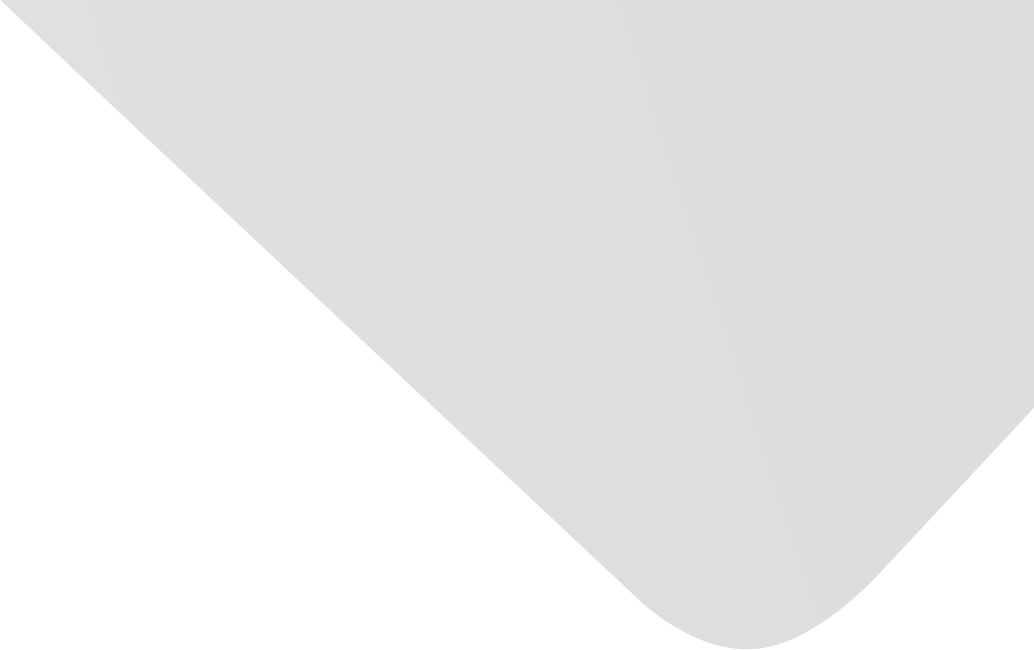
A Multiscale and High-Precision LSTM-GASVR Short-Term Traffic Flow Prediction Model
Joint Authors
Zhou, Jingmei
Chang, Hui
Cheng, Xin
Zhao, Xiangmo
Source
Issue
Vol. 2020, Issue 2020 (31 Dec. 2020), pp.1-17, 17 p.
Publisher
Hindawi Publishing Corporation
Publication Date
2020-06-17
Country of Publication
Egypt
No. of Pages
17
Main Subjects
Abstract EN
Short-term traffic flow has the characteristics of complex, changeable, strong timeliness, and so on.
So the traditional prediction algorithm is difficult to meet its high real-time and accuracy requirements.
In this paper, a multiscale and high-precision LSTM-GASVR short-term traffic flow prediction algorithm is proposed.
This method uses 15 min traffic flow data of the first 16 sections as input and completes the data preprocessing operation through reconstruction, normalization, and rising dimension by working day factor; establishing the prediction model based on the long- and short-term memory network (LSTM) and inverse normalization; and proposing the GA-SVR model to optimize the prediction results, so as to realize the real-time high-precision prediction of traffic flow.
The prediction experiment is carried out according to the charge data of a toll station in Xi’an, Shaanxi Province, from May 2018 to May 2019.
The comparison and analysis of various algorithms show that the prediction algorithm proposed in this paper is 20% higher than the LSTM, GRU, CNN, SAE, ARIMA, and SVR, and the R2 can reach 0.982, the explanatory variance is 0.982, and the MAPE is 0.118.
The proposed traffic flow prediction algorithm provides strong support for traffic managers to judge the state of the road network to control traffic and guide traffic flow.
American Psychological Association (APA)
Zhou, Jingmei& Chang, Hui& Cheng, Xin& Zhao, Xiangmo. 2020. A Multiscale and High-Precision LSTM-GASVR Short-Term Traffic Flow Prediction Model. Complexity،Vol. 2020, no. 2020, pp.1-17.
https://search.emarefa.net/detail/BIM-1139903
Modern Language Association (MLA)
Zhou, Jingmei…[et al.]. A Multiscale and High-Precision LSTM-GASVR Short-Term Traffic Flow Prediction Model. Complexity No. 2020 (2020), pp.1-17.
https://search.emarefa.net/detail/BIM-1139903
American Medical Association (AMA)
Zhou, Jingmei& Chang, Hui& Cheng, Xin& Zhao, Xiangmo. A Multiscale and High-Precision LSTM-GASVR Short-Term Traffic Flow Prediction Model. Complexity. 2020. Vol. 2020, no. 2020, pp.1-17.
https://search.emarefa.net/detail/BIM-1139903
Data Type
Journal Articles
Language
English
Notes
Includes bibliographical references
Record ID
BIM-1139903