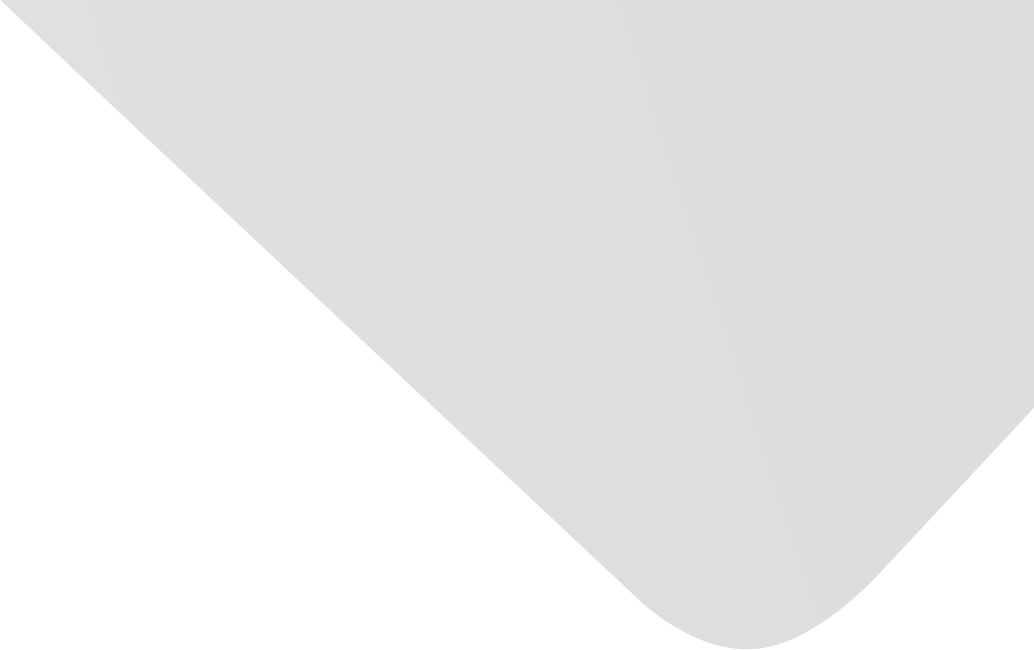
Identification of Anisomerous Motor Imagery EEG Signals Based on Complex Algorithms
Joint Authors
Duan, Feng
Liu, Rensong
Zhang, Zhiwen
Zhou, Xin
Meng, Zixuan
Source
Computational Intelligence and Neuroscience
Issue
Vol. 2017, Issue 2017 (31 Dec. 2017), pp.1-12, 12 p.
Publisher
Hindawi Publishing Corporation
Publication Date
2017-08-09
Country of Publication
Egypt
No. of Pages
12
Main Subjects
Abstract EN
Motor imagery (MI) electroencephalograph (EEG) signals are widely applied in brain-computer interface (BCI).
However, classified MI states are limited, and their classification accuracy rates are low because of the characteristics of nonlinearity and nonstationarity.
This study proposes a novel MI pattern recognition system that is based on complex algorithms for classifying MI EEG signals.
In electrooculogram (EOG) artifact preprocessing, band-pass filtering is performed to obtain the frequency band of MI-related signals, and then, canonical correlation analysis (CCA) combined with wavelet threshold denoising (WTD) is used for EOG artifact preprocessing.
We propose a regularized common spatial pattern (R-CSP) algorithm for EEG feature extraction by incorporating the principle of generic learning.
A new classifier combining the K-nearest neighbor (KNN) and support vector machine (SVM) approaches is used to classify four anisomerous states, namely, imaginary movements with the left hand, right foot, and right shoulder and the resting state.
The highest classification accuracy rate is 92.5%, and the average classification accuracy rate is 87%.
The proposed complex algorithm identification method can significantly improve the identification rate of the minority samples and the overall classification performance.
American Psychological Association (APA)
Liu, Rensong& Zhang, Zhiwen& Duan, Feng& Zhou, Xin& Meng, Zixuan. 2017. Identification of Anisomerous Motor Imagery EEG Signals Based on Complex Algorithms. Computational Intelligence and Neuroscience،Vol. 2017, no. 2017, pp.1-12.
https://search.emarefa.net/detail/BIM-1139856
Modern Language Association (MLA)
Liu, Rensong…[et al.]. Identification of Anisomerous Motor Imagery EEG Signals Based on Complex Algorithms. Computational Intelligence and Neuroscience No. 2017 (2017), pp.1-12.
https://search.emarefa.net/detail/BIM-1139856
American Medical Association (AMA)
Liu, Rensong& Zhang, Zhiwen& Duan, Feng& Zhou, Xin& Meng, Zixuan. Identification of Anisomerous Motor Imagery EEG Signals Based on Complex Algorithms. Computational Intelligence and Neuroscience. 2017. Vol. 2017, no. 2017, pp.1-12.
https://search.emarefa.net/detail/BIM-1139856
Data Type
Journal Articles
Language
English
Notes
Includes bibliographical references
Record ID
BIM-1139856