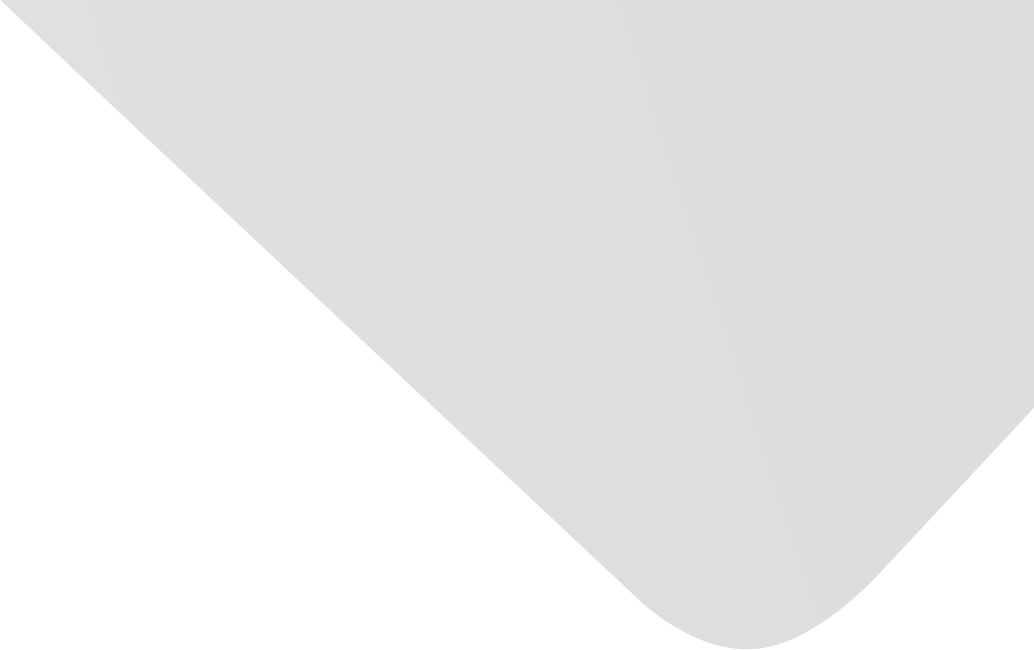
Automatic Sleep Stage Classification Based on Convolutional Neural Network and Fine-Grained Segments
Joint Authors
Cui, Zhihong
Zheng, Xiangwei
Shao, Xuexiao
Cui, Lizhen
Source
Issue
Vol. 2018, Issue 2018 (31 Dec. 2018), pp.1-13, 13 p.
Publisher
Hindawi Publishing Corporation
Publication Date
2018-10-08
Country of Publication
Egypt
No. of Pages
13
Main Subjects
Abstract EN
Sleep stage classification plays an important role in the diagnosis of sleep-related diseases.
However, traditional automatic sleep stage classification is quite challenging because of the complexity associated with the establishment of mathematical models and the extraction of handcrafted features.
In addition, the rapid fluctuations between sleep stages often result in blurry feature extraction, which might lead to an inaccurate assessment of electroencephalography (EEG) sleep stages.
Hence, we propose an automatic sleep stage classification method based on a convolutional neural network (CNN) combined with the fine-grained segment in multiscale entropy.
First, we define every 30 seconds of the multichannel EEG signal as a segment.
Then, we construct an input time series based on the fine-grained segments, which means that the posterior and current segments are reorganized as an input containing several segments and the size of the time series is decided based on the scale chosen depending on the fine-grained segments.
Next, each segment in this series is individually put into the designed CNN and feature maps are obtained after two blocks of convolution and max-pooling as well as a full-connected operation.
Finally, the results from the full-connected layer of each segment in the input time sequence are put into the softmax classifier together to get a single most likely sleep stage.
On a public dataset called ISRUC-Sleep, the average accuracy of our proposed method is 92.2%.
Moreover, it yields an accuracy of 90%, 86%, 93%, 97%, and 90% for stage W, stage N1, stage N2, stage N3, and stage REM, respectively.
Comparative analysis of performance suggests that the proposed method is better, as opposed to that of several state-of-the-art ones.
The sleep stage classification methods based on CNN and the fine-grained segments really improve a key step in the study of sleep disorders and expedite sleep research.
American Psychological Association (APA)
Cui, Zhihong& Zheng, Xiangwei& Shao, Xuexiao& Cui, Lizhen. 2018. Automatic Sleep Stage Classification Based on Convolutional Neural Network and Fine-Grained Segments. Complexity،Vol. 2018, no. 2018, pp.1-13.
https://search.emarefa.net/detail/BIM-1136596
Modern Language Association (MLA)
Cui, Zhihong…[et al.]. Automatic Sleep Stage Classification Based on Convolutional Neural Network and Fine-Grained Segments. Complexity No. 2018 (2018), pp.1-13.
https://search.emarefa.net/detail/BIM-1136596
American Medical Association (AMA)
Cui, Zhihong& Zheng, Xiangwei& Shao, Xuexiao& Cui, Lizhen. Automatic Sleep Stage Classification Based on Convolutional Neural Network and Fine-Grained Segments. Complexity. 2018. Vol. 2018, no. 2018, pp.1-13.
https://search.emarefa.net/detail/BIM-1136596
Data Type
Journal Articles
Language
English
Notes
Includes bibliographical references
Record ID
BIM-1136596