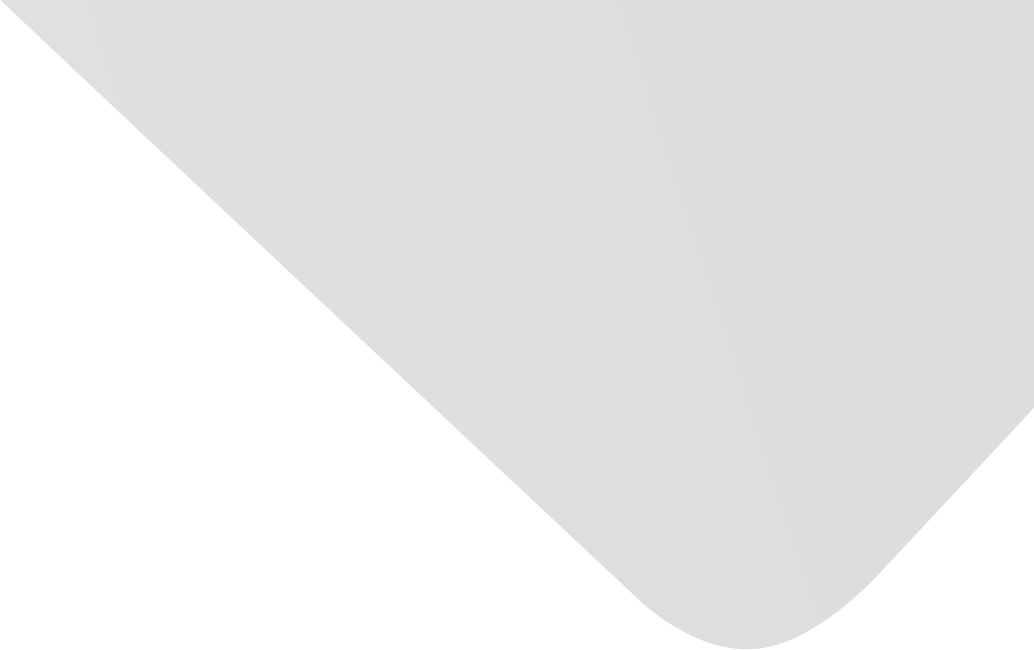
Machine Learning Based on a Multiparametric and Multiregional Radiomics Signature Predicts Radiotherapeutic Response in Patients with Glioblastoma
Joint Authors
Pan, Zi-Qi
Zhang, Shu-Jun
Wang, Xiang-Lian
Jiao, Yu-Xin
Qiu, Jian-Jian
Source
Issue
Vol. 2020, Issue 2020 (31 Dec. 2020), pp.1-12, 12 p.
Publisher
Hindawi Publishing Corporation
Publication Date
2020-10-24
Country of Publication
Egypt
No. of Pages
12
Main Subjects
Abstract EN
Background and Objective.
Although radiotherapy has become one of the main treatment methods for cancer, there is no noninvasive method to predict the radiotherapeutic response of individual glioblastoma (GBM) patients before surgery.
The purpose of this study is to develop and validate a machine learning-based radiomics signature to predict the radiotherapeutic response of GBM patients.
Methods.
The MRI images, genetic data, and clinical data of 152 patients with GBM were analyzed.
122 patients from the TCIA dataset (training set: n=82; validation set: n=40) and 30 patients from local hospitals were used as an independent test dataset.
Radiomics features were extracted from multiple regions of multiparameter MRI.
Kaplan-Meier survival analysis was used to verify the ability of the imaging signature to predict the response of GBM patients to radiotherapy before an operation.
Multivariate Cox regression including radiomics signature and preoperative clinical risk factors was used to further improve the ability to predict the overall survival (OS) of individual GBM patients, which was presented in the form of a nomogram.
Results.
The radiomics signature was built by eight selected features.
The C-index of the radiomics signature in the TCIA and independent test cohorts was 0.703 (P<0.001) and 0.757 (P=0.001), respectively.
Multivariate Cox regression analysis confirmed that the radiomics signature (HR: 0.290, P<0.001), age (HR: 1.023, P=0.01), and KPS (HR: 0.968, P<0.001) were independent risk factors for OS in GBM patients before surgery.
When the radiomics signature and preoperative clinical risk factors were combined, the radiomics nomogram further improved the performance of OS prediction in individual patients (C‐index=0.764 and 0.758 in the TCIA and test cohorts, respectively).
Conclusion.
This study developed a radiomics signature that can predict the response of individual GBM patients to radiotherapy and may be a new supplement for precise GBM radiotherapy.
American Psychological Association (APA)
Pan, Zi-Qi& Zhang, Shu-Jun& Wang, Xiang-Lian& Jiao, Yu-Xin& Qiu, Jian-Jian. 2020. Machine Learning Based on a Multiparametric and Multiregional Radiomics Signature Predicts Radiotherapeutic Response in Patients with Glioblastoma. Behavioural Neurology،Vol. 2020, no. 2020, pp.1-12.
https://search.emarefa.net/detail/BIM-1138362
Modern Language Association (MLA)
Pan, Zi-Qi…[et al.]. Machine Learning Based on a Multiparametric and Multiregional Radiomics Signature Predicts Radiotherapeutic Response in Patients with Glioblastoma. Behavioural Neurology No. 2020 (2020), pp.1-12.
https://search.emarefa.net/detail/BIM-1138362
American Medical Association (AMA)
Pan, Zi-Qi& Zhang, Shu-Jun& Wang, Xiang-Lian& Jiao, Yu-Xin& Qiu, Jian-Jian. Machine Learning Based on a Multiparametric and Multiregional Radiomics Signature Predicts Radiotherapeutic Response in Patients with Glioblastoma. Behavioural Neurology. 2020. Vol. 2020, no. 2020, pp.1-12.
https://search.emarefa.net/detail/BIM-1138362
Data Type
Journal Articles
Language
English
Notes
Includes bibliographical references
Record ID
BIM-1138362