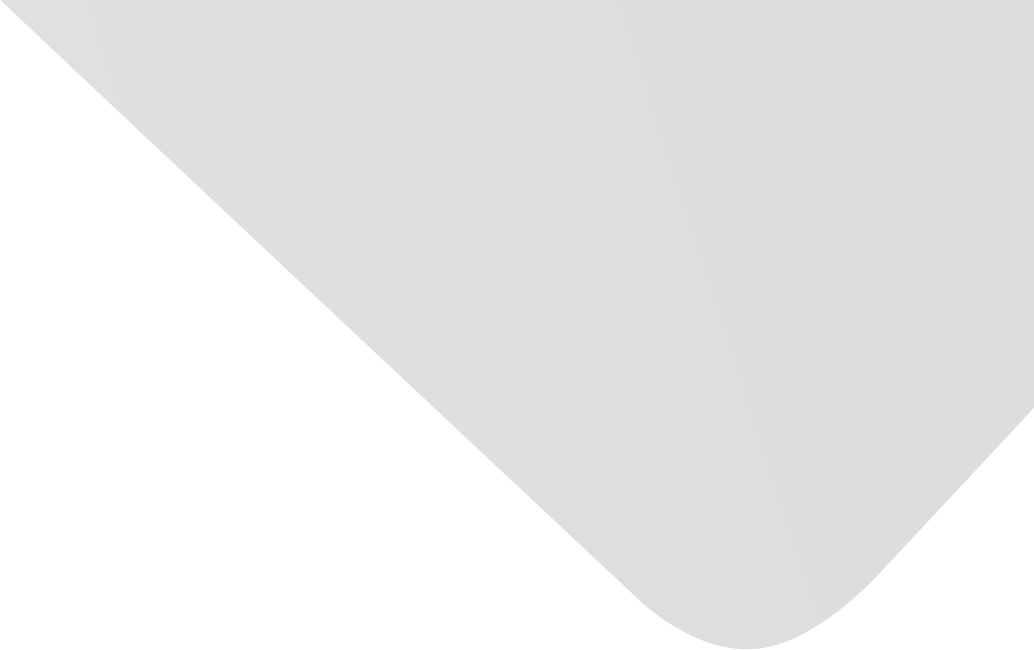
A Knowledge-Fusion Ranking System with an Attention Network for Making Assignment Recommendations
Joint Authors
Wang, Weisong
Zhang, Chi
Jin, Canghong
Zhou, Yuli
Ying, Shengyu
Wu, Minghui
Source
Computational Intelligence and Neuroscience
Issue
Vol. 2020, Issue 2020 (31 Dec. 2020), pp.1-11, 11 p.
Publisher
Hindawi Publishing Corporation
Publication Date
2020-12-23
Country of Publication
Egypt
No. of Pages
11
Main Subjects
Abstract EN
In recent decades, more teachers are using question generators to provide students with online homework.
Learning-to-rank (LTR) methods can partially rank questions to address the needs of individual students and reduce their study burden.
Unfortunately, ranking questions for students is not trivial because of three main challenges: (1) discovering students’ latent knowledge and cognitive level is difficult, (2) the content of quizzes can be totally different but the knowledge points of these quizzes may be inherently related, and (3) ranking models based on supervised, semisupervised, or reinforcement learning focus on the current assignment without considering past performance.
In this work, we propose KFRank, a knowledge-fusion ranking model based on reinforcement learning, which considers both a student’s assignment history and the relevance of quizzes with their knowledge points.
First, we load students’ assignment history, reorganize it using knowledge points, and calculate the effective features for ranking in terms of the relation between a student’s knowledge cognitive and the question.
Then, a similarity estimator is built to choose historical questions, and an attention neural network is used to calculate the attention value and update the current study state with knowledge fusion.
Finally, a rank algorithm based on a Markov decision process is used to optimize the parameters.
Extensive experiments were conducted on a real-life dataset spanning a year and we compared our model with the state-of-the-art ranking models (e.g., ListNET and LambdaMART) and reinforcement-learning methods (such as MDPRank).
Based on top-k nDCG values, our model outperforms other methods for groups of average and weak students, whose study abilities are relatively poor and thus their behaviors are more difficult to predict.
American Psychological Association (APA)
Jin, Canghong& Zhou, Yuli& Ying, Shengyu& Zhang, Chi& Wang, Weisong& Wu, Minghui. 2020. A Knowledge-Fusion Ranking System with an Attention Network for Making Assignment Recommendations. Computational Intelligence and Neuroscience،Vol. 2020, no. 2020, pp.1-11.
https://search.emarefa.net/detail/BIM-1138797
Modern Language Association (MLA)
Jin, Canghong…[et al.]. A Knowledge-Fusion Ranking System with an Attention Network for Making Assignment Recommendations. Computational Intelligence and Neuroscience No. 2020 (2020), pp.1-11.
https://search.emarefa.net/detail/BIM-1138797
American Medical Association (AMA)
Jin, Canghong& Zhou, Yuli& Ying, Shengyu& Zhang, Chi& Wang, Weisong& Wu, Minghui. A Knowledge-Fusion Ranking System with an Attention Network for Making Assignment Recommendations. Computational Intelligence and Neuroscience. 2020. Vol. 2020, no. 2020, pp.1-11.
https://search.emarefa.net/detail/BIM-1138797
Data Type
Journal Articles
Language
English
Notes
Includes bibliographical references
Record ID
BIM-1138797