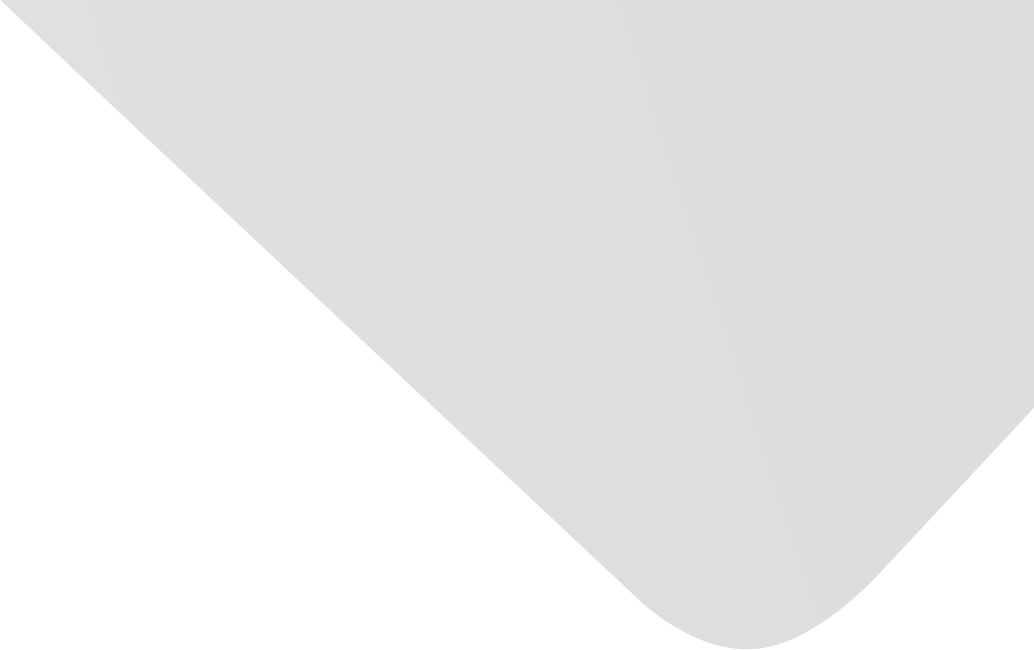
Long-Term Exchange Rate Probability Density Forecasting Using Gaussian Kernel and Quantile Random Forest
Joint Authors
Gyamerah, Samuel Asante
Moyo, Edwin
Source
Issue
Vol. 2020, Issue 2020 (31 Dec. 2020), pp.1-11, 11 p.
Publisher
Hindawi Publishing Corporation
Publication Date
2020-02-28
Country of Publication
Egypt
No. of Pages
11
Main Subjects
Abstract EN
In the midst of macro-economic uncertainties, accurate long-term exchange rate forecasting is crucial for decision-making and planning.
To measure the uncertainty associated with exchange rate and obtaining additional information of future exchange rate, a hybrid model based on quantile regression forest and Gaussian kernel (GQRF) is constructed.
Quarterly dataset of KSh/USD exchange rate and macro-economic variables from 2007 to 2016 are used.
The forecast horizon spans from 2013 to 2016.
With a prediction interval coverage probability and prediction interval average width of 95% and 29.6493%, the constructed model has a very high coverage probability.
The method of determining the probabilistic forecasts is very significant to achieve forecasts with correct coverage.
The probability density forecasting model for the exchange rate gave significant information–the probability distribution of the forecasted results.
In this way, uncertainties around the forecast can be evaluated because the complete exchange rate distribution are forecasted.
GQRF is efficient as it can uphold the uncertainty about the variance linked to each point, which is important for exchange rate forecasting.
Using the constructed model, the probabilities of exceedance such as the probability of future exchange rate exceeding the average exchange rate for the year can be computed.
This paper also adds to the scarce literature of exchange rate probability density forecasting using machine learning techniques.
American Psychological Association (APA)
Gyamerah, Samuel Asante& Moyo, Edwin. 2020. Long-Term Exchange Rate Probability Density Forecasting Using Gaussian Kernel and Quantile Random Forest. Complexity،Vol. 2020, no. 2020, pp.1-11.
https://search.emarefa.net/detail/BIM-1139985
Modern Language Association (MLA)
Gyamerah, Samuel Asante& Moyo, Edwin. Long-Term Exchange Rate Probability Density Forecasting Using Gaussian Kernel and Quantile Random Forest. Complexity No. 2020 (2020), pp.1-11.
https://search.emarefa.net/detail/BIM-1139985
American Medical Association (AMA)
Gyamerah, Samuel Asante& Moyo, Edwin. Long-Term Exchange Rate Probability Density Forecasting Using Gaussian Kernel and Quantile Random Forest. Complexity. 2020. Vol. 2020, no. 2020, pp.1-11.
https://search.emarefa.net/detail/BIM-1139985
Data Type
Journal Articles
Language
English
Notes
Includes bibliographical references
Record ID
BIM-1139985