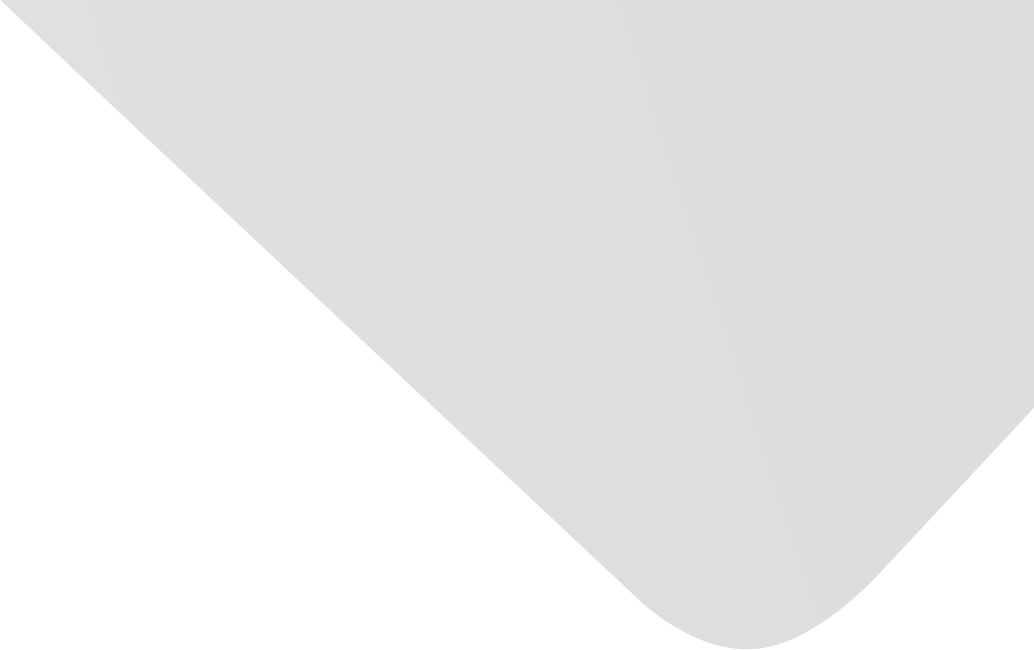
A Search History-Driven Offspring Generation Method for the Real-Coded Genetic Algorithm
Joint Authors
Nakane, Takumi
Zhang, Chao
Lu, Xuequan
Source
Computational Intelligence and Neuroscience
Issue
Vol. 2020, Issue 2020 (31 Dec. 2020), pp.1-20, 20 p.
Publisher
Hindawi Publishing Corporation
Publication Date
2020-09-27
Country of Publication
Egypt
No. of Pages
20
Main Subjects
Abstract EN
In evolutionary algorithms, genetic operators iteratively generate new offspring which constitute a potentially valuable set of search history.
To boost the performance of offspring generation in the real-coded genetic algorithm (RCGA), in this paper, we propose to exploit the search history cached so far in an online style during the iteration.
Specifically, survivor individuals over the past few generations are collected and stored in the archive to form the search history.
We introduce a simple yet effective crossover model driven by the search history (abbreviated as SHX).
In particular, the search history is clustered, and each cluster is assigned a score for SHX.
In essence, the proposed SHX is a data-driven method which exploits the search history to perform offspring selection after the offspring generation.
Since no additional fitness evaluations are needed, SHX is favorable for the tasks with limited budget or expensive fitness evaluations.
We experimentally verify the effectiveness of SHX over 15 benchmark functions.
Quantitative results show that our SHX can significantly enhance the performance of RCGA, in terms of both accuracy and convergence speed.
Also, the induced additional runtime is negligible compared to the total processing time.
American Psychological Association (APA)
Nakane, Takumi& Lu, Xuequan& Zhang, Chao. 2020. A Search History-Driven Offspring Generation Method for the Real-Coded Genetic Algorithm. Computational Intelligence and Neuroscience،Vol. 2020, no. 2020, pp.1-20.
https://search.emarefa.net/detail/BIM-1138877
Modern Language Association (MLA)
Nakane, Takumi…[et al.]. A Search History-Driven Offspring Generation Method for the Real-Coded Genetic Algorithm. Computational Intelligence and Neuroscience No. 2020 (2020), pp.1-20.
https://search.emarefa.net/detail/BIM-1138877
American Medical Association (AMA)
Nakane, Takumi& Lu, Xuequan& Zhang, Chao. A Search History-Driven Offspring Generation Method for the Real-Coded Genetic Algorithm. Computational Intelligence and Neuroscience. 2020. Vol. 2020, no. 2020, pp.1-20.
https://search.emarefa.net/detail/BIM-1138877
Data Type
Journal Articles
Language
English
Notes
Includes bibliographical references
Record ID
BIM-1138877