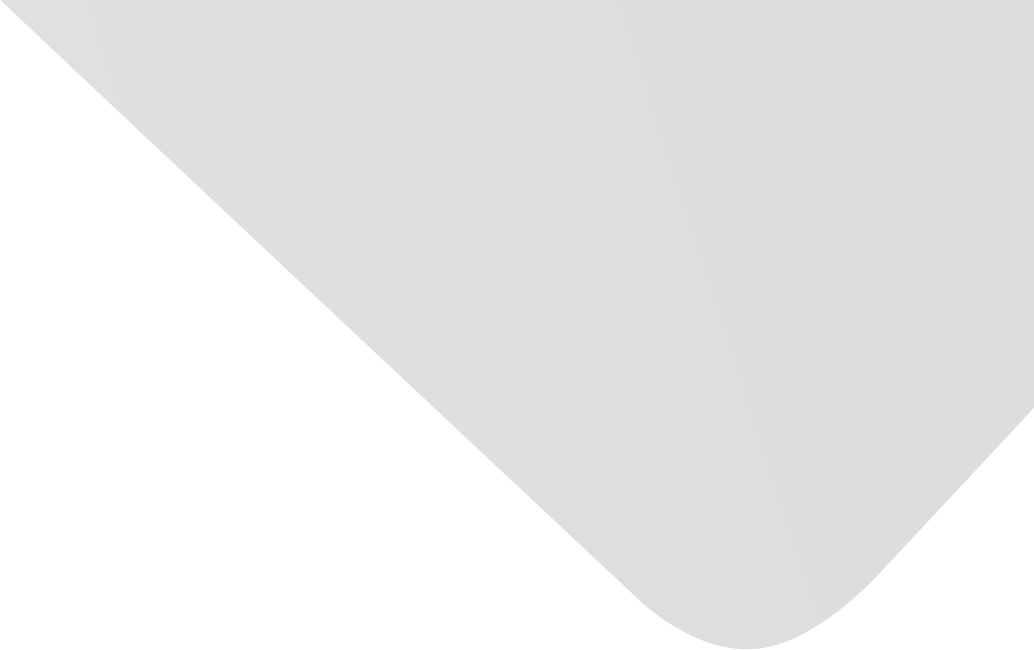
A Compressive Sensing Model for Speeding Up Text Classification
Joint Authors
Source
Computational Intelligence and Neuroscience
Issue
Vol. 2020, Issue 2020 (31 Dec. 2020), pp.1-11, 11 p.
Publisher
Hindawi Publishing Corporation
Publication Date
2020-08-07
Country of Publication
Egypt
No. of Pages
11
Main Subjects
Abstract EN
Text classification plays an important role in various applications of big data by automatically classifying massive text documents.
However, high dimensionality and sparsity of text features have presented a challenge to efficient classification.
In this paper, we propose a compressive sensing- (CS-) based model to speed up text classification.
Using CS to reduce the size of feature space, our model has a low time and space complexity while training a text classifier, and the restricted isometry property (RIP) of CS ensures that pairwise distances between text features can be well preserved in the process of dimensionality reduction.
In particular, by structural random matrices (SRMs), CS is free from computation and memory limitations in the construction of random projections.
Experimental results demonstrate that CS effectively accelerates the text classification while hardly causing any accuracy loss.
American Psychological Association (APA)
Shen, Kelin& Hao, Peinan& Li, Ran. 2020. A Compressive Sensing Model for Speeding Up Text Classification. Computational Intelligence and Neuroscience،Vol. 2020, no. 2020, pp.1-11.
https://search.emarefa.net/detail/BIM-1138943
Modern Language Association (MLA)
Shen, Kelin…[et al.]. A Compressive Sensing Model for Speeding Up Text Classification. Computational Intelligence and Neuroscience No. 2020 (2020), pp.1-11.
https://search.emarefa.net/detail/BIM-1138943
American Medical Association (AMA)
Shen, Kelin& Hao, Peinan& Li, Ran. A Compressive Sensing Model for Speeding Up Text Classification. Computational Intelligence and Neuroscience. 2020. Vol. 2020, no. 2020, pp.1-11.
https://search.emarefa.net/detail/BIM-1138943
Data Type
Journal Articles
Language
English
Notes
Includes bibliographical references
Record ID
BIM-1138943