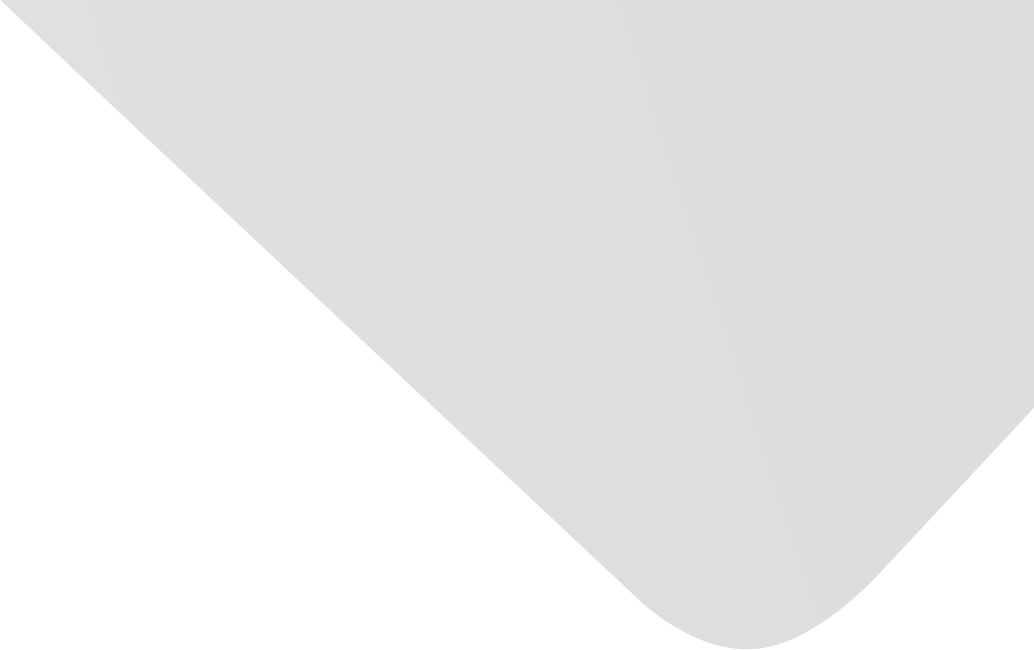
An Efficient and Effective Model to Handle Missing Data in Classification
Joint Authors
Ayatollahi, Seyyed Mohammad Taghi
Mehrabani-Zeinabad, Kamran
Doostfatemeh, Marziyeh
Source
Issue
Vol. 2020, Issue 2020 (31 Dec. 2020), pp.1-11, 11 p.
Publisher
Hindawi Publishing Corporation
Publication Date
2020-11-25
Country of Publication
Egypt
No. of Pages
11
Main Subjects
Abstract EN
Missing data is one of the most important causes in reduction of classification accuracy.
Many real datasets suffer from missing values, especially in medical sciences.
Imputation is a common way to deal with incomplete datasets.
There are various imputation methods that can be applied, and the choice of the best method depends on the dataset conditions such as sample size, missing percent, and missing mechanism.
Therefore, the better solution is to classify incomplete datasets without imputation and without any loss of information.
The structure of the “Bayesian additive regression trees” (BART) model is improved with the “Missingness Incorporated in Attributes” approach to solve its inefficiency in handling the missingness problem.
Implementation of MIA-within-BART is named “BART.m”.
As the abilities of BART.m are not investigated in classification of incomplete datasets, this simulation-based study aimed to provide such resource.
The results indicate that BART.m can be used even for datasets with 90 missing present and more importantly, it diagnoses the irrelevant variables and removes them by its own.
BART.m outperforms common models for classification with incomplete data, according to accuracy and computational time.
Based on the revealed properties, it can be said that BART.m is a high accuracy model in classification of incomplete datasets which avoids any assumptions and preprocess steps.
American Psychological Association (APA)
Mehrabani-Zeinabad, Kamran& Doostfatemeh, Marziyeh& Ayatollahi, Seyyed Mohammad Taghi. 2020. An Efficient and Effective Model to Handle Missing Data in Classification. BioMed Research International،Vol. 2020, no. 2020, pp.1-11.
https://search.emarefa.net/detail/BIM-1137664
Modern Language Association (MLA)
Mehrabani-Zeinabad, Kamran…[et al.]. An Efficient and Effective Model to Handle Missing Data in Classification. BioMed Research International No. 2020 (2020), pp.1-11.
https://search.emarefa.net/detail/BIM-1137664
American Medical Association (AMA)
Mehrabani-Zeinabad, Kamran& Doostfatemeh, Marziyeh& Ayatollahi, Seyyed Mohammad Taghi. An Efficient and Effective Model to Handle Missing Data in Classification. BioMed Research International. 2020. Vol. 2020, no. 2020, pp.1-11.
https://search.emarefa.net/detail/BIM-1137664
Data Type
Journal Articles
Language
English
Notes
Includes bibliographical references
Record ID
BIM-1137664