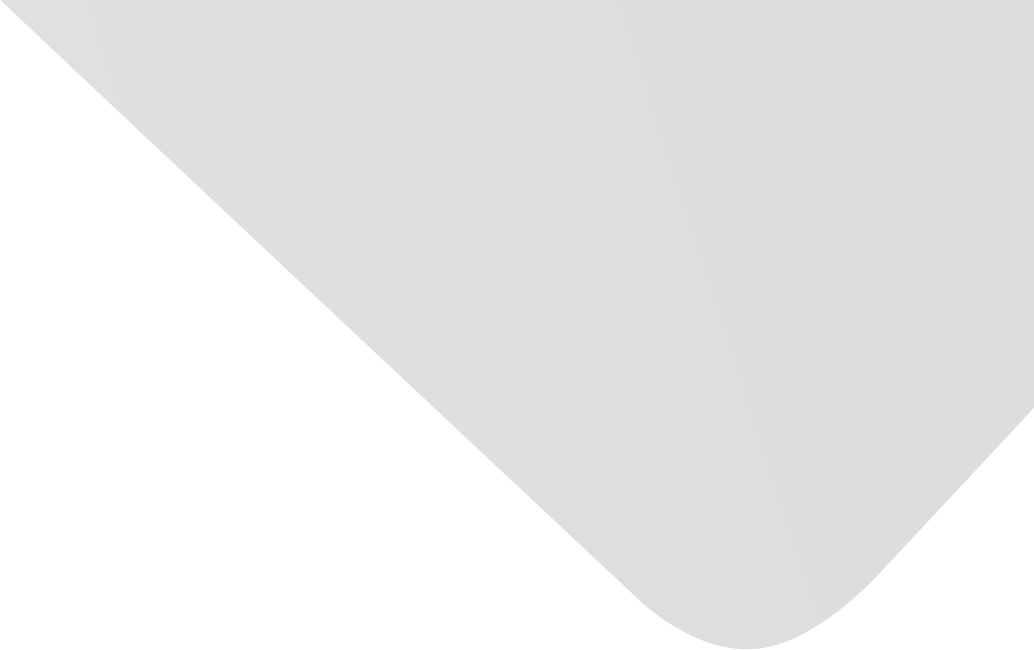
Instance Transfer Subject-Dependent Strategy for Motor Imagery Signal Classification Using Deep Convolutional Neural Networks
Joint Authors
Xu, Guanghua
Chen, Longting
Zhang, Kai
Tian, Peiyuan
Han, ChengCheng
Zhang, Sicong
Duan, Nan
Source
Computational and Mathematical Methods in Medicine
Issue
Vol. 2020, Issue 2020 (31 Dec. 2020), pp.1-10, 10 p.
Publisher
Hindawi Publishing Corporation
Publication Date
2020-08-28
Country of Publication
Egypt
No. of Pages
10
Main Subjects
Abstract EN
In the process of brain-computer interface (BCI), variations across sessions/subjects result in differences in the properties of potential of the brain.
This issue may lead to variations in feature distribution of electroencephalogram (EEG) across subjects, which greatly reduces the generalization ability of a classifier.
Although subject-dependent (SD) strategy provides a promising way to solve the problem of personalized classification, it cannot achieve expected performance due to the limitation of the amount of data especially for a deep neural network (DNN) classification model.
Herein, we propose an instance transfer subject-independent (ITSD) framework combined with a convolutional neural network (CNN) to improve the classification accuracy of the model during motor imagery (MI) task.
The proposed framework consists of the following steps.
Firstly, an instance transfer learning based on the perceptive Hash algorithm is proposed to measure similarity of spectrogram EEG signals between different subjects.
Then, we develop a CNN to decode these signals after instance transfer learning.
Next, the performance of classifications by different training strategies (subject-independent- (SI-) CNN, SD-CNN, and ITSD-CNN) are compared.
To verify the effectiveness of the algorithm, we evaluate it on the dataset of BCI competition IV-2b.
Experiments show that the instance transfer learning can achieve positive instance transfer using a CNN classification model.
Among the three different training strategies, the average classification accuracy of ITSD-CNN can achieve 94.7±2.6 and obtain obvious improvement compared with a contrast model p<0.01.
Compared with other methods proposed in previous research, the framework of ITSD-CNN outperforms the state-of-the-art classification methods with a mean kappa value of 0.664.
American Psychological Association (APA)
Zhang, Kai& Xu, Guanghua& Chen, Longting& Tian, Peiyuan& Han, ChengCheng& Zhang, Sicong…[et al.]. 2020. Instance Transfer Subject-Dependent Strategy for Motor Imagery Signal Classification Using Deep Convolutional Neural Networks. Computational and Mathematical Methods in Medicine،Vol. 2020, no. 2020, pp.1-10.
https://search.emarefa.net/detail/BIM-1139345
Modern Language Association (MLA)
Zhang, Kai…[et al.]. Instance Transfer Subject-Dependent Strategy for Motor Imagery Signal Classification Using Deep Convolutional Neural Networks. Computational and Mathematical Methods in Medicine No. 2020 (2020), pp.1-10.
https://search.emarefa.net/detail/BIM-1139345
American Medical Association (AMA)
Zhang, Kai& Xu, Guanghua& Chen, Longting& Tian, Peiyuan& Han, ChengCheng& Zhang, Sicong…[et al.]. Instance Transfer Subject-Dependent Strategy for Motor Imagery Signal Classification Using Deep Convolutional Neural Networks. Computational and Mathematical Methods in Medicine. 2020. Vol. 2020, no. 2020, pp.1-10.
https://search.emarefa.net/detail/BIM-1139345
Data Type
Journal Articles
Language
English
Notes
Includes bibliographical references
Record ID
BIM-1139345