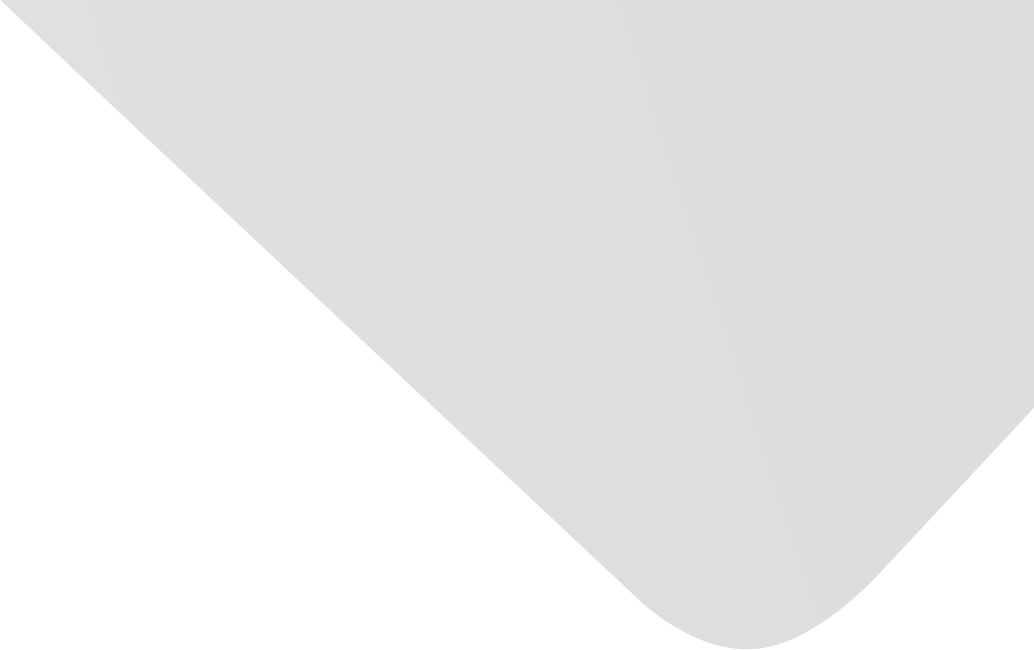
Schizophrenia EEG Signal Classification Based on Swarm Intelligence Computing
Joint Authors
Kim, Sun-Hee
Prabhakar, Sunil Kumar
Rajaguru, Harikumar
Source
Computational Intelligence and Neuroscience
Issue
Vol. 2020, Issue 2020 (31 Dec. 2020), pp.1-14, 14 p.
Publisher
Hindawi Publishing Corporation
Publication Date
2020-11-30
Country of Publication
Egypt
No. of Pages
14
Main Subjects
Abstract EN
One of the serious mental disorders where people interpret reality in an abnormal state is schizophrenia.
A combination of extremely disordered thinking, delusion, and hallucination is caused due to schizophrenia, and the daily functions of a person are severely disturbed because of this disorder.
A wide range of problems are caused due to schizophrenia such as disturbed thinking and behaviour.
In the field of human neuroscience, the analysis of brain activity is quite an important research area.
For general cognitive activity analysis, electroencephalography (EEG) signals are widely used as a low-resolution diagnosis tool.
The EEG signals are a great boon to understand the abnormality of the brain disorders, especially schizophrenia.
In this work, schizophrenia EEG signal classification is performed wherein, initially, features such as Detrend Fluctuation Analysis (DFA), Hurst Exponent, Recurrence Quantification Analysis (RQA), Sample Entropy, Fractal Dimension (FD), Kolmogorov Complexity, Hjorth exponent, Lempel Ziv Complexity (LZC), and Largest Lyapunov Exponent (LLE) are extracted initially.
The extracted features are, then, optimized for selecting the best features through four types of optimization algorithms here such as Artificial Flora (AF) optimization, Glowworm Search (GS) optimization, Black Hole (BH) optimization, and Monkey Search (MS) optimization, and finally, it is classified through certain classifiers.
The best results show that, for normal cases, a classification accuracy of 87.54% is obtained when BH optimization is utilized with Support Vector Machine-Radial Basis Function (SVM-RBF) kernel, and for schizophrenia cases, a classification accuracy of 92.17% is obtained when BH optimization is utilized with SVM-RBF kernel.
American Psychological Association (APA)
Prabhakar, Sunil Kumar& Rajaguru, Harikumar& Kim, Sun-Hee. 2020. Schizophrenia EEG Signal Classification Based on Swarm Intelligence Computing. Computational Intelligence and Neuroscience،Vol. 2020, no. 2020, pp.1-14.
https://search.emarefa.net/detail/BIM-1138906
Modern Language Association (MLA)
Prabhakar, Sunil Kumar…[et al.]. Schizophrenia EEG Signal Classification Based on Swarm Intelligence Computing. Computational Intelligence and Neuroscience No. 2020 (2020), pp.1-14.
https://search.emarefa.net/detail/BIM-1138906
American Medical Association (AMA)
Prabhakar, Sunil Kumar& Rajaguru, Harikumar& Kim, Sun-Hee. Schizophrenia EEG Signal Classification Based on Swarm Intelligence Computing. Computational Intelligence and Neuroscience. 2020. Vol. 2020, no. 2020, pp.1-14.
https://search.emarefa.net/detail/BIM-1138906
Data Type
Journal Articles
Language
English
Notes
Includes bibliographical references
Record ID
BIM-1138906