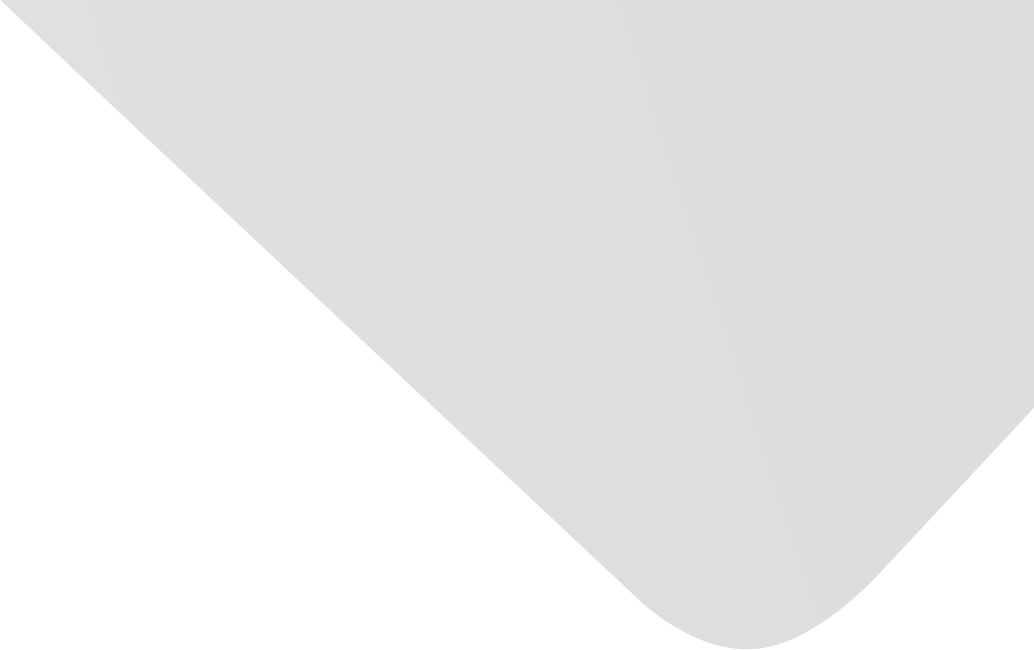
Group Guided Fused Laplacian Sparse Group Lasso for Modeling Alzheimer’s Disease Progression
Joint Authors
Wang, Jianzhong
Kong, Jun
Liu, Xiaoli
Ren, Fulong
Source
Computational and Mathematical Methods in Medicine
Issue
Vol. 2020, Issue 2020 (31 Dec. 2020), pp.1-23, 23 p.
Publisher
Hindawi Publishing Corporation
Publication Date
2020-02-07
Country of Publication
Egypt
No. of Pages
23
Main Subjects
Abstract EN
As the largest cause of dementia, Alzheimer’s disease (AD) has brought serious burdens to patients and their families, mostly in the financial, psychological, and emotional aspects.
In order to assess the progression of AD and develop new treatment methods for the disease, it is essential to infer the trajectories of patients’ cognitive performance over time to identify biomarkers that connect the patterns of brain atrophy and AD progression.
In this article, a structured regularized regression approach termed group guided fused Laplacian sparse group Lasso (GFL-SGL) is proposed to infer disease progression by considering multiple prediction of the same cognitive scores at different time points (longitudinal analysis).
The proposed GFL-SGL simultaneously exploits the interrelated structures within the MRI features and among the tasks with sparse group Lasso (SGL) norm and presents a novel group guided fused Laplacian (GFL) regularization.
This combination effectively incorporates both the relatedness among multiple longitudinal time points with a general weighted (undirected) dependency graphs and useful inherent group structure in features.
Furthermore, an alternating direction method of multipliers- (ADMM-) based algorithm is also derived to optimize the nonsmooth objective function of the proposed approach.
Experiments on the dataset from Alzheimer’s Disease Neuroimaging Initiative (ADNI) show that the proposed GFL-SGL outperformed some other state-of-the-art algorithms and effectively fused the multimodality data.
The compact sets of cognition-relevant imaging biomarkers identified by our approach are consistent with the results of clinical studies.
American Psychological Association (APA)
Liu, Xiaoli& Wang, Jianzhong& Ren, Fulong& Kong, Jun. 2020. Group Guided Fused Laplacian Sparse Group Lasso for Modeling Alzheimer’s Disease Progression. Computational and Mathematical Methods in Medicine،Vol. 2020, no. 2020, pp.1-23.
https://search.emarefa.net/detail/BIM-1139422
Modern Language Association (MLA)
Liu, Xiaoli…[et al.]. Group Guided Fused Laplacian Sparse Group Lasso for Modeling Alzheimer’s Disease Progression. Computational and Mathematical Methods in Medicine No. 2020 (2020), pp.1-23.
https://search.emarefa.net/detail/BIM-1139422
American Medical Association (AMA)
Liu, Xiaoli& Wang, Jianzhong& Ren, Fulong& Kong, Jun. Group Guided Fused Laplacian Sparse Group Lasso for Modeling Alzheimer’s Disease Progression. Computational and Mathematical Methods in Medicine. 2020. Vol. 2020, no. 2020, pp.1-23.
https://search.emarefa.net/detail/BIM-1139422
Data Type
Journal Articles
Language
English
Notes
Includes bibliographical references
Record ID
BIM-1139422