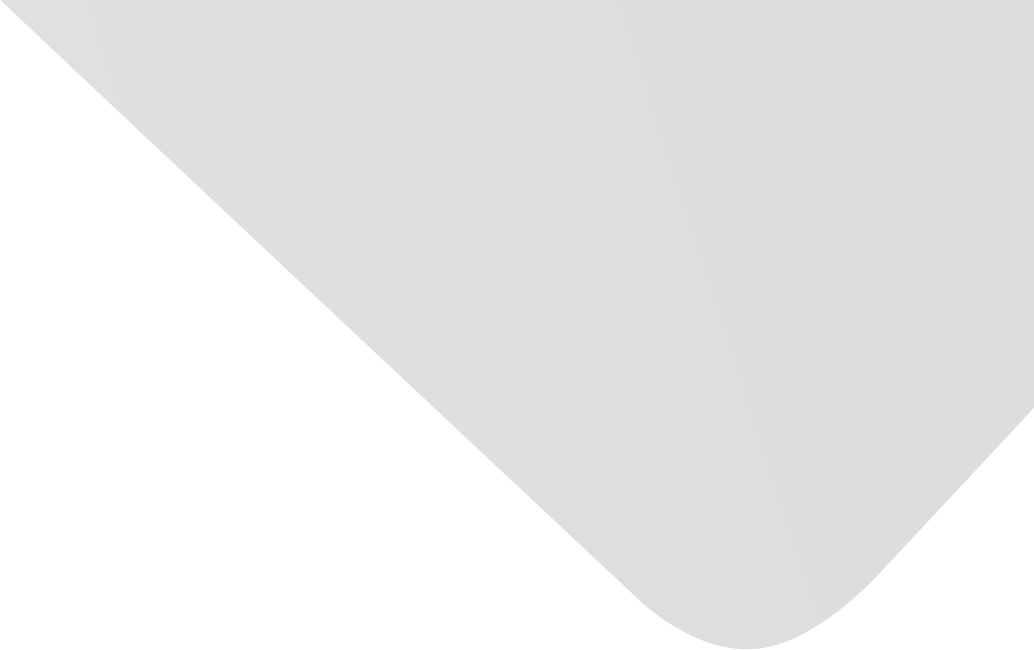
Loss Architecture Search for Few-Shot Object Recognition
Joint Authors
Yue, Jun
He, Yueguang
Du, Nianchun
Miao, Zelang
Source
Issue
Vol. 2020, Issue 2020 (31 Dec. 2020), pp.1-10, 10 p.
Publisher
Hindawi Publishing Corporation
Publication Date
2020-08-24
Country of Publication
Egypt
No. of Pages
10
Main Subjects
Abstract EN
Few-shot object recognition, which exploits a set of well-labeled data to build a classifier for new classes that have only several samples per class, has received extensive attention from the machine learning community.
In this paper, we investigate the problem of designing an optimal loss function for few-shot object recognition and propose a novel few-shot object recognition system that includes the following three steps: (1) generate a loss function architecture using a recurrent neural network (generator); (2) train a base embedding network with the generated loss function on a training set; (3) fine-tune the base embedding network using the few-shot instances from a validation set to obtain the accuracy and use it as a reward signal to update the generator.
This procedure is repeated and implemented in the reinforcement learning framework for finding the best loss architecture such that the embedding network yields the highest validation accuracy.
Our key insight is to create a search space of the loss function architectures and evaluate the quality of a particular loss function on the dataset of interest.
We conduct experiments on three popular datasets for few-shot learning.
The results show that the proposed approach achieves better performance than state-of-the-art methods.
American Psychological Association (APA)
Yue, Jun& Miao, Zelang& He, Yueguang& Du, Nianchun. 2020. Loss Architecture Search for Few-Shot Object Recognition. Complexity،Vol. 2020, no. 2020, pp.1-10.
https://search.emarefa.net/detail/BIM-1139860
Modern Language Association (MLA)
Yue, Jun…[et al.]. Loss Architecture Search for Few-Shot Object Recognition. Complexity No. 2020 (2020), pp.1-10.
https://search.emarefa.net/detail/BIM-1139860
American Medical Association (AMA)
Yue, Jun& Miao, Zelang& He, Yueguang& Du, Nianchun. Loss Architecture Search for Few-Shot Object Recognition. Complexity. 2020. Vol. 2020, no. 2020, pp.1-10.
https://search.emarefa.net/detail/BIM-1139860
Data Type
Journal Articles
Language
English
Notes
Includes bibliographical references
Record ID
BIM-1139860