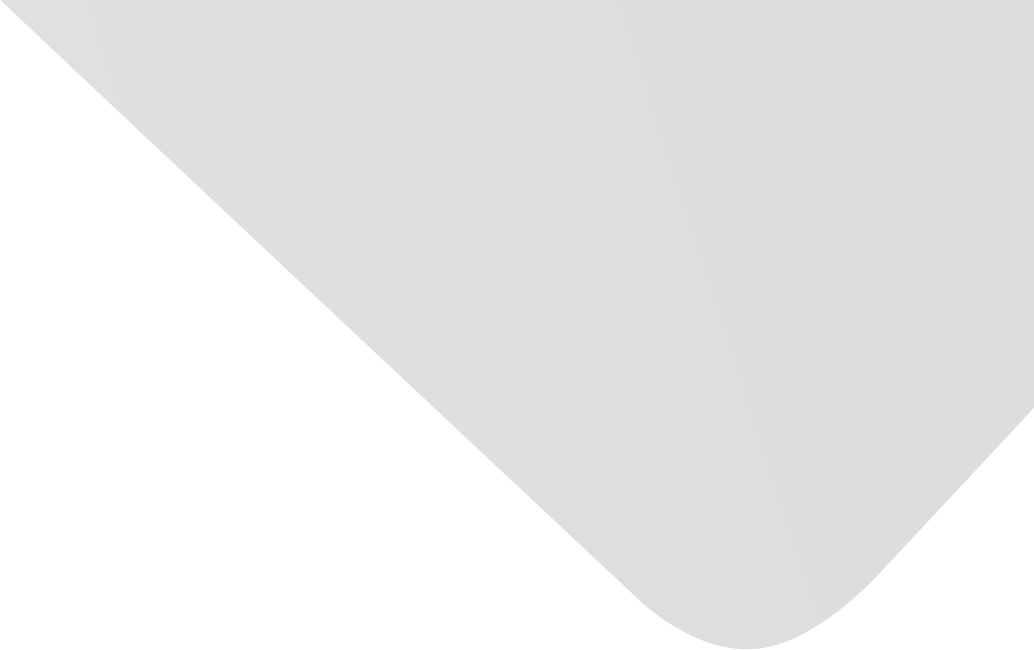
Analysis of Land Surface Temperature Driving Factors and Spatial Heterogeneity Research Based on Geographically Weighted Regression Model
Joint Authors
Zhi, Yin
Shan, Liang
Ke, Lina
Yang, Ruxin
Source
Issue
Vol. 2020, Issue 2020 (31 Dec. 2020), pp.1-9, 9 p.
Publisher
Hindawi Publishing Corporation
Publication Date
2020-10-06
Country of Publication
Egypt
No. of Pages
9
Main Subjects
Abstract EN
Acceleration of urbanization has brought about a series of problems, which include irreversible changes to urban surfaces and continuous increases in land surface temperatures (LSTs).
In this context, analysis of the driving factors and spatial heterogeneity of urban LST is of considerable importance for mitigating urban heat island effects and promoting healthy and comfortable urban living environments.
This study explored the relationship between the spatial characteristics and driving factors of the LST by using a geographically weighted regression (GWR) model to analyze multisource data from the Xigang District of Dalian City.
The results showed that the urban heat island effect in Xigang District is significant, with LSTs generally above 28°C at the end of August, mostly concentrated in the range of 38–40°C.
The highest LST values were detected in northern port and harbor areas; the lowest LST values occurred in mountainous forest areas.
The global Moran’s I value was 0.994, which was indicative of a very high positive correlation, and local Moran’s I values formed H-H and L-L type clusters concentrated in the northern harbor area and southern mountainous area, respectively.
Finally, the GWR model could reflect the spatial heterogeneity of the relationships between LST and its driving factors well.
Among these, in terms of natural physical factors, digital elevation model, normalized difference vegetation index, and modified normalized difference water index data were found to be negatively correlated with LSTs in most cases; in the social dimension, the point-of-interest number and building-coverage ratio were generally positively correlated with LSTs.
American Psychological Association (APA)
Zhi, Yin& Shan, Liang& Ke, Lina& Yang, Ruxin. 2020. Analysis of Land Surface Temperature Driving Factors and Spatial Heterogeneity Research Based on Geographically Weighted Regression Model. Complexity،Vol. 2020, no. 2020, pp.1-9.
https://search.emarefa.net/detail/BIM-1140029
Modern Language Association (MLA)
Zhi, Yin…[et al.]. Analysis of Land Surface Temperature Driving Factors and Spatial Heterogeneity Research Based on Geographically Weighted Regression Model. Complexity No. 2020 (2020), pp.1-9.
https://search.emarefa.net/detail/BIM-1140029
American Medical Association (AMA)
Zhi, Yin& Shan, Liang& Ke, Lina& Yang, Ruxin. Analysis of Land Surface Temperature Driving Factors and Spatial Heterogeneity Research Based on Geographically Weighted Regression Model. Complexity. 2020. Vol. 2020, no. 2020, pp.1-9.
https://search.emarefa.net/detail/BIM-1140029
Data Type
Journal Articles
Language
English
Notes
Includes bibliographical references
Record ID
BIM-1140029