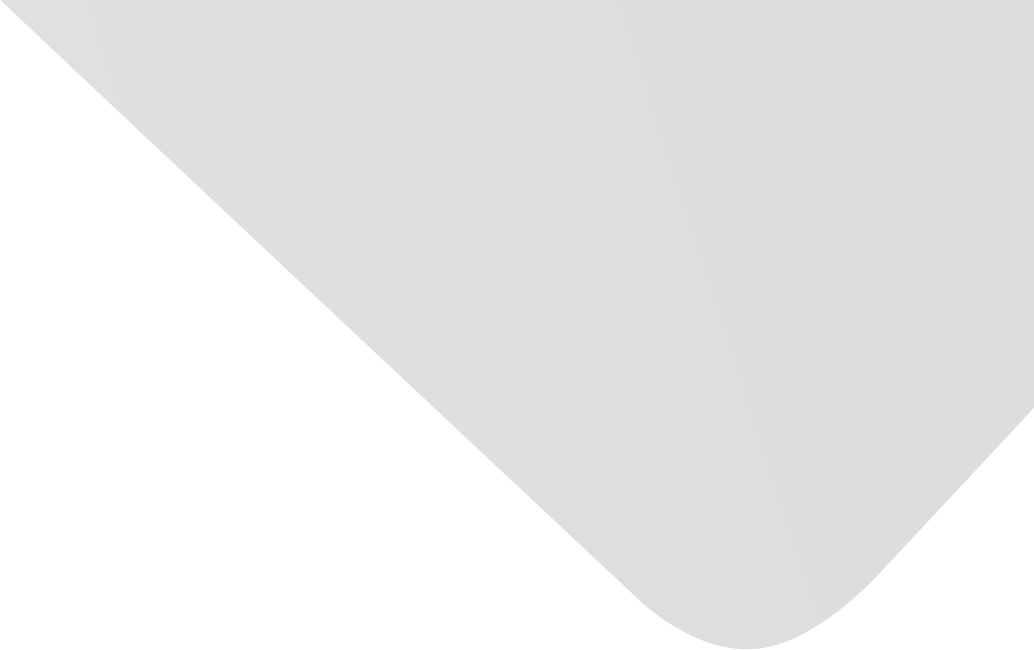
Anomaly Detection in EEG Signals: A Case Study on Similarity Measure
Joint Authors
Chen, Guangyuan
Lu, Guoliang
Xie, Zhaohong
Shang, Wei
Source
Computational Intelligence and Neuroscience
Issue
Vol. 2020, Issue 2020 (31 Dec. 2020), pp.1-16, 16 p.
Publisher
Hindawi Publishing Corporation
Publication Date
2020-01-10
Country of Publication
Egypt
No. of Pages
16
Main Subjects
Abstract EN
Motivation.
Anomaly EEG detection is a long-standing problem in analysis of EEG signals.
The basic premise of this problem is consideration of the similarity between two nonstationary EEG recordings.
A well-established scheme is based on sequence matching, typically including three steps: feature extraction, similarity measure, and decision-making.
Current approaches mainly focus on EEG feature extraction and decision-making, and few of them involve the similarity measure/quantification.
Generally, to design an appropriate similarity metric, that is compatible with the considered problem/data, is also an important issue in the design of such detection systems.
It is however impossible to directly apply those existing metrics to anomaly EEG detection without any consideration of domain specificity.
Methodology.
The main objective of this work is to investigate the impacts of different similarity metrics on anomaly EEG detection.
A few metrics that are potentially available for the EEG analysis have been collected from other areas by a careful review of related works.
The so-called power spectrum is extracted as features of EEG signals, and a null hypothesis testing is employed to make the final decision.
Two indicators have been used to evaluate the detection performance.
One is to reflect the level of measured similarity between two compared EEG signals, and the other is to quantify the detection accuracy.
Results.
Experiments were conducted on two data sets, respectively.
The results demonstrate the positive impacts of different similarity metrics on anomaly EEG detection.
The Hellinger distance (HD) and Bhattacharyya distance (BD) metrics show excellent performances: an accuracy of 0.9167 for our data set and an accuracy of 0.9667 for the Bern-Barcelona EEG data set.
Both of HD and BD metrics are constructed based on the Bhattacharyya coefficient, implying the priority of the Bhattacharyya coefficient when dealing with the highly noisy EEG signals.
In future work, we will exploit an integrated metric that combines HD and BD for the similarity measure of EEG signals.
American Psychological Association (APA)
Chen, Guangyuan& Lu, Guoliang& Xie, Zhaohong& Shang, Wei. 2020. Anomaly Detection in EEG Signals: A Case Study on Similarity Measure. Computational Intelligence and Neuroscience،Vol. 2020, no. 2020, pp.1-16.
https://search.emarefa.net/detail/BIM-1138799
Modern Language Association (MLA)
Chen, Guangyuan…[et al.]. Anomaly Detection in EEG Signals: A Case Study on Similarity Measure. Computational Intelligence and Neuroscience No. 2020 (2020), pp.1-16.
https://search.emarefa.net/detail/BIM-1138799
American Medical Association (AMA)
Chen, Guangyuan& Lu, Guoliang& Xie, Zhaohong& Shang, Wei. Anomaly Detection in EEG Signals: A Case Study on Similarity Measure. Computational Intelligence and Neuroscience. 2020. Vol. 2020, no. 2020, pp.1-16.
https://search.emarefa.net/detail/BIM-1138799
Data Type
Journal Articles
Language
English
Notes
Includes bibliographical references
Record ID
BIM-1138799