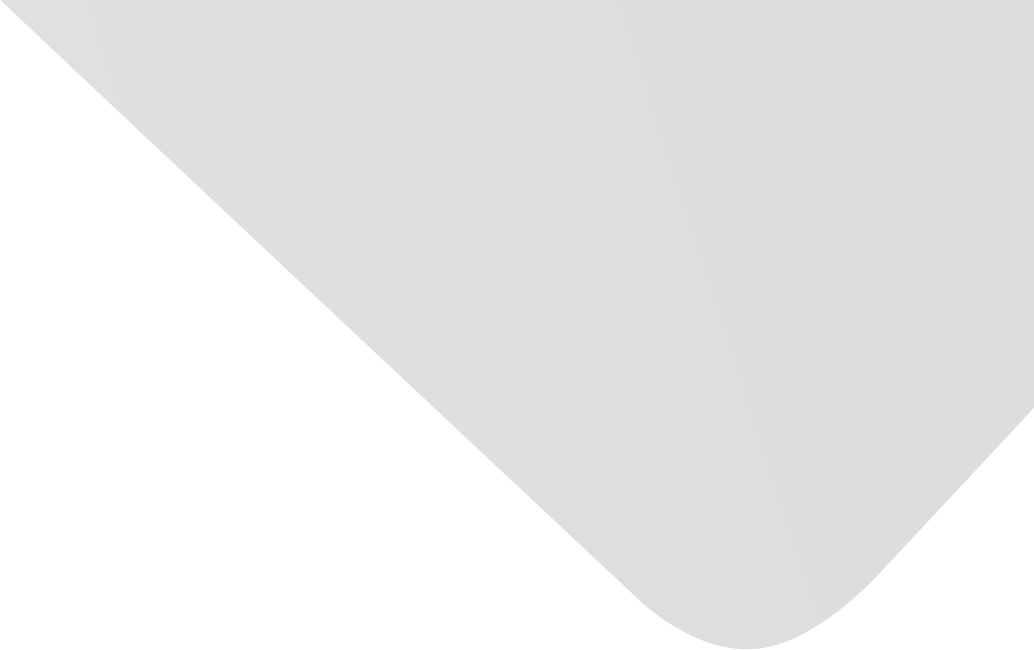
Using Deep Learning to Predict Complex Systems: A Case Study in Wind Farm Generation
Joint Authors
Source
Issue
Vol. 2018, Issue 2018 (31 Dec. 2018), pp.1-10, 10 p.
Publisher
Hindawi Publishing Corporation
Publication Date
2018-04-03
Country of Publication
Egypt
No. of Pages
10
Main Subjects
Abstract EN
Making every component of an electrical system work in unison is being made more challenging by the increasing number of renewable energies used, the electrical output of which is difficult to determine beforehand.
In Spain, the daily electricity market opens with a 12-hour lead time, where the supply and demand expected for the following 24 hours are presented.
When estimating the generation, energy sources like nuclear are highly stable, while peaking power plants can be run as necessary.
Renewable energies, however, which should eventually replace peakers insofar as possible, are reliant on meteorological conditions.
In this paper we propose using different deep-learning techniques and architectures to solve the problem of predicting wind generation in order to participate in the daily market, by making predictions 12 and 36 hours in advance.
We develop and compare various estimators based on feedforward, convolutional, and recurrent neural networks.
These estimators were trained and validated with data from a wind farm located on the island of Tenerife.
We show that the best candidates for each type are more precise than the reference estimator and the polynomial regression currently used at the wind farm.
We also conduct a sensitivity analysis to determine which estimator type is most robust to perturbations.
An analysis of our findings shows that the most accurate and robust estimators are those based on feedforward neural networks with a SELU activation function and convolutional neural networks.
American Psychological Association (APA)
Torres, J. M.& Aguilar, R. M.. 2018. Using Deep Learning to Predict Complex Systems: A Case Study in Wind Farm Generation. Complexity،Vol. 2018, no. 2018, pp.1-10.
https://search.emarefa.net/detail/BIM-1136681
Modern Language Association (MLA)
Torres, J. M.& Aguilar, R. M.. Using Deep Learning to Predict Complex Systems: A Case Study in Wind Farm Generation. Complexity No. 2018 (2018), pp.1-10.
https://search.emarefa.net/detail/BIM-1136681
American Medical Association (AMA)
Torres, J. M.& Aguilar, R. M.. Using Deep Learning to Predict Complex Systems: A Case Study in Wind Farm Generation. Complexity. 2018. Vol. 2018, no. 2018, pp.1-10.
https://search.emarefa.net/detail/BIM-1136681
Data Type
Journal Articles
Language
English
Notes
Includes bibliographical references
Record ID
BIM-1136681