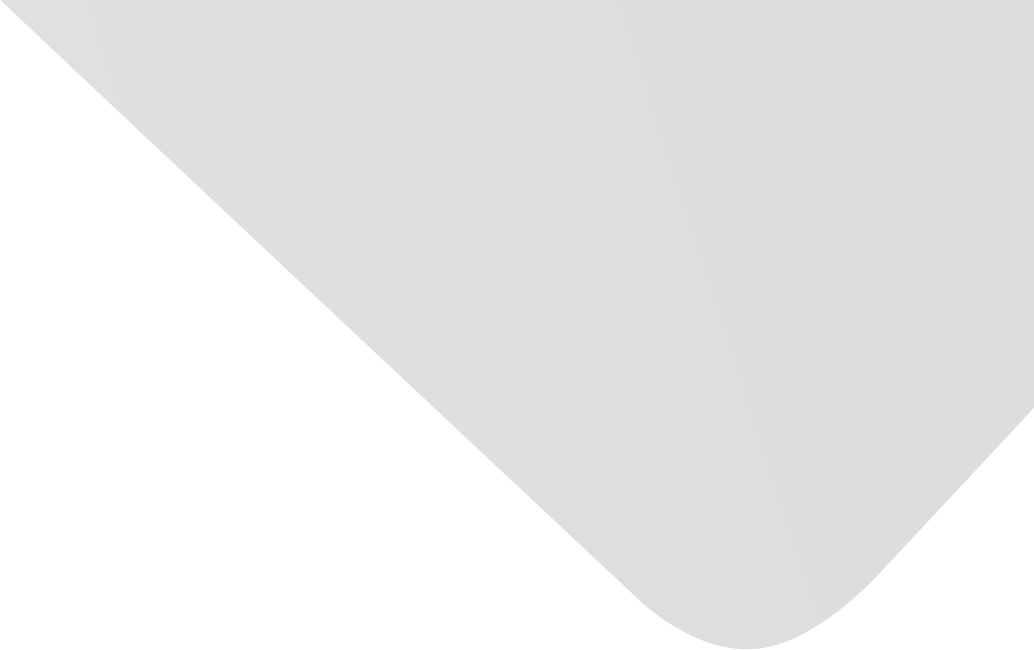
An Extreme Learning Machine-Based Community Detection Algorithm in Complex Networks
Joint Authors
Wang, Feifan
Chai, Senchun
Xia, Yuanqing
Zhang, Baihai
Source
Issue
Vol. 2018, Issue 2018 (31 Dec. 2018), pp.1-10, 10 p.
Publisher
Hindawi Publishing Corporation
Publication Date
2018-08-06
Country of Publication
Egypt
No. of Pages
10
Main Subjects
Abstract EN
Community structure, one of the most popular properties in complex networks, has long been a cornerstone in the advance of various scientific branches.
Over the past few years, a number of tools have been used in the development of community detection algorithms.
In this paper, by means of fusing unsupervised extreme learning machines and the k-means clustering techniques, we propose a novel community detection method that surpasses traditional k-means approaches in terms of precision and stability while adding very few extra computational costs.
Furthermore, results of extensive experiments undertaken on computer-generated networks and real-world datasets illustrate acceptable performances of the introduced algorithm in comparison with other typical community detection algorithms.
American Psychological Association (APA)
Wang, Feifan& Zhang, Baihai& Chai, Senchun& Xia, Yuanqing. 2018. An Extreme Learning Machine-Based Community Detection Algorithm in Complex Networks. Complexity،Vol. 2018, no. 2018, pp.1-10.
https://search.emarefa.net/detail/BIM-1136013
Modern Language Association (MLA)
Wang, Feifan…[et al.]. An Extreme Learning Machine-Based Community Detection Algorithm in Complex Networks. Complexity No. 2018 (2018), pp.1-10.
https://search.emarefa.net/detail/BIM-1136013
American Medical Association (AMA)
Wang, Feifan& Zhang, Baihai& Chai, Senchun& Xia, Yuanqing. An Extreme Learning Machine-Based Community Detection Algorithm in Complex Networks. Complexity. 2018. Vol. 2018, no. 2018, pp.1-10.
https://search.emarefa.net/detail/BIM-1136013
Data Type
Journal Articles
Language
English
Notes
Includes bibliographical references
Record ID
BIM-1136013