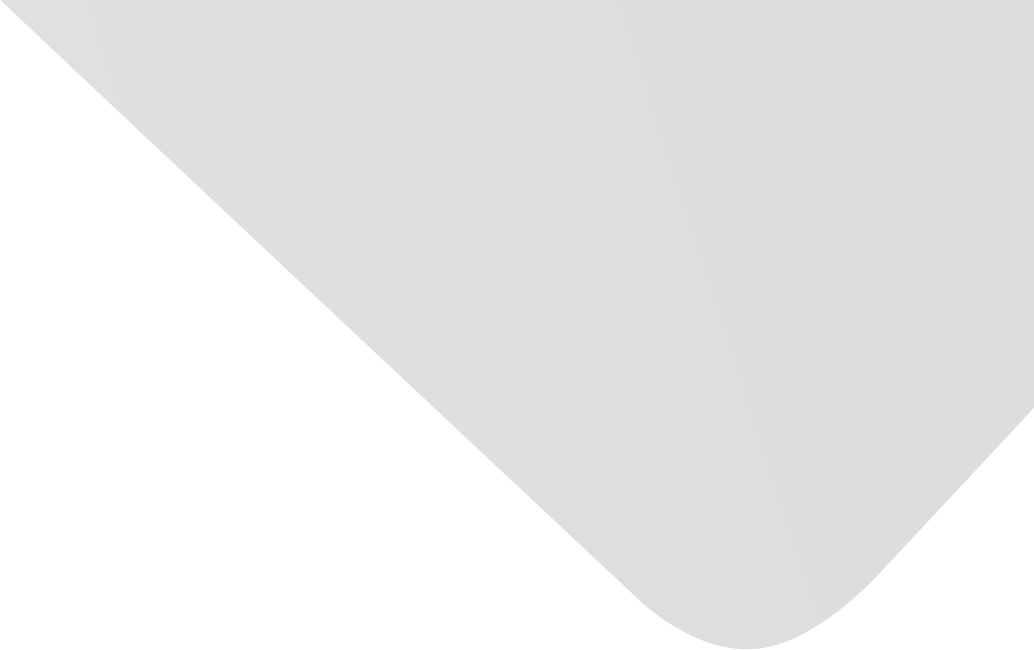
A Comparative Analysis of Visual Encoding Models Based on Classification and Segmentation Task-Driven CNNs
Joint Authors
Tong, Li
Zhang, Chi
Yu, Ziya
Wang, Linyuan
Yan, Bin
Source
Computational and Mathematical Methods in Medicine
Issue
Vol. 2020, Issue 2020 (31 Dec. 2020), pp.1-15, 15 p.
Publisher
Hindawi Publishing Corporation
Publication Date
2020-08-01
Country of Publication
Egypt
No. of Pages
15
Main Subjects
Abstract EN
Nowadays, visual encoding models use convolution neural networks (CNNs) with outstanding performance in computer vision to simulate the process of human information processing.
However, the prediction performances of encoding models will have differences based on different networks driven by different tasks.
Here, the impact of network tasks on encoding models is studied.
Using functional magnetic resonance imaging (fMRI) data, the features of natural visual stimulation are extracted using a segmentation network (FCN32s) and a classification network (VGG16) with different visual tasks but similar network structure.
Then, using three sets of features, i.e., segmentation, classification, and fused features, the regularized orthogonal matching pursuit (ROMP) method is used to establish the linear mapping from features to voxel responses.
The analysis results indicate that encoding models based on networks performing different tasks can effectively but differently predict stimulus-induced responses measured by fMRI.
The prediction accuracy of the encoding model based on VGG is found to be significantly better than that of the model based on FCN in most voxels but similar to that of fused features.
The comparative analysis demonstrates that the CNN performing the classification task is more similar to human visual processing than that performing the segmentation task.
American Psychological Association (APA)
Yu, Ziya& Zhang, Chi& Wang, Linyuan& Tong, Li& Yan, Bin. 2020. A Comparative Analysis of Visual Encoding Models Based on Classification and Segmentation Task-Driven CNNs. Computational and Mathematical Methods in Medicine،Vol. 2020, no. 2020, pp.1-15.
https://search.emarefa.net/detail/BIM-1139475
Modern Language Association (MLA)
Yu, Ziya…[et al.]. A Comparative Analysis of Visual Encoding Models Based on Classification and Segmentation Task-Driven CNNs. Computational and Mathematical Methods in Medicine No. 2020 (2020), pp.1-15.
https://search.emarefa.net/detail/BIM-1139475
American Medical Association (AMA)
Yu, Ziya& Zhang, Chi& Wang, Linyuan& Tong, Li& Yan, Bin. A Comparative Analysis of Visual Encoding Models Based on Classification and Segmentation Task-Driven CNNs. Computational and Mathematical Methods in Medicine. 2020. Vol. 2020, no. 2020, pp.1-15.
https://search.emarefa.net/detail/BIM-1139475
Data Type
Journal Articles
Language
English
Notes
Includes bibliographical references
Record ID
BIM-1139475