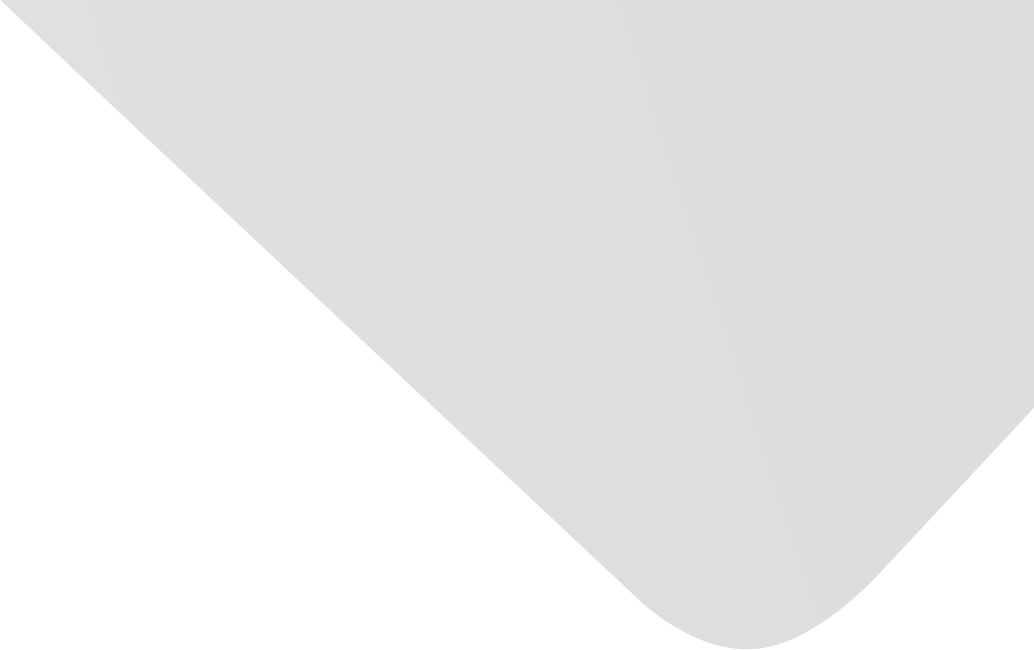
Short-Term Load Forecasting with Improved CEEMDAN and GWO-Based Multiple Kernel ELM
Joint Authors
Li, Taiyong
Qian, Zijie
He, Ting
Source
Issue
Vol. 2020, Issue 2020 (31 Dec. 2020), pp.1-20, 20 p.
Publisher
Hindawi Publishing Corporation
Publication Date
2020-02-25
Country of Publication
Egypt
No. of Pages
20
Main Subjects
Abstract EN
Short-term load forecasting (STLF) is an essential and challenging task for power- or energy-providing companies.
Recent research has demonstrated that a framework called “decomposition and ensemble” is very powerful for energy forecasting.
To improve the effectiveness of STLF, this paper proposes a novel approach integrating the improved complete ensemble empirical mode decomposition with adaptive noise (ICEEMDAN), grey wolf optimization (GWO), and multiple kernel extreme learning machine (MKELM), namely, ICEEMDAN-GWO-MKELM, for STLF, following this framework.
The proposed ICEEMDAN-GWO-MKELM consists of three stages.
First, the complex raw load data are decomposed into a couple of relatively simple components by ICEEMDAN.
Second, MKELM is used to forecast each decomposed component individually.
Specifically, we use GWO to optimize both the weight and the parameters of every single kernel in extreme learning machine to improve the forecasting ability.
Finally, the results of all the components are aggregated as the final forecasting result.
The extensive experiments reveal that the ICEEMDAN-GWO-MKELM can outperform several state-of-the-art forecasting approaches in terms of some evaluation criteria, showing that the ICEEMDAN-GWO-MKELM is very effective for STLF.
American Psychological Association (APA)
Li, Taiyong& Qian, Zijie& He, Ting. 2020. Short-Term Load Forecasting with Improved CEEMDAN and GWO-Based Multiple Kernel ELM. Complexity،Vol. 2020, no. 2020, pp.1-20.
https://search.emarefa.net/detail/BIM-1139877
Modern Language Association (MLA)
Li, Taiyong…[et al.]. Short-Term Load Forecasting with Improved CEEMDAN and GWO-Based Multiple Kernel ELM. Complexity No. 2020 (2020), pp.1-20.
https://search.emarefa.net/detail/BIM-1139877
American Medical Association (AMA)
Li, Taiyong& Qian, Zijie& He, Ting. Short-Term Load Forecasting with Improved CEEMDAN and GWO-Based Multiple Kernel ELM. Complexity. 2020. Vol. 2020, no. 2020, pp.1-20.
https://search.emarefa.net/detail/BIM-1139877
Data Type
Journal Articles
Language
English
Notes
Includes bibliographical references
Record ID
BIM-1139877