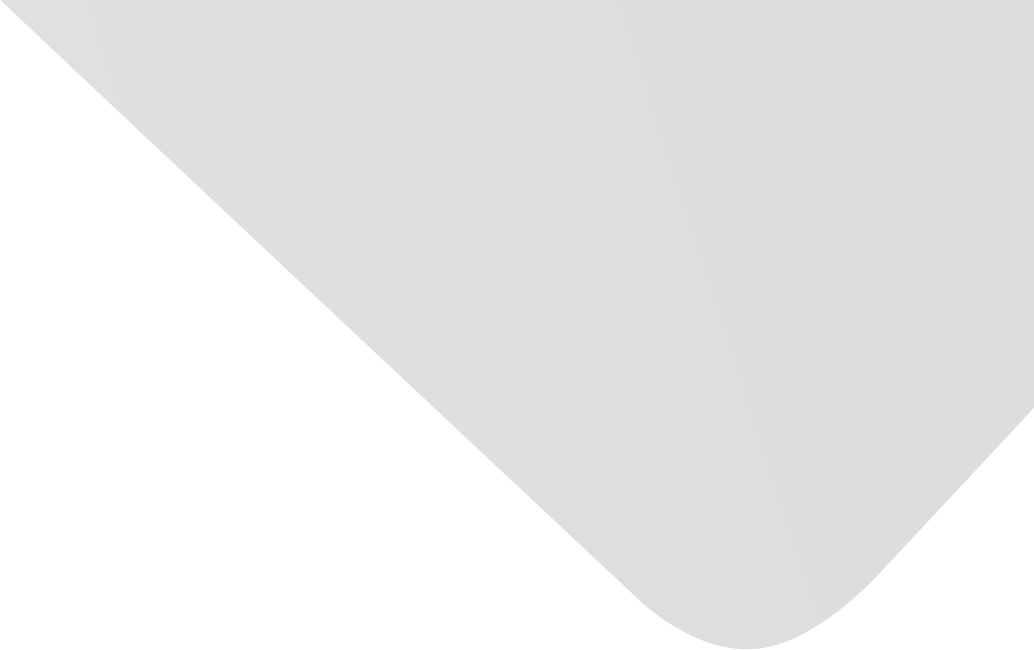
Deep Layer Kernel Sparse Representation Network for the Detection of Heart Valve Ailments from the Time-Frequency Representation of PCG Recordings
Joint Authors
Ghosh, Samit Kumar
Ponnalagu, R. N.
Tripathy, R. K.
Acharya, U. Rajendra
Source
Issue
Vol. 2020, Issue 2020 (31 Dec. 2020), pp.1-16, 16 p.
Publisher
Hindawi Publishing Corporation
Publication Date
2020-12-21
Country of Publication
Egypt
No. of Pages
16
Main Subjects
Abstract EN
The heart valve ailments (HVAs) are due to the defects in the valves of the heart and if untreated may cause heart failure, clots, and even sudden cardiac death.
Automated early detection of HVAs is necessary in the hospitals for proper diagnosis of pathological cases, to provide timely treatment, and to reduce the mortality rate.
The heart valve abnormalities will alter the heart sound and murmurs which can be faithfully captured by phonocardiogram (PCG) recordings.
In this paper, a time-frequency based deep layer kernel sparse representation network (DLKSRN) is proposed for the detection of various HVAs using PCG signals.
Spline kernel-based Chirplet transform (SCT) is used to evaluate the time-frequency representation of PCG recording, and the features like L1-norm (LN), sample entropy (SEN), and permutation entropy (PEN) are extracted from the different frequency components of the time-frequency representation of PCG recording.
The DLKSRN formulated using the hidden layers of extreme learning machine- (ELM-) autoencoders and kernel sparse representation (KSR) is used for the classification of PCG recordings as normal, and pathology cases such as mitral valve prolapse (MVP), mitral regurgitation (MR), aortic stenosis (AS), and mitral stenosis (MS).
The proposed approach has been evaluated using PCG recordings from both public and private databases, and the results demonstrated that an average sensitivity of 100%, 97.51%, 99.00%, 98.72%, and 99.13% are obtained for normal, MVP, MR, AS, and MS cases using the hold-out cross-validation (CV) method.
The proposed approach is applicable for the Internet of Things- (IoT-) driven smart healthcare system for the accurate detection of HVAs.
American Psychological Association (APA)
Ghosh, Samit Kumar& Ponnalagu, R. N.& Tripathy, R. K.& Acharya, U. Rajendra. 2020. Deep Layer Kernel Sparse Representation Network for the Detection of Heart Valve Ailments from the Time-Frequency Representation of PCG Recordings. BioMed Research International،Vol. 2020, no. 2020, pp.1-16.
https://search.emarefa.net/detail/BIM-1137739
Modern Language Association (MLA)
Ghosh, Samit Kumar…[et al.]. Deep Layer Kernel Sparse Representation Network for the Detection of Heart Valve Ailments from the Time-Frequency Representation of PCG Recordings. BioMed Research International No. 2020 (2020), pp.1-16.
https://search.emarefa.net/detail/BIM-1137739
American Medical Association (AMA)
Ghosh, Samit Kumar& Ponnalagu, R. N.& Tripathy, R. K.& Acharya, U. Rajendra. Deep Layer Kernel Sparse Representation Network for the Detection of Heart Valve Ailments from the Time-Frequency Representation of PCG Recordings. BioMed Research International. 2020. Vol. 2020, no. 2020, pp.1-16.
https://search.emarefa.net/detail/BIM-1137739
Data Type
Journal Articles
Language
English
Notes
Includes bibliographical references
Record ID
BIM-1137739