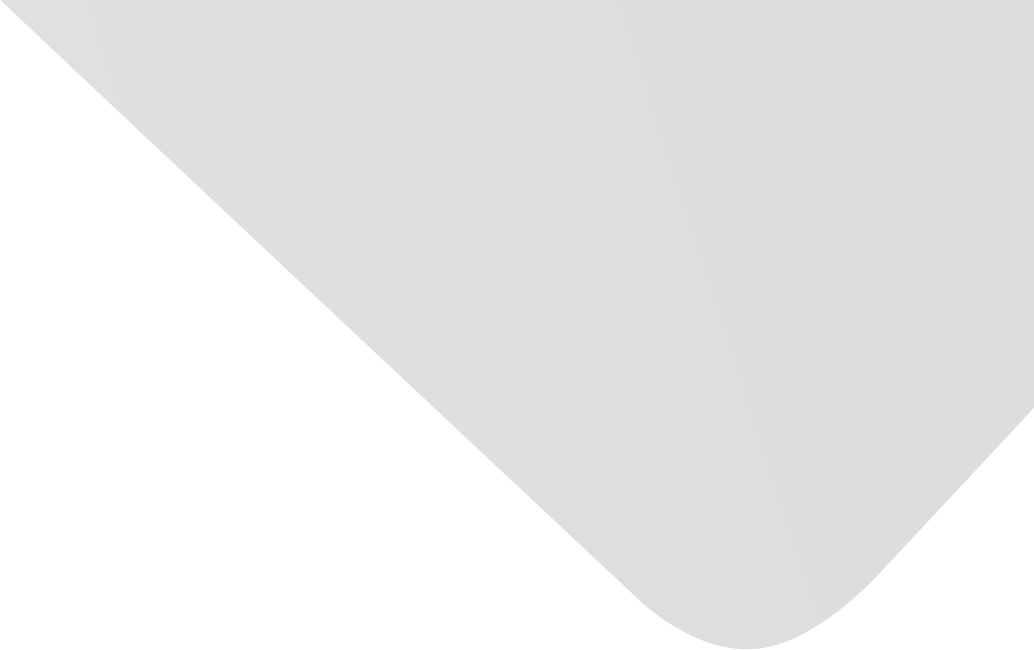
Optimized Mahalanobis–Taguchi System for High-Dimensional Small Sample Data Classification
Joint Authors
Xiao, Xinping
Fu, Dian
Shi, Yu
Wen, Jianghui
Source
Computational Intelligence and Neuroscience
Issue
Vol. 2020, Issue 2020 (31 Dec. 2020), pp.1-15, 15 p.
Publisher
Hindawi Publishing Corporation
Publication Date
2020-04-26
Country of Publication
Egypt
No. of Pages
15
Main Subjects
Abstract EN
The Mahalanobis–Taguchi system (MTS) is a multivariate data diagnosis and prediction technology, which is widely used to optimize large sample data or unbalanced data, but it is rarely used for high-dimensional small sample data.
In this paper, the optimized MTS for the classification of high-dimensional small sample data is discussed from two aspects, namely, the inverse matrix instability of the covariance matrix and the instability of feature selection.
Firstly, based on regularization and smoothing techniques, this paper proposes a modified Mahalanobis metric to calculate the Mahalanobis distance, which is aimed at reducing the influence of the inverse matrix instability under small sample conditions.
Secondly, the minimum redundancy-maximum relevance (mRMR) algorithm is introduced into the MTS for the instability problem of feature selection.
By using the mRMR algorithm and signal-to-noise ratio (SNR), a two-stage feature selection method is proposed: the mRMR algorithm is first used to remove noise and redundant variables; the orthogonal table and SNR are then used to screen the combination of variables that make great contribution to classification.
Then, the feasibility and simplicity of the optimized MTS are shown in five datasets from the UCI database.
The Mahalanobis distance based on regularization and smoothing techniques (RS-MD) is more robust than the traditional Mahalanobis distance.
The two-stage feature selection method improves the effectiveness of feature selection for MTS.
Finally, the optimized MTS is applied to email classification of the Spambase dataset.
The results show that the optimized MTS outperforms the classical MTS and the other 3 machine learning algorithms.
American Psychological Association (APA)
Xiao, Xinping& Fu, Dian& Shi, Yu& Wen, Jianghui. 2020. Optimized Mahalanobis–Taguchi System for High-Dimensional Small Sample Data Classification. Computational Intelligence and Neuroscience،Vol. 2020, no. 2020, pp.1-15.
https://search.emarefa.net/detail/BIM-1138757
Modern Language Association (MLA)
Xiao, Xinping…[et al.]. Optimized Mahalanobis–Taguchi System for High-Dimensional Small Sample Data Classification. Computational Intelligence and Neuroscience No. 2020 (2020), pp.1-15.
https://search.emarefa.net/detail/BIM-1138757
American Medical Association (AMA)
Xiao, Xinping& Fu, Dian& Shi, Yu& Wen, Jianghui. Optimized Mahalanobis–Taguchi System for High-Dimensional Small Sample Data Classification. Computational Intelligence and Neuroscience. 2020. Vol. 2020, no. 2020, pp.1-15.
https://search.emarefa.net/detail/BIM-1138757
Data Type
Journal Articles
Language
English
Notes
Includes bibliographical references
Record ID
BIM-1138757