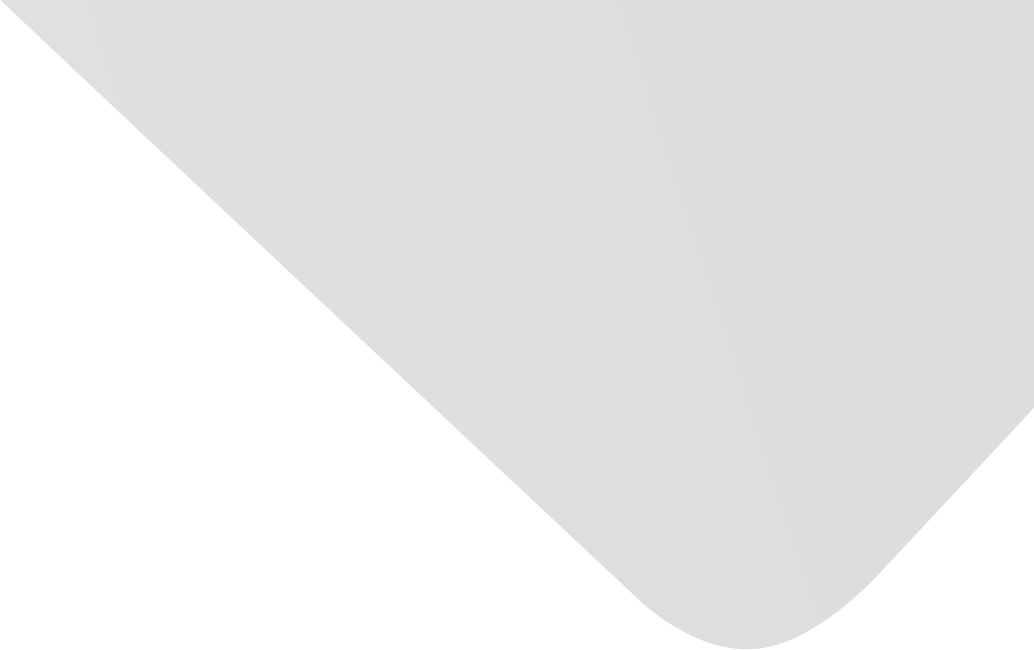
Continuous Similarity Learning with Shared Neural Semantic Representation for Joint Event Detection and Evolution
Joint Authors
Cao, Zhen
Zhou, Pengpeng
Luo, Yao
Ning, Nianwen
Jia, Bingjing
Wu, Bin
Source
Computational Intelligence and Neuroscience
Issue
Vol. 2020, Issue 2020 (31 Dec. 2020), pp.1-13, 13 p.
Publisher
Hindawi Publishing Corporation
Publication Date
2020-12-14
Country of Publication
Egypt
No. of Pages
13
Main Subjects
Abstract EN
In the era of the rapid development of today’s Internet, people often feel overwhelmed by vast official news streams or unofficial self-media tweets.
To help people obtain the news topics they care about, there is a growing need for systems that can extract important events from this amount of data and construct the evolution procedure of events logically into a story.
Most existing methods treat event detection and evolution as two independent subtasks under an integrated pipeline setting.
However, the interdependence between these two subtasks is often ignored, which leads to a biased propagation.
Besides, due to the limitations of news documents’ semantic representation, the performance of event detection and evolution is still limited.
To tackle these problems, we propose a Joint Event Detection and Evolution (JEDE) model, to detect events and discover the event evolution relationships from news streams in this paper.
Specifically, the proposed JEDE model is built upon the Siamese network, which first introduces the bidirectional GRU attention network to learn the vector-based semantic representation for news documents shared across two subtask networks.
Then, two continuous similarity metrics are learned using stacked neural networks to judge whether two news documents are related to the same event or two events are related to the same story.
Furthermore, due to the limited available dataset with ground truths, we make efforts to construct a new dataset, named EDENS, which contains valid labels of events and stories.
The experimental results on this newly created dataset demonstrate that, thanks to the shared representation and joint training, the proposed model consistently achieves significant improvements over the baseline methods.
American Psychological Association (APA)
Zhou, Pengpeng& Luo, Yao& Ning, Nianwen& Cao, Zhen& Jia, Bingjing& Wu, Bin. 2020. Continuous Similarity Learning with Shared Neural Semantic Representation for Joint Event Detection and Evolution. Computational Intelligence and Neuroscience،Vol. 2020, no. 2020, pp.1-13.
https://search.emarefa.net/detail/BIM-1138917
Modern Language Association (MLA)
Zhou, Pengpeng…[et al.]. Continuous Similarity Learning with Shared Neural Semantic Representation for Joint Event Detection and Evolution. Computational Intelligence and Neuroscience No. 2020 (2020), pp.1-13.
https://search.emarefa.net/detail/BIM-1138917
American Medical Association (AMA)
Zhou, Pengpeng& Luo, Yao& Ning, Nianwen& Cao, Zhen& Jia, Bingjing& Wu, Bin. Continuous Similarity Learning with Shared Neural Semantic Representation for Joint Event Detection and Evolution. Computational Intelligence and Neuroscience. 2020. Vol. 2020, no. 2020, pp.1-13.
https://search.emarefa.net/detail/BIM-1138917
Data Type
Journal Articles
Language
English
Notes
Includes bibliographical references
Record ID
BIM-1138917