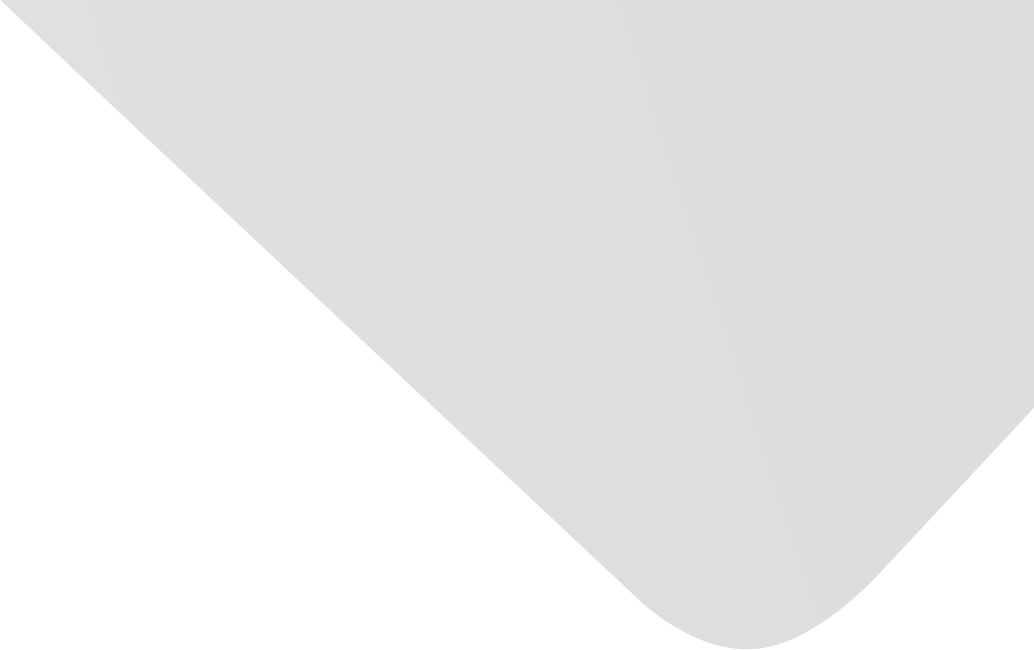
Improved Distance Functions for Instance-Based Text Classification
Joint Authors
Abu Shawar, Bayan
El Hindi, Khalil
Aljulaidan, Reem
Alsalamn, Hussien
Source
Computational Intelligence and Neuroscience
Issue
Vol. 2020, Issue 2020 (31 Dec. 2020), pp.1-10, 10 p.
Publisher
Hindawi Publishing Corporation
Publication Date
2020-11-23
Country of Publication
Egypt
No. of Pages
10
Main Subjects
Abstract EN
Text classification has many applications in text processing and information retrieval.
Instance-based learning (IBL) is among the top-performing text classification methods.
However, its effectiveness depends on the distance function it uses to determine similar documents.
In this study, we evaluate some popular distance measures’ performance and propose new ones that exploit word frequencies and the ordinal relationship between them.
In particular, we propose new distance measures that are based on the value distance metric (VDM) and the inverted specific-class distance measure (ISCDM).
The proposed measures are suitable for documents represented as vectors of word frequencies.
We compare these measures’ performance with their original counterparts and with powerful Naïve Bayesian-based text classification algorithms.
We evaluate the proposed distance measures using the kNN algorithm on 18 benchmark text classification datasets.
Our empirical results reveal that the distance metrics for nominal values render better classification results for text classification than the Euclidean distance measure for numeric values.
Furthermore, our results indicate that ISCDM substantially outperforms VDM, but it is also more susceptible to make use of the ordinal nature of term-frequencies than VDM.
Thus, we were able to propose more ISCDM-based distance measures for text classification than VDM-based measures.
We also compare the proposed distance measures with Naïve Bayesian-based text classification, namely, multinomial Naïve Bayes (MNB), complement Naïve Bayes (CNB), and the one-versus-all-but-one (OVA) model.
It turned out that when kNN uses some of the proposed measures, it outperforms NB-based text classifiers for most datasets.
American Psychological Association (APA)
El Hindi, Khalil& Abu Shawar, Bayan& Aljulaidan, Reem& Alsalamn, Hussien. 2020. Improved Distance Functions for Instance-Based Text Classification. Computational Intelligence and Neuroscience،Vol. 2020, no. 2020, pp.1-10.
https://search.emarefa.net/detail/BIM-1138759
Modern Language Association (MLA)
El Hindi, Khalil…[et al.]. Improved Distance Functions for Instance-Based Text Classification. Computational Intelligence and Neuroscience No. 2020 (2020), pp.1-10.
https://search.emarefa.net/detail/BIM-1138759
American Medical Association (AMA)
El Hindi, Khalil& Abu Shawar, Bayan& Aljulaidan, Reem& Alsalamn, Hussien. Improved Distance Functions for Instance-Based Text Classification. Computational Intelligence and Neuroscience. 2020. Vol. 2020, no. 2020, pp.1-10.
https://search.emarefa.net/detail/BIM-1138759
Data Type
Journal Articles
Language
English
Notes
Includes bibliographical references
Record ID
BIM-1138759