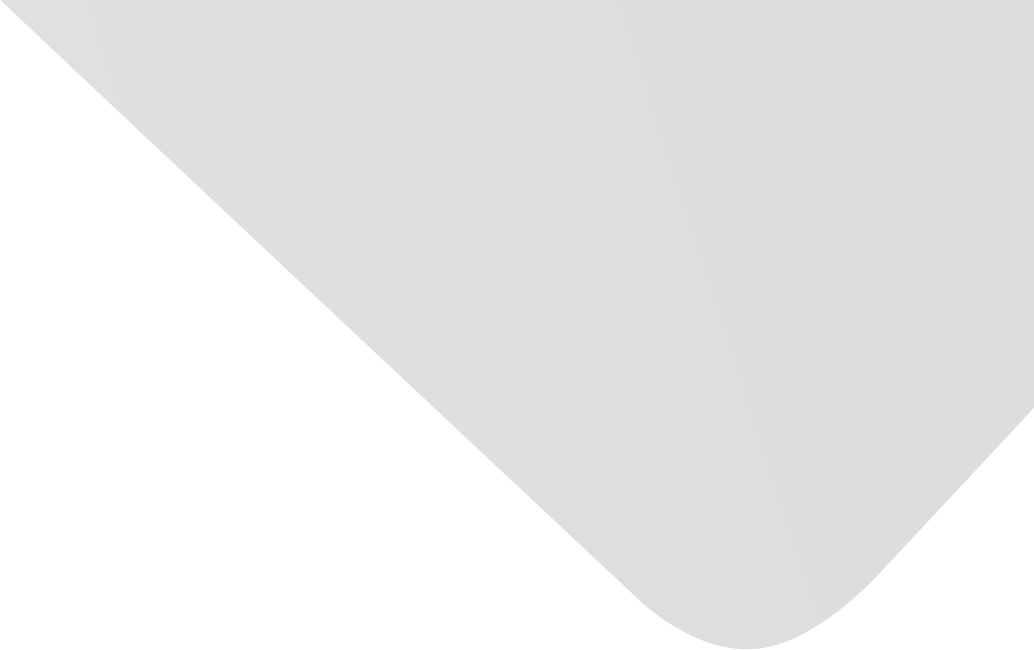
Medical Image Segmentation Algorithm Based on Optimized Convolutional Neural Network-Adaptive Dropout Depth Calculation
Joint Authors
Source
Issue
Vol. 2020, Issue 2020 (31 Dec. 2020), pp.1-13, 13 p.
Publisher
Hindawi Publishing Corporation
Publication Date
2020-05-15
Country of Publication
Egypt
No. of Pages
13
Main Subjects
Abstract EN
Medical image segmentation is a key technology for image guidance.
Therefore, the advantages and disadvantages of image segmentation play an important role in image-guided surgery.
Traditional machine learning methods have achieved certain beneficial effects in medical image segmentation, but they have problems such as low classification accuracy and poor robustness.
Deep learning theory has good generalizability and feature extraction ability, which provides a new idea for solving medical image segmentation problems.
However, deep learning has problems in terms of its application to medical image segmentation: one is that the deep learning network structure cannot be constructed according to medical image characteristics; the other is that the generalizability y of the deep learning model is weak.
To address these issues, this paper first adapts a neural network to medical image features by adding cross-layer connections to a traditional convolutional neural network.
In addition, an optimized convolutional neural network model is established.
The optimized convolutional neural network model can segment medical images using the features of two scales simultaneously.
At the same time, to solve the generalizability problem of the deep learning model, an adaptive distribution function is designed according to the position of the hidden layer, and then the activation probability of each layer of neurons is set.
This enhances the generalizability of the dropout model, and an adaptive dropout model is proposed.
This model better addresses the problem of the weak generalizability of deep learning models.
Based on the above ideas, this paper proposes a medical image segmentation algorithm based on an optimized convolutional neural network with adaptive dropout depth calculation.
An ultrasonic tomographic image and lumbar CT medical image were separately segmented by the method of this paper.
The experimental results show that not only are the segmentation effects of the proposed method improved compared with those of the traditional machine learning and other deep learning methods but also the method has a high adaptive segmentation ability for various medical images.
The research work in this paper provides a new perspective for research on medical image segmentation.
American Psychological Association (APA)
An, Feng-Ping& Liu, Jun-e. 2020. Medical Image Segmentation Algorithm Based on Optimized Convolutional Neural Network-Adaptive Dropout Depth Calculation. Complexity،Vol. 2020, no. 2020, pp.1-13.
https://search.emarefa.net/detail/BIM-1139931
Modern Language Association (MLA)
An, Feng-Ping& Liu, Jun-e. Medical Image Segmentation Algorithm Based on Optimized Convolutional Neural Network-Adaptive Dropout Depth Calculation. Complexity No. 2020 (2020), pp.1-13.
https://search.emarefa.net/detail/BIM-1139931
American Medical Association (AMA)
An, Feng-Ping& Liu, Jun-e. Medical Image Segmentation Algorithm Based on Optimized Convolutional Neural Network-Adaptive Dropout Depth Calculation. Complexity. 2020. Vol. 2020, no. 2020, pp.1-13.
https://search.emarefa.net/detail/BIM-1139931
Data Type
Journal Articles
Language
English
Notes
Includes bibliographical references
Record ID
BIM-1139931