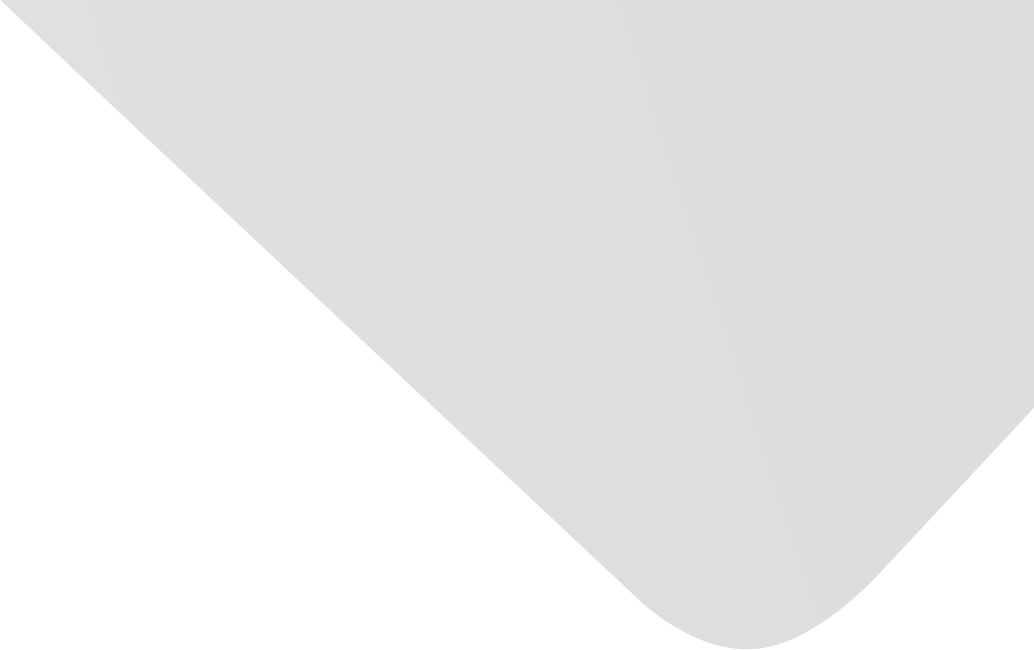
A Novel Ensemble Method for Imbalanced Data Learning: Bagging of Extrapolation-SMOTE SVM
Joint Authors
Feng, Yanghe
Wang, Qi
Luo, ZhiHao
Huang, JinCai
Liu, Zhong
Source
Computational Intelligence and Neuroscience
Issue
Vol. 2017, Issue 2017 (31 Dec. 2017), pp.1-11, 11 p.
Publisher
Hindawi Publishing Corporation
Publication Date
2017-01-30
Country of Publication
Egypt
No. of Pages
11
Main Subjects
Abstract EN
Class imbalance ubiquitously exists in real life, which has attracted much interest from various domains.
Direct learning from imbalanced dataset may pose unsatisfying results overfocusing on the accuracy of identification and deriving a suboptimal model.
Various methodologies have been developed in tackling this problem including sampling, cost-sensitive, and other hybrid ones.
However, the samples near the decision boundary which contain more discriminative information should be valued and the skew of the boundary would be corrected by constructing synthetic samples.
Inspired by the truth and sense of geometry, we designed a new synthetic minority oversampling technique to incorporate the borderline information.
What is more, ensemble model always tends to capture more complicated and robust decision boundary in practice.
Taking these factors into considerations, a novel ensemble method, called Bagging of Extrapolation Borderline-SMOTE SVM (BEBS), has been proposed in dealing with imbalanced data learning (IDL) problems.
Experiments on open access datasets showed significant superior performance using our model and a persuasive and intuitive explanation behind the method was illustrated.
As far as we know, this is the first model combining ensemble of SVMs with borderline information for solving such condition.
American Psychological Association (APA)
Wang, Qi& Luo, ZhiHao& Huang, JinCai& Feng, Yanghe& Liu, Zhong. 2017. A Novel Ensemble Method for Imbalanced Data Learning: Bagging of Extrapolation-SMOTE SVM. Computational Intelligence and Neuroscience،Vol. 2017, no. 2017, pp.1-11.
https://search.emarefa.net/detail/BIM-1139843
Modern Language Association (MLA)
Wang, Qi…[et al.]. A Novel Ensemble Method for Imbalanced Data Learning: Bagging of Extrapolation-SMOTE SVM. Computational Intelligence and Neuroscience No. 2017 (2017), pp.1-11.
https://search.emarefa.net/detail/BIM-1139843
American Medical Association (AMA)
Wang, Qi& Luo, ZhiHao& Huang, JinCai& Feng, Yanghe& Liu, Zhong. A Novel Ensemble Method for Imbalanced Data Learning: Bagging of Extrapolation-SMOTE SVM. Computational Intelligence and Neuroscience. 2017. Vol. 2017, no. 2017, pp.1-11.
https://search.emarefa.net/detail/BIM-1139843
Data Type
Journal Articles
Language
English
Notes
Includes bibliographical references
Record ID
BIM-1139843