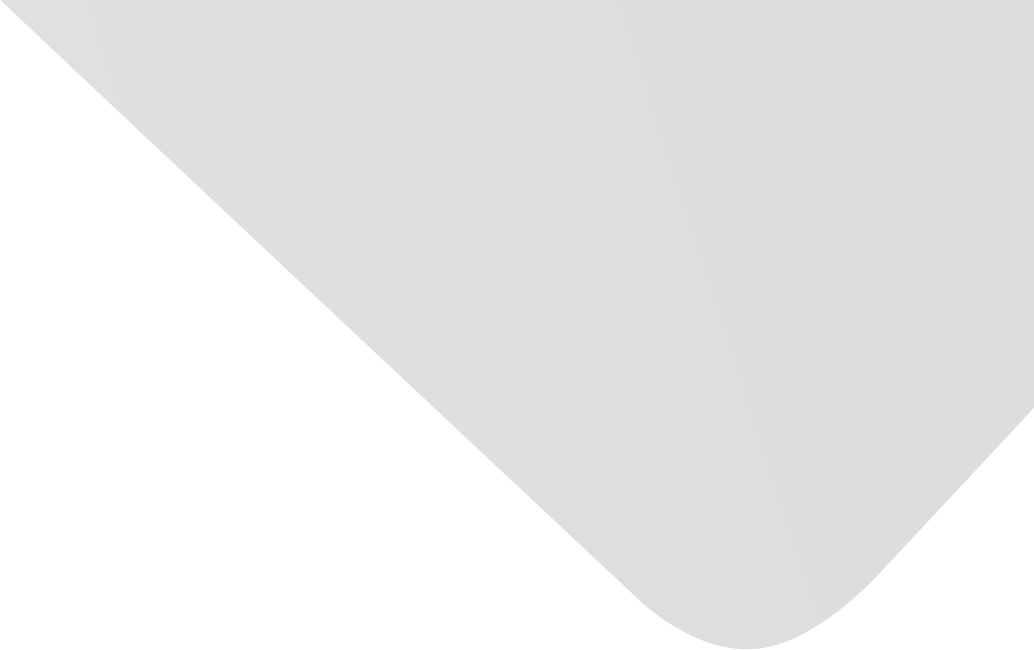
Labelling Training Samples Using Crowdsourcing Annotation for Recommendation
Joint Authors
Wang, Qingren
Zhang, Min
Tao, Tao
Sheng, Victor S.
Source
Issue
Vol. 2020, Issue 2020 (31 Dec. 2020), pp.1-10, 10 p.
Publisher
Hindawi Publishing Corporation
Publication Date
2020-05-05
Country of Publication
Egypt
No. of Pages
10
Main Subjects
Abstract EN
The supervised learning-based recommendation models, whose infrastructures are sufficient training samples with high quality, have been widely applied in many domains.
In the era of big data with the explosive growth of data volume, training samples should be labelled timely and accurately to guarantee the excellent recommendation performance of supervised learning-based models.
Machine annotation cannot complete the tasks of labelling training samples with high quality because of limited machine intelligence.
Although expert annotation can achieve a high accuracy, it requires a long time as well as more resources.
As a new way of human intelligence to participate in machine computing, crowdsourcing annotation makes up for shortages of machine annotation and expert annotation.
Therefore, in this paper, we utilize crowdsourcing annotation to label training samples.
First, a suitable crowdsourcing mechanism is designed to create crowdsourcing annotation-based tasks for training sample labelling, and then two entropy-based ground truth inference algorithms (i.e., HILED and HILI) are proposed to achieve quality improvement of noise labels provided by the crowd.
In addition, the descending and random order manners in crowdsourcing annotation-based tasks are also explored.
The experimental results demonstrate that crowdsourcing annotation significantly improves the performance of machine annotation.
Among the ground truth inference algorithms, both HILED and HILI improve the performance of baselines; meanwhile, HILED performs better than HILI.
American Psychological Association (APA)
Wang, Qingren& Zhang, Min& Tao, Tao& Sheng, Victor S.. 2020. Labelling Training Samples Using Crowdsourcing Annotation for Recommendation. Complexity،Vol. 2020, no. 2020, pp.1-10.
https://search.emarefa.net/detail/BIM-1139938
Modern Language Association (MLA)
Wang, Qingren…[et al.]. Labelling Training Samples Using Crowdsourcing Annotation for Recommendation. Complexity No. 2020 (2020), pp.1-10.
https://search.emarefa.net/detail/BIM-1139938
American Medical Association (AMA)
Wang, Qingren& Zhang, Min& Tao, Tao& Sheng, Victor S.. Labelling Training Samples Using Crowdsourcing Annotation for Recommendation. Complexity. 2020. Vol. 2020, no. 2020, pp.1-10.
https://search.emarefa.net/detail/BIM-1139938
Data Type
Journal Articles
Language
English
Notes
Includes bibliographical references
Record ID
BIM-1139938