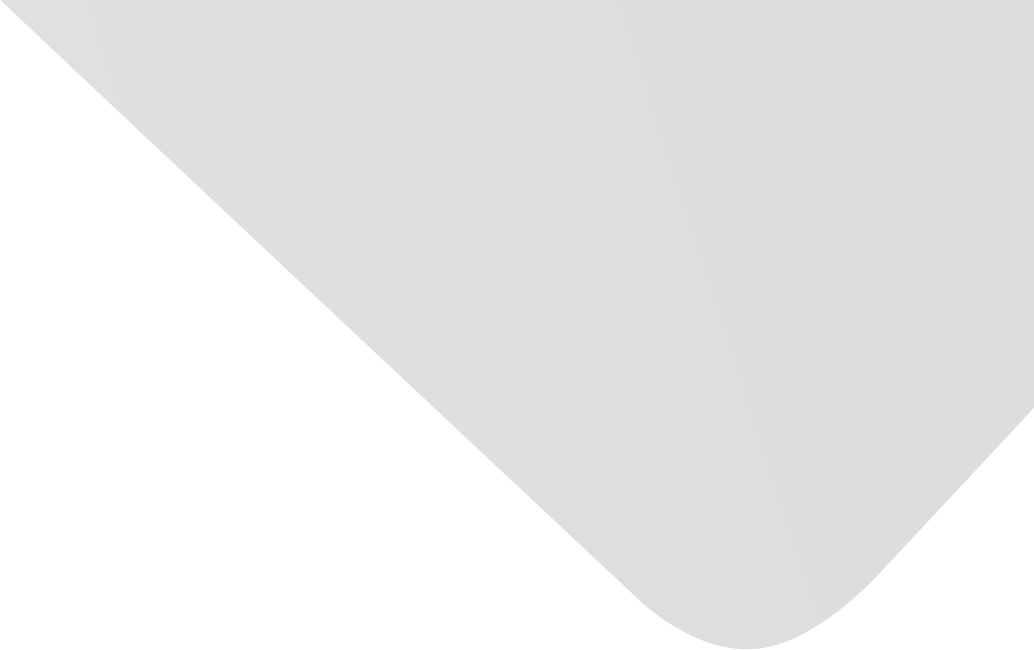
A New Volumetric CNN for 3D Object Classification Based on Joint Multiscale Feature and Subvolume Supervised Learning Approaches
Joint Authors
Hou, Li
Muzahid, A. A. M.
Wan, Wanggen
Source
Computational Intelligence and Neuroscience
Issue
Vol. 2020, Issue 2020 (31 Dec. 2020), pp.1-17, 17 p.
Publisher
Hindawi Publishing Corporation
Publication Date
2020-05-28
Country of Publication
Egypt
No. of Pages
17
Main Subjects
Abstract EN
The advancement of low-cost RGB-D and LiDAR three-dimensional (3D) sensors has permitted the obtainment of the 3D model easier in real-time.
However, making intricate 3D features is crucial for the advancement of 3D object classifications.
The existing volumetric voxel-based CNN approaches have achieved remarkable progress, but they generate huge computational overhead that limits the extraction of global features at higher resolutions of 3D objects.
In this paper, a low-cost 3D volumetric deep convolutional neural network is proposed for 3D object classification based on joint multiscale hierarchical and subvolume supervised learning strategies.
Our proposed deep neural network inputs 3D data, which are preprocessed by implementing memory-efficient octree representation, and we propose to limit the full layer octree depth to a certain level based on the predefined input volume resolution for storing high-precision contour features.
Multiscale features are concatenated from multilevel octree depths inside the network, aiming to adaptively generate high-level global features.
The strategy of the subvolume supervision approach is to train the network on subparts of the 3D object in order to learn local features.
Our framework has been evaluated with two publicly available 3D repositories.
Experimental results demonstrate the effectiveness of our proposed method where the classification accuracy is improved in comparison to existing volumetric approaches, and the memory consumption ratio and run-time are significantly reduced.
American Psychological Association (APA)
Muzahid, A. A. M.& Wan, Wanggen& Hou, Li. 2020. A New Volumetric CNN for 3D Object Classification Based on Joint Multiscale Feature and Subvolume Supervised Learning Approaches. Computational Intelligence and Neuroscience،Vol. 2020, no. 2020, pp.1-17.
https://search.emarefa.net/detail/BIM-1138777
Modern Language Association (MLA)
Muzahid, A. A. M.…[et al.]. A New Volumetric CNN for 3D Object Classification Based on Joint Multiscale Feature and Subvolume Supervised Learning Approaches. Computational Intelligence and Neuroscience No. 2020 (2020), pp.1-17.
https://search.emarefa.net/detail/BIM-1138777
American Medical Association (AMA)
Muzahid, A. A. M.& Wan, Wanggen& Hou, Li. A New Volumetric CNN for 3D Object Classification Based on Joint Multiscale Feature and Subvolume Supervised Learning Approaches. Computational Intelligence and Neuroscience. 2020. Vol. 2020, no. 2020, pp.1-17.
https://search.emarefa.net/detail/BIM-1138777
Data Type
Journal Articles
Language
English
Notes
Includes bibliographical references
Record ID
BIM-1138777