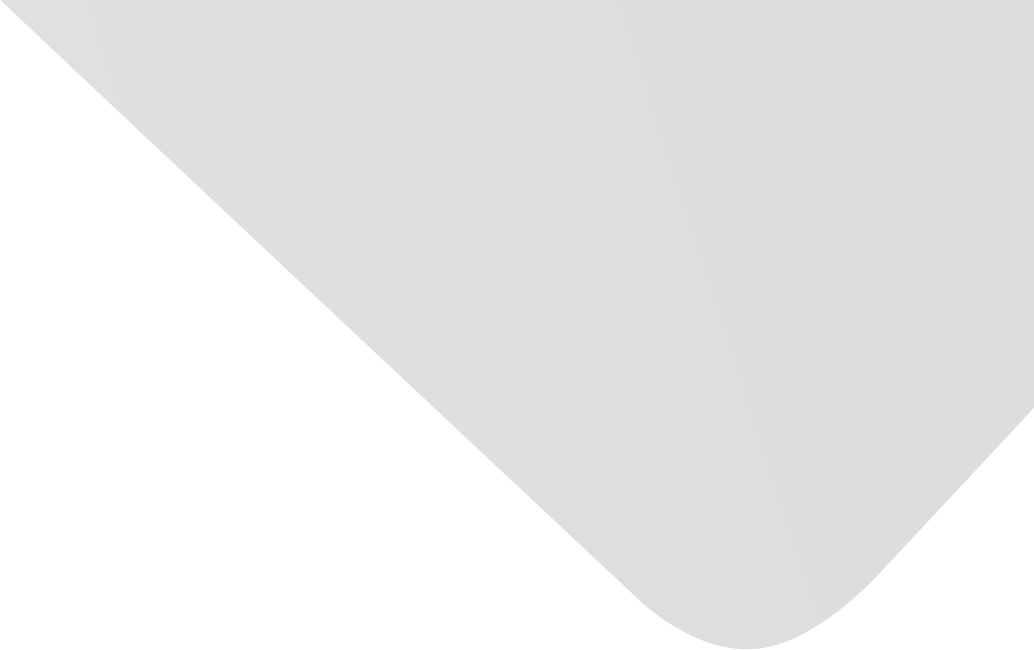
Multichannel Deep Attention Neural Networks for the Classification of Autism Spectrum Disorder Using Neuroimaging and Personal Characteristic Data
Joint Authors
Niu, Ke
Li, Hailong
Gao, Xin
Guo, Jiayang
Pan, Yijie
Peng, Xueping
Li, Ning
Source
Issue
Vol. 2020, Issue 2020 (31 Dec. 2020), pp.1-9, 9 p.
Publisher
Hindawi Publishing Corporation
Publication Date
2020-01-31
Country of Publication
Egypt
No. of Pages
9
Main Subjects
Abstract EN
Autism spectrum disorder (ASD) is a developmental disorder that impacts more than 1.6% of children aged 8 across the United States.
It is characterized by impairments in social interaction and communication, as well as by a restricted repertoire of activity and interests.
The current standardized clinical diagnosis of ASD remains to be a subjective diagnosis, mainly relying on behavior-based tests.
However, the diagnostic process for ASD is not only time consuming, but also costly, causing a tremendous financial burden for patients’ families.
Therefore, automated diagnosis approaches have been an attractive solution for earlier identification of ASD.
In this work, we set to develop a deep learning model for automated diagnosis of ASD.
Specifically, a multichannel deep attention neural network (DANN) was proposed by integrating multiple layers of neural networks, attention mechanism, and feature fusion to capture the interrelationships in multimodality data.
We evaluated the proposed multichannel DANN model on the Autism Brain Imaging Data Exchange (ABIDE) repository with 809 subjects (408 ASD patients and 401 typical development controls).
Our model achieved a state-of-the-art accuracy of 0.732 on ASD classification by integrating three scales of brain functional connectomes and personal characteristic data, outperforming multiple peer machine learning models in a k-fold cross validation experiment.
Additional k-fold and leave-one-site-out cross validation were conducted to test the generalizability and robustness of the proposed multichannel DANN model.
The results show promise for deep learning models to aid the future automated clinical diagnosis of ASD.
American Psychological Association (APA)
Niu, Ke& Guo, Jiayang& Pan, Yijie& Gao, Xin& Peng, Xueping& Li, Ning…[et al.]. 2020. Multichannel Deep Attention Neural Networks for the Classification of Autism Spectrum Disorder Using Neuroimaging and Personal Characteristic Data. Complexity،Vol. 2020, no. 2020, pp.1-9.
https://search.emarefa.net/detail/BIM-1139893
Modern Language Association (MLA)
Niu, Ke…[et al.]. Multichannel Deep Attention Neural Networks for the Classification of Autism Spectrum Disorder Using Neuroimaging and Personal Characteristic Data. Complexity No. 2020 (2020), pp.1-9.
https://search.emarefa.net/detail/BIM-1139893
American Medical Association (AMA)
Niu, Ke& Guo, Jiayang& Pan, Yijie& Gao, Xin& Peng, Xueping& Li, Ning…[et al.]. Multichannel Deep Attention Neural Networks for the Classification of Autism Spectrum Disorder Using Neuroimaging and Personal Characteristic Data. Complexity. 2020. Vol. 2020, no. 2020, pp.1-9.
https://search.emarefa.net/detail/BIM-1139893
Data Type
Journal Articles
Language
English
Notes
Includes bibliographical references
Record ID
BIM-1139893