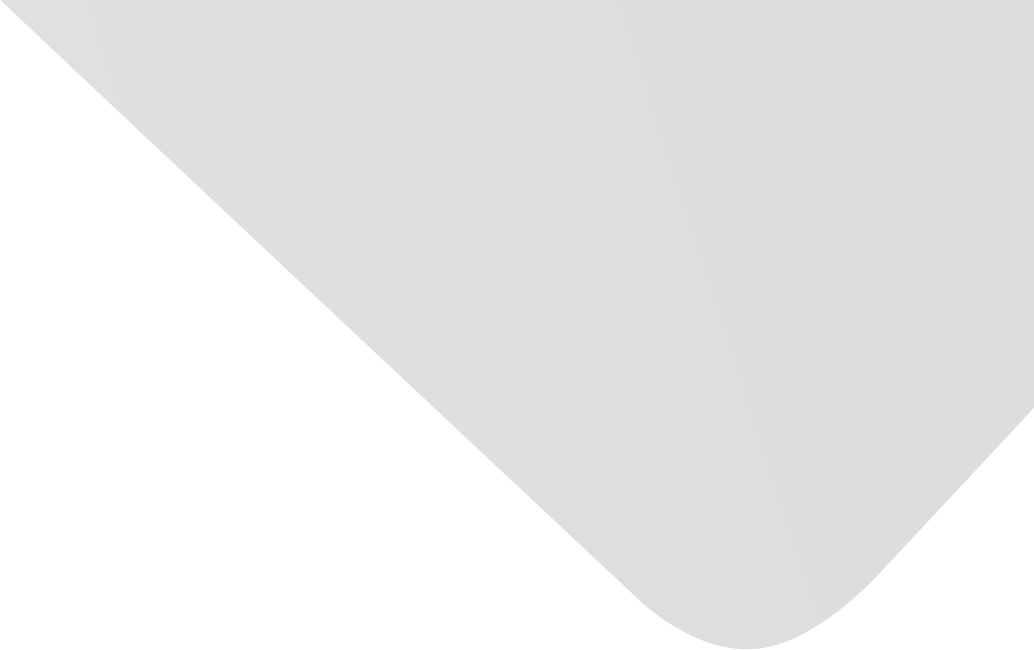
A Hybrid Pathfinder Optimizer for Unconstrained and Constrained Optimization Problems
Joint Authors
Qi, Xiangbo
Yuan, Zhonghu
Song, Yan
Source
Computational Intelligence and Neuroscience
Issue
Vol. 2020, Issue 2020 (31 Dec. 2020), pp.1-25, 25 p.
Publisher
Hindawi Publishing Corporation
Publication Date
2020-05-29
Country of Publication
Egypt
No. of Pages
25
Main Subjects
Abstract EN
Hybridization of metaheuristic algorithms with local search has been investigated in many studies.
This paper proposes a hybrid pathfinder algorithm (HPFA), which incorporates the mutation operator in differential evolution (DE) into the pathfinder algorithm (PFA).
The proposed algorithm combines the searching ability of both PFA and DE.
With a test on a set of twenty-four unconstrained benchmark functions including both unimodal continuous functions, multimodal continuous functions, and composition functions, HPFA is proved to have significant improvement over the pathfinder algorithm and the other comparison algorithms.
Then HPFA is used for data clustering, constrained problems, and engineering design problems.
The experimental results show that the proposed HPFA got better results than the other comparison algorithms and is a competitive approach for solving partitioning clustering, constrained problems, and engineering design problems.
American Psychological Association (APA)
Qi, Xiangbo& Yuan, Zhonghu& Song, Yan. 2020. A Hybrid Pathfinder Optimizer for Unconstrained and Constrained Optimization Problems. Computational Intelligence and Neuroscience،Vol. 2020, no. 2020, pp.1-25.
https://search.emarefa.net/detail/BIM-1138775
Modern Language Association (MLA)
Qi, Xiangbo…[et al.]. A Hybrid Pathfinder Optimizer for Unconstrained and Constrained Optimization Problems. Computational Intelligence and Neuroscience No. 2020 (2020), pp.1-25.
https://search.emarefa.net/detail/BIM-1138775
American Medical Association (AMA)
Qi, Xiangbo& Yuan, Zhonghu& Song, Yan. A Hybrid Pathfinder Optimizer for Unconstrained and Constrained Optimization Problems. Computational Intelligence and Neuroscience. 2020. Vol. 2020, no. 2020, pp.1-25.
https://search.emarefa.net/detail/BIM-1138775
Data Type
Journal Articles
Language
English
Notes
Includes bibliographical references
Record ID
BIM-1138775