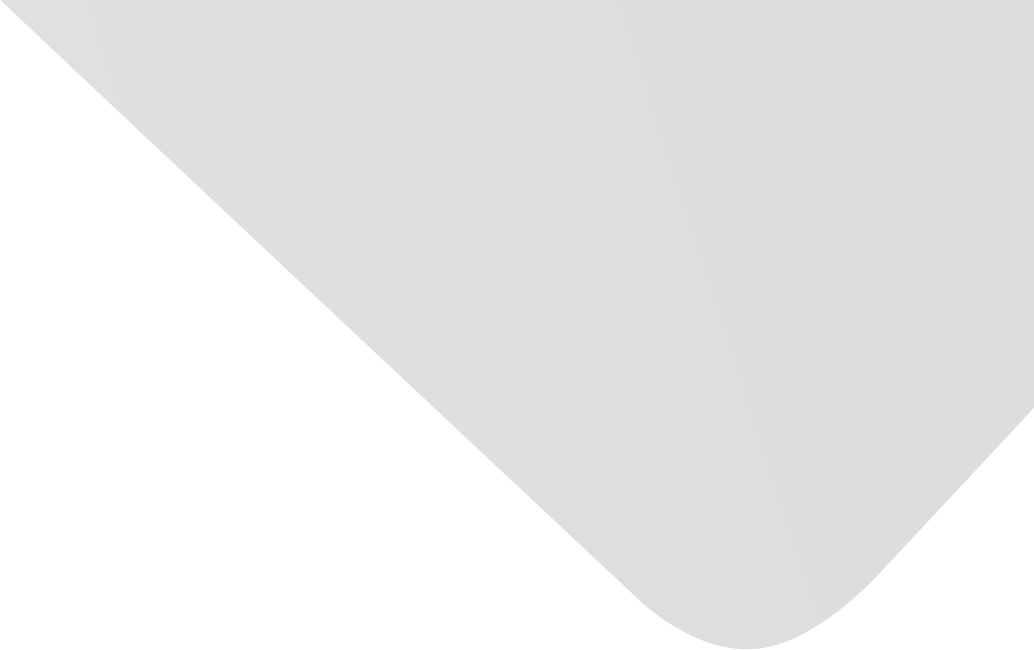
A Novel Low-Bit Quantization Strategy for Compressing Deep Neural Networks
Joint Authors
Zeng, Xiangrong
Long, Xin
Ben, Zongcheng
Zhou, Dianle
Zhang, Maojun
Source
Computational Intelligence and Neuroscience
Issue
Vol. 2020, Issue 2020 (31 Dec. 2020), pp.1-7, 7 p.
Publisher
Hindawi Publishing Corporation
Publication Date
2020-02-18
Country of Publication
Egypt
No. of Pages
7
Main Subjects
Abstract EN
The increase in sophistication of neural network models in recent years has exponentially expanded memory consumption and computational cost, thereby hindering their applications on ASIC, FPGA, and other mobile devices.
Therefore, compressing and accelerating the neural networks are necessary.
In this study, we introduce a novel strategy to train low-bit networks with weights and activations quantized by several bits and address two corresponding fundamental issues.
One is to approximate activations through low-bit discretization for decreasing network computational cost and dot-product memory.
The other is to specify weight quantization and update mechanism for discrete weights to avoid gradient mismatch.
With quantized low-bit weights and activations, the costly full-precision operation will be replaced by shift operation.
We evaluate the proposed method on common datasets, and results show that this method can dramatically compress the neural network with slight accuracy loss.
American Psychological Association (APA)
Long, Xin& Zeng, Xiangrong& Ben, Zongcheng& Zhou, Dianle& Zhang, Maojun. 2020. A Novel Low-Bit Quantization Strategy for Compressing Deep Neural Networks. Computational Intelligence and Neuroscience،Vol. 2020, no. 2020, pp.1-7.
https://search.emarefa.net/detail/BIM-1138825
Modern Language Association (MLA)
Long, Xin…[et al.]. A Novel Low-Bit Quantization Strategy for Compressing Deep Neural Networks. Computational Intelligence and Neuroscience No. 2020 (2020), pp.1-7.
https://search.emarefa.net/detail/BIM-1138825
American Medical Association (AMA)
Long, Xin& Zeng, Xiangrong& Ben, Zongcheng& Zhou, Dianle& Zhang, Maojun. A Novel Low-Bit Quantization Strategy for Compressing Deep Neural Networks. Computational Intelligence and Neuroscience. 2020. Vol. 2020, no. 2020, pp.1-7.
https://search.emarefa.net/detail/BIM-1138825
Data Type
Journal Articles
Language
English
Notes
Includes bibliographical references
Record ID
BIM-1138825