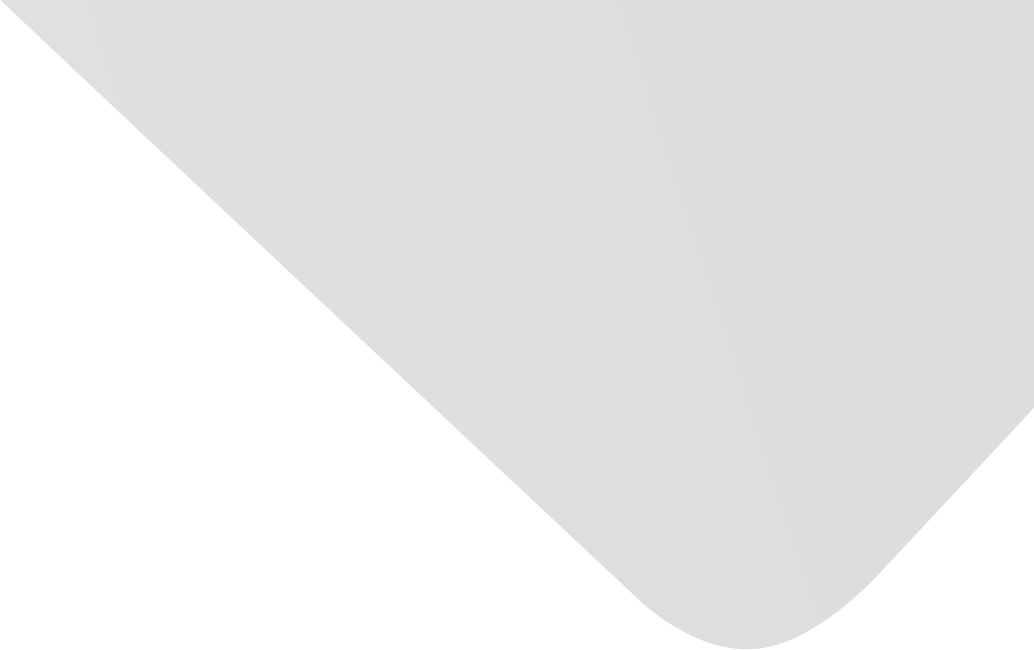
Dynamic Multi-Swarm Differential Learning Quantum Bird Swarm Algorithm and Its Application in Random Forest Classification Model
Joint Authors
Zhang, Jiangnan
Fan, Shurui
Xia, Kewen
He, Ziping
Source
Computational Intelligence and Neuroscience
Issue
Vol. 2020, Issue 2020 (31 Dec. 2020), pp.1-24, 24 p.
Publisher
Hindawi Publishing Corporation
Publication Date
2020-08-07
Country of Publication
Egypt
No. of Pages
24
Main Subjects
Abstract EN
Bird swarm algorithm is one of the swarm intelligence algorithms proposed recently.
However, the original bird swarm algorithm has some drawbacks, such as easy to fall into local optimum and slow convergence speed.
To overcome these short-comings, a dynamic multi-swarm differential learning quantum bird swarm algorithm which combines three hybrid strategies was established.
First, establishing a dynamic multi-swarm bird swarm algorithm and the differential evolution strategy was adopted to enhance the randomness of the foraging behavior’s movement, which can make the bird swarm algorithm have a stronger global exploration capability.
Next, quantum behavior was introduced into the bird swarm algorithm for more efficient search solution space.
Then, the improved bird swarm algorithm is used to optimize the number of decision trees and the number of predictor variables on the random forest classification model.
In the experiment, the 18 benchmark functions, 30 CEC2014 functions, and the 8 UCI datasets are tested to show that the improved algorithm and model are very competitive and outperform the other algorithms and models.
Finally, the effective random forest classification model was applied to actual oil logging prediction.
As the experimental results show, the three strategies can significantly boost the performance of the bird swarm algorithm and the proposed learning scheme can guarantee a more stable random forest classification model with higher accuracy and efficiency compared to others.
American Psychological Association (APA)
Zhang, Jiangnan& Xia, Kewen& He, Ziping& Fan, Shurui. 2020. Dynamic Multi-Swarm Differential Learning Quantum Bird Swarm Algorithm and Its Application in Random Forest Classification Model. Computational Intelligence and Neuroscience،Vol. 2020, no. 2020, pp.1-24.
https://search.emarefa.net/detail/BIM-1138798
Modern Language Association (MLA)
Zhang, Jiangnan…[et al.]. Dynamic Multi-Swarm Differential Learning Quantum Bird Swarm Algorithm and Its Application in Random Forest Classification Model. Computational Intelligence and Neuroscience No. 2020 (2020), pp.1-24.
https://search.emarefa.net/detail/BIM-1138798
American Medical Association (AMA)
Zhang, Jiangnan& Xia, Kewen& He, Ziping& Fan, Shurui. Dynamic Multi-Swarm Differential Learning Quantum Bird Swarm Algorithm and Its Application in Random Forest Classification Model. Computational Intelligence and Neuroscience. 2020. Vol. 2020, no. 2020, pp.1-24.
https://search.emarefa.net/detail/BIM-1138798
Data Type
Journal Articles
Language
English
Notes
Includes bibliographical references
Record ID
BIM-1138798