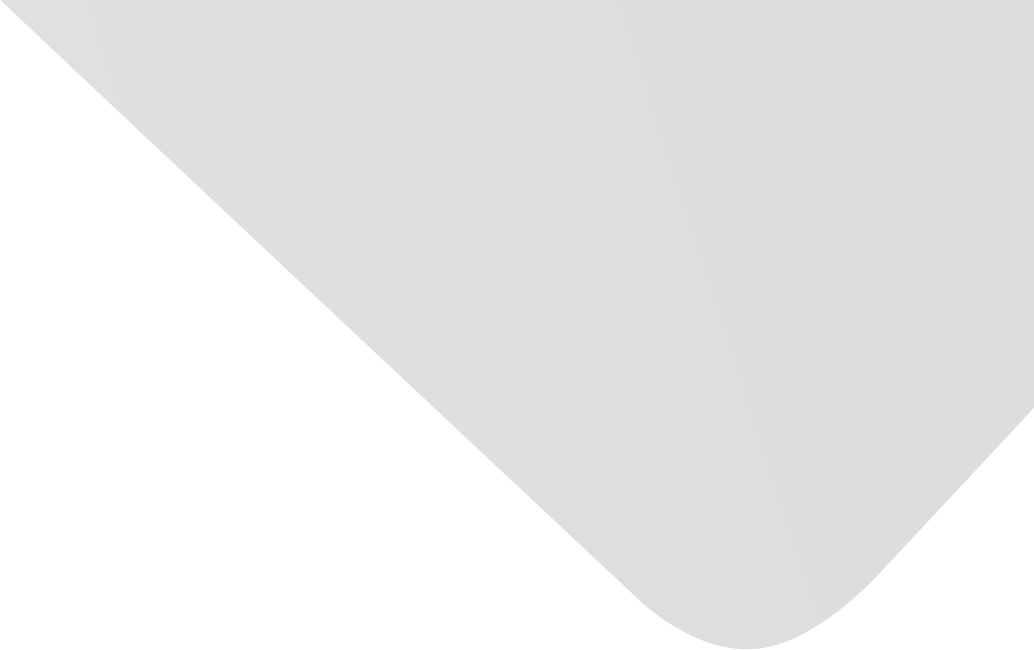
Prediction of Future Terrorist Activities Using Deep Neural Networks
Joint Authors
Aziz, Furqan
Saeed, Yousaf
Uddin, M. Irfan
Zada, Nazir
Zeb, Asim
Ali Shah, Syed Atif
Al-Khasawneh, Mahmoud Ahmad
Mahmoud, Marwan
Source
Issue
Vol. 2020, Issue 2020 (31 Dec. 2020), pp.1-16, 16 p.
Publisher
Hindawi Publishing Corporation
Publication Date
2020-04-22
Country of Publication
Egypt
No. of Pages
16
Main Subjects
Abstract EN
One of the most important threats to today’s civilization is terrorism.
Terrorism not only disturbs the law and order situations in a society but also affects the quality of lives of humans and makes them suppressed physically and emotionally and deprives them of enjoying life.
The more the civilizations have advanced, the more the people are working towards exploring different mechanisms to protect the mankind from terrorism.
Different techniques have been used as counterterrorism to protect the lives of individuals in society and to improve the quality of life in general.
Machine learning methods have been recently explored to develop techniques for counterterrorism based on artificial intelligence (AI).
Since deep learning has recently gained more popularity in machine learning domain, in this paper, these techniques are explored to understand the behavior of terrorist activities.
Five different models based on deep neural network (DNN) are created to understand the behavior of terrorist activities such as is the attack going to be successful or not? Or whether the attack is going to be suicide or not? Or what type of weapon is going to be used in the attack? Or what type of attack is going to be carried out? Or what region is going to be attacked? The models are implemented in single-layer neural network (NN), five-layer DNN, and three traditional machine learning algorithms, i.e., logistic regression, SVM, and Naïve Bayes.
The performance of the DNN is compared with NN and the three machine learning algorithms, and it is demonstrated that the performance in DNN is more than 95% in terms of accuracy, precision, recall, and F1-Score, while ANN and traditional machine learning algorithms have achieved a maximum of 83% accuracy.
This concludes that DNN is a suitable model to be used for predicting the behavior of terrorist activities.
Our experiments also demonstrate that the dataset for terrorist activities is big data; therefore, a DNN is a suitable model to process big data and understand the underlying patterns in the dataset.
American Psychological Association (APA)
Uddin, M. Irfan& Zada, Nazir& Aziz, Furqan& Saeed, Yousaf& Zeb, Asim& Ali Shah, Syed Atif…[et al.]. 2020. Prediction of Future Terrorist Activities Using Deep Neural Networks. Complexity،Vol. 2020, no. 2020, pp.1-16.
https://search.emarefa.net/detail/BIM-1139894
Modern Language Association (MLA)
Uddin, M. Irfan…[et al.]. Prediction of Future Terrorist Activities Using Deep Neural Networks. Complexity No. 2020 (2020), pp.1-16.
https://search.emarefa.net/detail/BIM-1139894
American Medical Association (AMA)
Uddin, M. Irfan& Zada, Nazir& Aziz, Furqan& Saeed, Yousaf& Zeb, Asim& Ali Shah, Syed Atif…[et al.]. Prediction of Future Terrorist Activities Using Deep Neural Networks. Complexity. 2020. Vol. 2020, no. 2020, pp.1-16.
https://search.emarefa.net/detail/BIM-1139894
Data Type
Journal Articles
Language
English
Notes
Includes bibliographical references
Record ID
BIM-1139894